Establishment, contagiousness, and initial spread of SARS-CoV-2 in Canada
Abstract
This study empirically quantifies dynamics of SARS-CoV-2 establishment and early spread in Canada. We developed a transmission model that was simulation tested and fitted in a Bayesian framework to timeseries of new cases per day prior to physical distancing interventions. A hierarchical version was fitted to all provinces simultaneously to obtain average estimates for Canada. Across scenarios of a latent period of 2–4 d and an infectious period of 5–9 d, the R0 estimate for Canada ranges from a minimum of 3.0 (95% CI: 2.3–3.9) to a maximum of 5.3 (95% CI: 3.9–7.1). Among provinces, the estimated commencement of community transmission ranged from 3 d before to 50 d after the first reported case and from 2 to 25 d before the first reports of community transmission. Among parameter scenarios and provinces, the median reduction in transmission needed to obtain R0 < 1 ranged from 46% (95% CI: 43%–48%) to 89% (95% CI: 88%–90%). Our results indicate that local epidemics of SARS-CoV-2 in Canada entail high levels of stochasticity, contagiousness, and observation delay, which facilitates rapid undetected spread and requires comprehensive testing and contact tracing for its containment.
1. Introduction
The pandemic of COVID-19, caused by the Severe Acute Respiratory Syndrome Coronavirus 2 (SARS-CoV-2), has spread quickly, straining health care systems and causing societal and economic upheaval (Gatto et al. 2020; Moghadas et al. 2020; Nicola et al. 2020; Tuite et al. 2020). After emerging and quickly spreading in Wuhan City in Hubei Province in China in late 2019, cases began appearing globally through international travellers (Wilson and Chen 2020; World Health Organization 2020). Transmission of the virus from asymptomatic and presymptomatic carriers has likely contributed to its rapid spread (Gatto et al. 2020; He et al. 2020; R. Li et al. 2020; Moghadas et al. 2020).
The global spread of local SARS-CoV-2 epidemics involves immigration of the virus to a new area via travellers followed by its establishment in the local community where it can spread. Demographic stochasticity of the local establishment process creates high uncertainty in the onset of a local epidemic (Bartlett 1964), which may then go undetected for an uncertain period of time due to a presymptomatic period and stochastic sampling error, possibly delaying public health interventions. The early local spread of SARS-CoV-2 was initially estimated in Hubei with a basic reproductive value, R0, in the range of 1.4–3.9 (Q. Li et al. 2020), indicating that one infected individual would on average go on to infect one to four other people. Recent analyses have however indicated that R0 in Wuhan may be higher, in the range of 3.8–8.9 (Sanche et al. 2020; Tang et al. 2020), and that R0 may similarly be higher in other countries (Gatto et al. 2020; Kochańczyk et al. 2020).
We developed a mathematical model of SARS-CoV-2 transmission that separates cases from travellers versus community spread as latent (unobserved) processes and fitted it to timeseries data of new cases per day in each Canadian province for the time period preceding social distancing interventions. The model accounts for the initial slow growth of cases prior to the onset of community transmission and could therefore give a more accurate estimate of the basic reproductive value than models that assume a specific start date for a local epidemic. The model estimates the rate of introduction of infection into the population via infected travellers (here referred to as the “infection immigration” rate), the commencement date of community spread, the transmission coefficient, and the basic reproductive value, R0. The results quantify the time delays to local establishment from travellers and from the onset of community transmission to its first reported cases. A hierarchical version of the model, fitted to data from all provinces simultaneously, provides a Canada-level meta-analytic estimate for R0 and quantifies the reduction in transmission rate needed to halt epidemic spread.
2. Methods
We used two models in our analysis, the first is the “estimating model” that we fitted to empirical data on the number of new cases per day and which constitutes the main set of results in this paper. The second model is a more complex “simulation model” that we used to simulate data to which the estimating model was fitted. The purpose of the simulation test was to evaluate estimability and bias of parameter estimates of the estimating model relative to unobserved processes such as asymptomatic transmission as well as temporal changes in the immigration rate of infected travelers. The simulation tests, described below, indicated that estimable parameters were unbiased but that the latent period and infectious period needed to be specified rather than estimated when fitting the estimating model to data.
2.1. Estimating model
Demographic stochasticity in the process of infection spilling over from travellers into a community can create a delay between the appearance of initial cases and the commencement of community transmission. To account for, and estimate, the potentially long delay in the onset of local epidemics, the estimating model, which is implemented deterministically within a Bayesian model-fitting framework, treats cases arising from returning travelers as a separate process from cases arising from local community spread. The model tracks the numbers of returning infectious travellers (T), and the numbers of susceptible (S), exposed (E), infectious (I), and recovered (R) people in a local population over time (t),where the function Ft describes the transmission dynamics in relation to the date of spillover of infection from travellers to local community spread at time tc via
(1)
(2)
The other parameters of the model describe the immigration rate of infectious travellers (ϕ), the duration of the infectious period (1/ω) such that 1−ω is the fraction of infected individuals that recover each day, the latent period (1/γ) such that 1−γ is the fraction of exposed individuals that become infectious each day, the transmission coefficient (β), and the population size of each province (N).
The basic reproductive value, R0, from this model without interventions is R0 = β/ω. The proportional reduction, θ, of the transmission rate that arises from physical distancing interventions or vaccination can be introduced into the model as a coefficient (1−θ) on β. This then gives the hypothetical proportion reduction in transmission rate required to prevent an outbreak as θ >1−1/R0 (Anderson and May 1991). Given that an outbreak has already occurred, a suppression strategy should reduce the effective reproductive value , where N is the population size, below one continuously (Ridenhour et al. 2014). This condition translates θe > 1−N/(R0S), which is lower than θ due to depletion of susceptibles due to immunity (from previous infections or vaccination).
2.2. Simulation testing
To evaluate parameter estimability, and the robustness of parameter estimates to unobserved asymptomatic transmission and nonconstant rates of infection immigration, we tested the model by fitting it to data that were simulated by a more complex data-generating model. The data-generating model expands eq. (1) into asymptomatic (Ia), presymptomatic (Ip), and symptomatic (Is), infectious classes, and also includes a constant mixing of returning infected travellers with the local population such thatwhere q is the fraction of exposed individuals that go on to become symptomatic and the duration of the infectious classes are 1/ωa, 1/ωp, and 1/ωs, respectively. The net reproductive value, R0, for the data-generating model was calculated using the next generation matrix approach (Allen and van den Driessche 2008), and the model was implemented in a demographic stochastic framework with all transitions occurring as Poisson (new infections) or binomial (all other transitions) processes. As such, the onset of community spread is not a specified parameter, but rather an emergent property of the stochastic process. We fitted the estimating model (eq. (1)) using the same likelihood function as for the empirical data to 200 simulations of the number of symptomatic individuals per day over 40 d generated by eq. (3) to evaluate if the correct value of R0 was recovered despite no information on asymptomatic transmission being provided to the estimating model. We also considered rates of immigration that vary with time (ϕ), and the influence on estimates when the latent period (1/γ) was assumed to be higher or lower than its true value. The parameter values used in the simulations were N = 1 000 000, βa = 0.3, βp = 0.3, βs = 0.8, γ = 1/3, ωa = 1/5, ωp = 1/1.1, ωa = 1/8, and q = 0.5, which give R0 = 4.1.
(3)
2.3. Onset of community spread in the models
A note is needed regarding a subtle but important difference between the estimating model and the generating model regarding the onset of community spread, tc. The generating model was implemented using demographic stochastic simulations to include the inherent randomness of disease arrival and establishment, and as such the timing of onset of community spread is random and requires a sufficient cluster of chance to allow deterministic epidemic spread to take hold and then dominate as exponential growth. In the estimating model, the dynamical model is implemented deterministically within a Bayesian model fitting framework, and in that case the onset of community spread is estimated as a parameter of the model that separates the time series into conditions that are pre-epidemic but with infected individuals arriving (t < tc) versus those after community spread begins. These phases are separated by not allowing transmission from travelers to the local community until day tc, when one infection in the community is introduced and after which it spreads. Of course subsequent introductions may also occur; however, under deterministic implementations of the model they are inconsequential to the growth of the epidemic because the exponential growth in the community quickly dominates the dynamics.
2.4. Empirical data
The model was then fitted in a Bayesian framework to timeseries of the number of new cases of COVID-19 per day in two ways, described below. The data used were timeseries of the number of new provincially confirmed cases of COVID-19 per day, based on quantitative polymerase chain reaction testing, that were maintained by Berry et al. (2020). The first cases in Canada appeared in Ontario and British Columbia in late January 2020, all associated with international travellers returning to Canada (Table 1). Implementation of physical distancing measures in Canada began the week of 16–20 March 2020, when many provinces issued declarations of emergency, and federal government interventions intensified (Ministry of Health 2020; Rodrigues 2020). We did not fit the model for the territories because there were too few data. We used 1 April 2020 as the end date of the data to which we fitted the models to constrain the data to a time period that reflects the initial transmission dynamics preceding the effects of physical distancing. This end date reflects a latent period of 2–4 d from exposure to the onset of symptoms plus about an average week delay from the onset of symptoms to an individual seeking a test to the results of the test being reported (Q. Li et al. 2020; Linton et al. 2020).
Table 1.
Estimated infection immigration rate (ϕ) | Estimated onset date of community spread (2020) | Delay from first case to estimated onset date of community spread (days) | Delay from estimated onset date of community spread to first reported case of community spread (days) | |||||||||||
---|---|---|---|---|---|---|---|---|---|---|---|---|---|---|
Province | First reported case (2020) | First reported case of community spread (2020) | Med | 2.5% | 97.5% | Med | 2.5% | 97.5% | Med | 2.5% | 97.5% | Med | 2.5% | 97.5% |
Alberta | 5 March | 15 March | 0.5674 | 0.3576 | 0.7968 | 2 March | 2 March | 2 March | −3 | −3 | −3 | 13 | 13 | 13 |
British Columbia | 28 January | 5 March | 0.0212 | 0.0027 | 0.0459 | 9 February | 4 February | 12 February | 12 | 7 | 15 | 25 | 22 | 30 |
Manitoba | 12 March | 1 April | 0.0998 | 0.0000 | 0.2203 | 15 March | 11 March | 20 March | 3 | −1 | 8 | 17 | 12 | 21 |
New Brunswick | 12 March | 30 March | 0.0548 | 0.0000 | 0.1526 | 14 March | 9 March | 16 March | 2 | −3 | 4 | 16 | 14 | 21 |
Newfoundland and Labrador | 14 March | 28 March | 0.0318 | 0.0000 | 0.1224 | 11 March | 11 March | 13 March | −3 | −3 | −1 | 17 | 15 | 17 |
Nova Scotia | 15 March | 30 March | 0.2007 | 0.0325 | 0.3969 | 13 March | 12 March | 15 March | −2 | −3 | 0 | 17 | 15 | 18 |
Ontario | 25 January | 10 March | 0.2306 | 0.1682 | 0.2973 | 8 March | 8 March | 8 March | 43 | 43 | 43 | 2 | 2 | 2 |
Prince Edward Island | 14 March | NA | 0.0648 | 0.0015 | 0.1500 | 20 March | 15 March | 25 March | 6 | 1 | 11 | NA | NA | NA |
Quebec | 27 February | 25 March | 0.1520 | 0.0468 | 0.2741 | 7 March | 7 March | 7 March | 9 | 9 | 9 | 18 | 18 | 18 |
Saskatchewan | 12 March | 24 March | 0.1881 | 0.0237 | 0.3699 | 9 March | 9 March | 12 March | −3 | −3 | 0 | 15 | 12 | 15 |
Note
Estimates are based on an assumed latent period of 3 d and infectious period of 7 d. See Supplementary Material 3 for estimates using latent periods of 2, 3, and 4 d and infectious periods of 5, 7, and 9 d.
2.5. Empirical parameter estimation
The model was implemented in the statistical programming language R version 3.6.3 (R Project for Statistical Computing, r-project.org/) using the RJags package (Plummer 2019, Supplementary Material 1), and it was fitted to the data in two ways: (i) independently for each province and (ii) hierarchically for all provinces simultaneously (described below). Initial simulation testing of the model indicated that not all parameters were estimable from early-epidemic data; this was reinforced by convergence problems when fitting the model to the empirical data. We therefore focus on the estimation of parameters that cannot be obtained from independent patient-level data—transmission coefficient (β), infection immigration rate (ϕ), and the commencement day of community transmission (Tc). Based on independent patient-level data we constrained the latent period (1/γ) to between 2 and 4 d and infectious period (1/ω) to a range between 5 and 9 d (Lauer et al. 2020; Linton et al. 2020).
The hierarchical version of the model was fitted to all provinces in Canada simultaneously by modelling the transmission coefficient and the immigration rate as hyperparameters with a Canadian-level mean and province-level random effects to account for inter-provincial variation. The day of spill-over to community spread, (Tc), was modelled as a fixed effect per province. The hierarchical model therefore provides an overall estimation of the transmission coefficient and immigration rate for Canada that consolidates within- and between-province variation in the data.
For the basic model fitted separately to each province, we supplied flat (uniform) priors for the transmission coefficient (β) and immigration rate (ϕ) that each ranged between 0 and 5, and 16 chains, with a 60 000 step adaptation, 60 000 step burn-in, and sampling of the chains for 1000 steps. The prior for the start day of community spread (Tc) was modelled as a uniform categorical variable between day 2 and the second last day of the data timeseries for each province. For the hierarchical model the transmission coefficient (β) and immigration rate (ϕ) were both modeled as log-normal distributions, with the prior for the mean uniformly distributed between −5 and 5 on a natural log scale and a standard deviation for inter-provincial variation that was uniformly distributed between zero and 1 000 000. The likelihood function modeled the observed counts of new cases per day as a Poisson process with mean rate parameter each day given by Ti(t) + Ii(t), where the subscript i indicates the focal province. The likelihood therefore does not explicitly treat cases from travellers and nontravellers separately, as this was not consistently reported in the data.
Note that this model did not explicitly consider under-reporting of cases, which could be incorporated in the model in a couple of ways—with the number of cases reported per day being proportional to the total number of infected individuals on that day or with subcompartments for infected individuals in reported and not-reported categories. In both situations, there are unestimable parameters because the true total number of infected individuals in the population is not known and other data are needed to inform testing rates and post-testing transmission rates. Our model is based on the first scenario, where the likelihood function has the number of observed cases per day matched with the number of predicted cases per day as a Poisson process, and which is an appropriate approximation of a subcompartment model if the proportion of infected individuals that get tested and test positive is low, both reasonable approximations under conditions of frequent asymptomatic infecteds and low testing rates. Our model does not consider time lags in testing and reporting or a constant under-reporting rate, as neither affect the estimation of the other parameters; introducing an under-reporting coefficient only scales the total model but does not affect the relative differences between traveller and community population parameters that determine the shape of the curve that is fitted.
3. Results
Initial model tests indicated that the latent period (1/γ) and infectious period (1/ω) were not estimable (i.e., chains did not converge) and must instead be specified. The simulation testing indicated that estimates of R0 were unbiased despite unobserved asymptomatic cases and presymptomatic transmission, and that the infection immigration rate, ϕ, was slightly underestimated (Fig. 1a). When the immigration rate was not constant but rather a wave of travellers, estimates of R0 remained unbiased but contained lower uncertainty under conditions of constant immigration (Figs. 1b and 1c). The nonconstant immigration rates also resulted in a contraction of the distribution of the date of onset of community spread, Tc, towards earlier days. Misspecification of the latent period introduced bias to the estimation of R0 such that a latent period that is too high (low) results in overestimation (underestimation), and in both cases resulted in slight overestimation of the immigration rate ϕ (Figs. 1c and 1d). There is no true value for the infectious period in the simulation testing with which to test the estimating model as the infectious period is distributed among the three infectious classes. We therefore evaluated sensitivity of estimates to the specification of the infectious period by refitting the model to the empirical data under a range of scenarios, with the latent period at 2, 3, and 4 d as well as the infectious period specified as 5, 7, and 9 d.
Fig. 1.

The fits of the models exhibited good convergence properties (Supplementary Material 2), and agreed well with the observed data (Fig. 2). The province-level parameter estimates from the hierarchical model also agreed well with the parameter estimates from the model when fitted to each province independently (Supplementary Material 3). All provincial data sets and fitted models clearly show the exponential growth that is consistent with the early epidemic phase that follows variable periods of constant rates of new cases arising from returning travellers prior to the onset of local community spread (Fig. 2). For less populated provinces, such as Nova Scotia, where case counts were low but increasing, stochastic variation arising from sampling error resulted in strong uncertainty in the case counts and the epidemic trajectories. In contrast, for more populated provinces with higher case counts, such as Ontario and Quebec, variation from stochastic sampling error was diminished as the deterministic growth of the epidemic became more dominant on variation in the number of new cases per day (Fig. 2).
Fig. 2.

The estimated rates of immigrating infection, (ϕ), varied by province (Table 1), but were not related to population size. The dates of onset of community transmission also varied (Table 1): they were estimated at 12 and 43 d following the first reported case in British Columbia and Ontario, respectively, but were estimated just before, or within a couple days after, the first reported case in six of the eight remaining provinces. Among provinces, the model estimates that the onset of community spread happened 2–25 d earlier than the first reported cases of community spread (Table 1).
Across a latent period of 2–4 d and an infectious period of 5–9 d, the basic reproductive value was commonly in the range of 3–5 among provinces, with the lowest values observed in British Columbia and the highest values observed in Ontario and Quebec (Table 2 and Fig. 3). The median reduction in transmission rate needed to obtain R0 < 1, calculated as , varied across the parameter space and among provinces from as low as 46% in British Columbia to as high as 89% in Ontario (Supplementary Material 3). Across parameter scenarios, the hierarchical model estimated an overall R0 for Canada that ranged between 3.0 (95% CI: 2.3–3.9) to 5.3 (95% CI: 3.9–7.1) for Canada (Table 3). The reduction in the transmission coefficient needed to obtain an R0 < 1 is then estimated to be in the range of 67% (95% CI: 57%–74%) to 81% (95% CI: 0.74–0.86) (Table 3).
Table 2.
Transmission coefficient | Basic reproductive value (R0) | Transmission reduction for R0 < 1 | |||||||
---|---|---|---|---|---|---|---|---|---|
Province | Med | 2.5% | 97.5% | Med | 2.5% | 97.5% | Med | 2.5% | 97.5% |
Alberta | 0.524 | 0.514 | 0.535 | 3.669 | 3.595 | 3.745 | 0.727 | 0.722 | 0.733 |
British Columbia | 0.321 | 0.298 | 0.339 | 2.244 | 2.083 | 2.374 | 0.554 | 0.520 | 0.579 |
Manitoba | 0.624 | 0.435 | 0.997 | 4.368 | 3.047 | 6.977 | 0.771 | 0.672 | 0.857 |
New Brunswick | 0.451 | 0.357 | 0.559 | 3.157 | 2.502 | 3.914 | 0.683 | 0.600 | 0.745 |
Newfoundland and Labrador | 0.563 | 0.514 | 0.638 | 3.944 | 3.598 | 4.468 | 0.746 | 0.722 | 0.776 |
Nova Scotia | 0.590 | 0.523 | 0.703 | 4.130 | 3.658 | 4.920 | 0.758 | 0.727 | 0.797 |
Ontario | 0.979 | 0.971 | 0.988 | 6.854 | 6.794 | 6.913 | 0.854 | 0.853 | 0.855 |
Prince Edward Island | 0.518 | 0.003 | 1.177 | 3.628 | 0.022 | 8.237 | 0.724 | 0.000 | 0.879 |
Quebec | 1.020 | 1.013 | 1.026 | 7.139 | 7.093 | 7.185 | 0.860 | 0.859 | 0.861 |
Saskatchewan | 0.488 | 0.443 | 0.549 | 3.415 | 3.102 | 3.842 | 0.707 | 0.678 | 0.740 |
Note
Estimates are given for an assumed latent period of 3 d and infectious period for 7 d. See the supplement for estimates under assumed latent periods of 2, 3, and 4 d and infectious periods of 5, 7, and 9 d.
Fig. 3.

Table 3.
Transmission coefficient | Basic reproductive value | Transmission reduction for R0 < 1 | ||||||||
---|---|---|---|---|---|---|---|---|---|---|
Latent period (d) | Infectious period (d) | Med | 2.5% | 97.5% | Med | 2.5% | 97.5% | Med | 2.5% | 97.5% |
2 | 5 | 0.60 | 0.46 | 0.78 | 2.99 | 2.31 | 3.90 | 0.67 | 0.57 | 0.74 |
7 | 0.52 | 0.38 | 0.70 | 3.61 | 2.63 | 4.87 | 0.72 | 0.62 | 0.79 | |
9 | 0.47 | 0.34 | 0.67 | 4.24 | 3.02 | 6.06 | 0.76 | 0.67 | 0.83 | |
3 | 5 | 0.67 | 0.53 | 0.83 | 3.33 | 2.63 | 4.17 | 0.70 | 0.62 | 0.76 |
7 | 0.58 | 0.44 | 0.78 | 4.07 | 3.06 | 5.44 | 0.75 | 0.67 | 0.82 | |
9 | 0.53 | 0.38 | 0.73 | 4.81 | 3.46 | 6.59 | 0.79 | 0.71 | 0.85 | |
4 | 5 | 0.74 | 0.59 | 0.94 | 3.70 | 2.97 | 4.68 | 0.73 | 0.66 | 0.79 |
7 | 0.65 | 0.49 | 0.84 | 4.52 | 3.42 | 5.88 | 0.78 | 0.71 | 0.83 | |
9 | 0.59 | 0.43 | 0.79 | 5.30 | 3.88 | 7.12 | 0.81 | 0.74 | 0.86 |
Note
Estimates are provided for all combinations of a latent period of 2, 3, and 4 d and infectious periods of 5, 7, and 9 d.
4. Discussion
Our results indicate that several epidemiological parameters—the transmission coefficient, basic reproductive value, immigration rate of infection, and commencement of community spread—are estimable from time-series of new reported cases prior to public health intervention despite unobserved asymptomatic cases and asymptomatic and pre-symptomatic transmission. The accuracy of these estimates depends; however, on the accuracy with which the population-average latent period and infectious period are specified, with potential biases arising from misspecification. The estimated parameters indicate that the initial process of establishment and spread of SARS-CoV-2 in provinces of Canada is characterized by high uncertainty of onset arising from demographic stochasticity in the commencement of community spread as well as from stochastic sampling error from testing. That uncertainty creates time lags among establishment, detection, and intervention during which the virus has a high rate of community spread. The estimated R0 implies that interventions such as physical distancing or vaccination would have needed to reduce transmission rate by an average of 46%–89% across Canada to contain the virus under the conditions of the initial outbreak. To halt the current epidemic, the threshold reduction in transmission rate needed will be lower, due to the depletion of susceptibles from immunity from previous infection. However, it is not currently known the degree of immunity conferred by previous infection with SARS-Cov2 and potentially other coronavirus nor the true prevalence of infection in the population.
Since SARS-CoV-2 has already spread in Canada, the realized growth rate, Re, will be less than R0 proportional to the extent of depletion of susceptibles due to immunity and to changes in social behaviours and environment relative to the conditions during initial invasion. As such, the estimated R0 and associated thresholds for control (θ) provide a reference point against which current conditions or intervention scenarios can be compared. Our estimates therefore provide threshold levels of intervention strength that need to be met or exceeded to ensure that Re < 1, which is needed for containment so long as the following conditions are met: (i) social behaviour maintains contact rates less than that during initial transmission, (ii) there is some level of immunity conferred by previous infection, (iii) changes in environmental conditions such as climate do not increase spread, and (iv) the virus does not evolve higher transmission rates or infectious period. If those conditions are met then the effective reproduction rate Re is likely to be less than R0, and (θ) provides a minimum target level for control above which halting spread is likely achievable.
The early local spread of SARS-CoV-2 in Hubei Province in China was initially estimated as having an R0 in the range of 1.4–3.9 (Q. Li et al. 2020), whereas latter re-analyses have estimated R0 to be as high as 3.8–8.9 (Sanche et al. 2020) and 5.7–7.2 (Tang et al. 2020). The R0 for Italy has been estimated at 3.5–3.8 (Gatto et al. 2020) and then 4.7–11.4 internationally (Kochańczyk et al. 2020). Our results have empirically estimated that R0 is between a minimum of 3.0 (95% CI: 2.3–3.9) to a maximum of 5.3 (95% CI: 3.9–7.1) for Canada as a whole, depending on assumption of the latent and infectious periods. However, we also estimated substantial variation among provinces in R0, which falls within the range of R0 estimates for other countries, and is consistent with the variation in rapid increase among provinces and may reflect interprovincial variation in public health responses to SARS-CoV-2 for which British Columbia has been lauded. However, it is also possible that random unbiased error in the estimating model, as indicated in the simulation testing, may have simply by chance alone contributed to among-province variation in the parameter estimates. Furthermore, variation among provinces in testing capacity or specificity may contribute to among-province variation in R0.
Theoretical studies using compartmental models, similar to the models we worked with but often with much more complex transmission dynamics, usually need to specify R0 and (or) the transmission coefficient, and have assumed R0 = 2.5 in models of Canada (Shoukat et al. 2020) and the United States (Moghadas et al. 2020). Our results have empirically estimated that, overall for Canada, there is a higher level of contagiousness than assumed in these models that assume R0 = 2.5. Consequently, models that assume R0 = 2.5 may have underestimated the strength of intervention necessary to avoid overwhelming health care systems. In addition, our estimates suggest that transmission needs to be reduced by 46%–89% to obtain R0 < 1, which means obtaining herd immunity requires 46%–89% immunity in the population compared with 60% if R0 = 2.5. However, it is important to note that our estimates for R0 are contingent on our specification of the latent and infectious periods. We implicitly assumed the distribution of the latent and infectious periods were exponential, which if relaxed to allow for gamma distributed periods by including subcompartments in exposed and infectious classes, can also reduce the value of R0 (Roberts and Heesterbeek 2007). Overall, despite these uncertainties, our estimates reinforce the high level of contagiousness of SARS-CoV-2.
The parameter estimates from our analysis provide empirical reference points against which estimates for the effective reproductive rate (Re) under future conditions can be compared to evaluate the effectiveness of interventions. For example, analysis of the outbreak in Germany shows that interventions reduced the exponential growth rate 4.8-fold from 0.43 to 0.09 (Dehning et al. 2020), and our methods can be similarly expanded to accommodate shifts in transmission rate in response to interventions. Our estimates also allow for revised assessment and planning for testing, case tracking, and containment as the epidemic unfolds, because the level of contagiousness may be higher than previously thought. Because it is not possible to estimate the contribution of asymptomatic and presymptomatic transmission from our model given the available data, there is clearly a need for future analyses to parse out their contributions to the overall transmission rate and R0 to inform mathematical models used for scenario analyses and public health planning. Given the contingency of our estimates on two key parameters that must be specified—latent period and infectious period—a future direction is to improve the precision and accuracy of those parameters in the context of community spread rather than from individual data from patients in hospitalized settings.
One limitation of this study is that it does not account for within-province variation in SARS-CoV-2 spread, for example, because of geography, density, demography, or occupation. Rather, our estimates are at the population level. Other analyses of in R0 account for within-population heterogeneity by combining exponential growth, the generation time of the virus, and a dispersion parameter for the distribution of generation time (Park et al. 2020). Such heterogeneity is important to quantify and needs to be coupled with intrapopulation data to identify the conditions of superspreading events, which would help prioritize intervention strategies to contain spread. As most compartment epidemiological models exhibit exponential growth in the early phases of an epidemic, it is therefore not surprising that the model achieved a good fit to the data. Nonetheless, the parameters estimated via this exercise should be useful for understanding the level of contagiousness of the virus and magnitude of sustained interventions needed for its containment as evaluated by models for the latter stages of the epidemic that allow calculation of Re.
Another limitation is that the data on the number of new cases per day, to which the model is fitted, may not be representative of the population due to limited testing capacity or prioritized testing of high-risk subpopulations. Further, changes in infection testing methods and reporting procedures midway through the epidemic add variation to the data such as the high number of cases reported in Quebec on 22 March 2020 due to removing the requirement for secondary confirmation or in Ontario on 1 April 2020 due to a switch to reporting from public health units. Our model does not account for such heterogeneities or under-reporting in the data. However, our estimates should be representative under conditions prior to physical distancing assuming that case numbers were low relative to testing capacity and reflected the propensity of symptomatic and exposed individuals seeking tests, proportional to the prevalence of the virus in the overall population. Finally, another limitation of our study is a possible bias in under- and overestimating R0, due to misspecification of the latent period and infectious period. We therefore provided estimates of the epidemiological parameters under a range of assumed values for latent period and infectious period that cover the possible realistic range (see Supplementary Material).
In Canada, and in the absence of intervention, SARS-CoV-2 is estimated to be highly contagious. To contain the epidemic in Canada, the model estimates that the degree of sustained intervention needed is a 46%–89% reduction in transmission rate relative to conditions early in the outbreak, which could come from a combination of behavioural change, immunity from previous exposure, and future interventions such as vaccination. Provinces in Canada vary in policy-relevant ways regarding SARS-CoV-2 and the strength of interventions that are needed for containment. There has clearly been a slowdown in most provinces in the rate of new cases per day in response to physical distancing; as provinces continue to ease restrictions, our results provide guidance and reference points against which to measure the success of interventions as well as how fast infection could spread should conditions return to early-epidemic levels.
Acknowledgements
We are grateful for discussions with Marie-Josee Fortin that helped improve the work. This work was supported by a Canada Research Chair to MK, NSERC Discovery Grants (MK, NM, PM), Canada Foundation for Innovation (CFI) John R. Evans Leaders Fund (MK, PM), and Ministry of Research, Innovation and Sciences (MRIS) Ontario Research Fund (MK, PM), NSERC Doctoral Grant (AG), and a Vanier Canada Graduate Scholarship (IB, J-PRS).
References
Allen LJS, and van den Driessche P. 2008. The basic reproduction number in some discrete-time epidemic model. Journal of Difference Equations and Applications, 14(10): 1127–1147.
Anderson RMM, and May RMM. 1991. Infectious diseases of humans. Oxford University Press, Oxford, UK.
Bartlett MS. 1964. The relevance of stochastic models for large-scale epidemiological phenomena. Journal of the Royal Statistical Society. Series C (Applied Statistics), 13(1): 2–8.
Berry I, Soucy JR, Tuite A, and Fisman D. 2020. Open access epidemiologic data and an interactive dashboard to monitor the COVID-19 outbreak in Canada. Canadian Medical Association Journal, 192(15): E420.
Dehning J, Zierenberg J, Spitzner PF, Wibral M, Neto JP, Wilczek M, et al. 2020. Inferring change points in the spread of COVID-19 reveals the effectiveness of interventions. Science, 369(6500): eabb9789.
Gatto M, Bertuzzo E, Mari L, Miccoli S, Carraro L, Casagrandi R, et al. 2020. Spread and dynamics of the COVID-19 epidemic in Italy: effects of emergency containment measures. Proceedings of the National Academy of Sciences of the United States of America, 117(19): 10484–10491.
He X, Lau EH, Wu P, Deng X, Wang J, Hao X, et al. 2020. Temporal dynamics in viral shedding and transmissibility of COVID-19. Nature Medicine, 26(5): 672–675.
Kochańczyk M, Grabowski F, and Lipniacki T. 2020. Super-spreading events initiated the exponential growth phase of COVID-19 with R0 higher than initially estimated. Royal Society Open Science, 7: 200786.
Lauer SA, Grantz KH, Bi Q, Jones FK, Zheng Q, Meredith HR, et al. 2020. The incubation period of coronavirus disease 2019 (COVID-19) from publicaly reported confirmed cases: estimation and application. Annals of Internal Medicine, 172(9): 577–582.
Li Q, Guan X, Wu P, Wang X, Zhou X, Tong Y, et al. 2020. Early transmission dynamics in Wuhan, China, of novel coronavirus-infected pneumonia. The New England Journal of Medicine, 382(13): 1199–1207.
Li R, Pei S, Chen B, Song Y, Zhang T, Yang W, et al. 2020. Substantial undocumented infection facilitates the rapid dissemination of novel coronavirus (SARS-CoV-2). Science, 368(6490): 489–493.
Linton NM, Kobayashi T, Yang Y, Hayashi K, Akhmetzhanov AR, Jung S, et al. 2020. Incubation period and other epidemiological characteristics of 2019 novel coronavirus infections with right truncation: a statistical analysis of publicly available case data. Journal of Clinical Medicine, 9(2): 538.
Ministry of Health. 2020. Enhanced measures to protect Ontarians from COVID-19 [online]: Available from news.ontario.ca/mohltc/en/2020/03/enhanced-measures-to-protect-ontarians-from-covid-19.html.
Moghadas SM, Shoukat A, Fitzpatrick MC, Wells CR, Sah P, Pandey A, et al. 2020. Projecting hospital utilization during the COVID-19 outbreaks in the United States. Proceedings of the National Academy of Sciences of the United States of America, 117(16): 9122–9126.
Nicola M, Alsafi Z, Sohrabi C, Kerwan A, Al-Jabir A, Iosifidis C, et al. 2020. The socio-economic implications of the coronavirus and COVID-19 pandemic: a review. International Journal of Surgery, 78: 185–193.
Park SW, Bolker BM, Champredon D, Earn DJD, Li M, Weitz JS, et al. 2020. Reconciling early-outbreak estimates of the basic reproductive number and its uncertainty: framework and applications to the novel coronavirus (SARS-CoV-2) outbreak. Journal of the Royal Society Interface, 17(168): 20200144.
Plummer M. 2019. rjags: Bayesian graphical models using MCMC. R package version 4-10 [online]: Available from cran.r-project.org/web/packages/rjags/index.html.
Ridenhour B, Kowalik JM, and Shay DK. 2014. Unraveling R0: considerations for public health applications. American Journal of Public Health, 104(2): E32–E41.
Roberts M, and Heesterbeek JAP. 2007. Model-consistent estimation of the basic reproduction number from the incidence of an emerging infection. Journal of Mathematical Biology, 55: 803–816.
Rodrigues G. 2020. Ontario government declares state of emergency [online]: Available from globalnews.ca/news/6688074/ontario-doug-ford-coronavirus-covid-19-march-17/.
Sanche S, Lin Y, Xu C, Romero-Severson E, Hengartner N, Ke R, et al. 2020. High contagiousness and rapid spread of severe acute respiratory syndrome coronavirus 2. Emerging Infectious Diseases, 26(7): 1470–1477.
Shoukat A, Wells CR, Langley JM, Singer BH, Galvani AP, Moghadas SM, et al. 2020. Projecting demand for critical care beds during COVID-19 outbreaks in Canada. Canadian Medical Association Journal, 192(19): E489–E496.
Tang B, Wang X, Li Q, Bragazzi NL, Tang S, Xiao Y, et al. 2020. Estimation of the transmission risk of 2019-nCov and its implication for public health interventions. Journal of Clinical Medicine, 9(2): 462.
Tuite AR, Fisman DN, and Greer AL. 2020. Mathematical modelling of COVID-19 transmission and mitigation strategies in the population of Ontario, Canada. Canadian Medical Association Journal, 192(19): E497–E505.
Wilson M, and Chen L. 2020. Travellers give wings to novel coronavirus (2019-nCoV). Journal of Travel Medicine, 27(2): 1–3.
World Health Organization. 2020. Coronavirus disease 2019 (COVID-19) situation report-38 [online]: Available from who.int/docs/default-source/coronaviruse/situation-reports/20200227-sitrep-38-covid-19.pdf?sfvrsn=47fdaf7_4.
Supplementary materials
Supplementary Material 1 (ZIP / 165 KB)
- Download
- 165.67 KB
Supplementary Material 2 (PDF / 11.2 MB)
- Download
- 11.21 MB
Supplementary Material 3 (XLSX / 27.8 KB)
- Download
- 27.86 KB
Information & Authors
Information
Published In
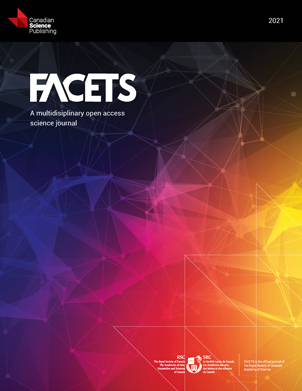
FACETS
Volume 6 • Number 1 • January 2021
Pages: 180 - 194
Editor: David Moher
History
Received: 14 July 2020
Accepted: 9 November 2020
Version of record online: 11 February 2021
Copyright
© 2021 Krkošek et al. This work is licensed under a Creative Commons Attribution 4.0 International License (CC BY 4.0), which permits unrestricted use, distribution, and reproduction in any medium, provided the original author(s) and source are credited.
Data Availability Statement
All relevant data are within the paper and in the Supplementary Material.
Key Words
Sections
Subjects
Authors
Author Contributions
MK, NM, and PKM conceived and designed the study.
MK, MJ-C, KW, IB, and J-PRS performed the experiments/collected the data.
MK, MJ-C, KB, AG, SP, EWT, NM, and PKM analyzed and interpreted the data.
MK contributed resources.
MK, KW, IB, KB, AG, LK, SP, DS, JSVS, EWT, NM, and PKM drafted or revised the manuscript.
Competing Interests
The authors have declared that no competing interests exist.
Metrics & Citations
Metrics
Other Metrics
Citations
Cite As
Martin Krkošek, Madeline Jarvis-Cross, Kiran Wadhawan, Isha Berry, Jean-Paul R. Soucy, Korryn Bodner, Ariel Greiner, Leila Krichel, Stephanie Penk, Dylan Shea, Juan S. Vargas Soto, Ed W. Tekwa, Nicole Mideo, and Péter K. Molnár. 2021. Establishment, contagiousness, and initial spread of SARS-CoV-2 in Canada. FACETS.
6: 180-194.
https://doi.org/10.1139/facets-2020-0055
Export Citations
If you have the appropriate software installed, you can download article citation data to the citation manager of your choice. Simply select your manager software from the list below and click Download.
Cited by
1. Social, economic, and environmental factors influencing the basic reproduction number of COVID-19 across countries