Monitoring social–ecological networks for biodiversity and ecosystem services in human-dominated landscapes
Abstract
The demand the human population is placing on the environment has triggered accelerated rates of biodiversity change and created trade-offs among the ecosystem services we depend upon. Decisions designed to reverse these trends require the best possible information obtained by monitoring ecological and social dimensions of change. Here, we conceptualize a network framework to monitor change in social–ecological systems. We contextualize our framework within Ostrom’s social–ecological system framework and use it to discuss the challenges of monitoring biodiversity and ecosystem services across spatial and temporal scales. We propose that spatially explicit multilayer and multiscale monitoring can help estimate the range of variability seen in social–ecological systems with varying levels of human modification across the landscape. We illustrate our framework using a conceptual case study on the ecosystem service of maple syrup production. We argue for the use of analytical tools capable of integrating qualitative and quantitative knowledge of social–ecological systems to provide a causal understanding of change across a network. Altogether, our conceptual framework provides a foundation for establishing monitoring systems. Operationalizing our framework will allow for the detection of ecosystem service change and assessment of its drivers across several scales, informing the long-term sustainability of biodiversity and ecosystem services.
1. Introduction
Humans and nature are inextricably connected through ecosystem benefits and ecosystem services (ES(s) hereafter). The demands of a growing human population for provisioning ESs (e.g., food production, energy, timber) have triggered the worldwide erosion of biodiversity (Ceballos et al. 2015; IPBES 2019), the increase in species invasions, the loss of ecosystem functions (Cardinale et al. 2011), and trade-offs among the ESs we depend on (Cord et al. 2017a; Martín-López et al. 2014). Particularly in the past half century, 75% of basic ESs (i.e., provisioning, regulating, supporting, and cultural) are estimated to be in a degraded state (Díaz et al. 2019). Our capacity to make decisions that attenuate and reverse these impacts on biodiversity and ESs loss can be improved via the implementation of adaptive monitoring strategies (IPBES 2019; MA 2005).
The need to monitor change in biodiversity and ESs has been identified at regional and global scales (GEO BON 2017). Commitments to the international (i.e., Convention on Biological Diversity) and national biodiversity and sustainability goals (e.g., Canada’s Sustainable Development Goals) will likely see renewed investment in monitoring capacity in the coming decade. Despite the surge in investment in the monitoring of biodiversity and ESs across spatial scales, integrated monitoring schemes that evaluate the ecological and social dimensions of change at different spatial scales still remain scarce (Geijzendorffer and Roche 2013; Geijzendorffer et al. 2017b; Kühl et al. 2020). Likewise, while current ecological monitoring captures important dynamics in particular species and ES stocks, they generally fail to monitor the co-dependence, or connectivity, among ecosystems and human uses (e.g., ES flow, drivers of change, beneficiaries, demand; for review see Kluger et al. 2020), which is essential if we are to assess the long-term sustainability of biodiversity and ESs under changing or novel environmental and social conditions (Tallis et al. 2012).
Monitoring biodiversity and ESs in spatially extended social–ecological systems (hereafter SES) is a complex challenge (Dee et al. 2017; Bodin et al. 2019). It is clear no single strategy for ecosystem monitoring and management can solve complex and context-dependent environmental problems (e.g., Ostrom 2007; McGinnis & Ostrom 2014). Nevertheless, network-based approaches can help assess how changes within and between components of ecological and social systems affect ESs (Dee et al. 2017). Thus, a monitoring design based on networked SES can provide critical understanding of how changing interconnections in SES can generate risks for ES supply or human well-being (e.g., expressed as large temporal fluctuations; Duncan et al. 2015; Henderson et al. 2016; Tallis et al. 2012). Through this approach, monitoring can help identify early warning indicators of ecosystem degradation or collapse, tipping points in SES and rates at which irreversible loss is being approached (Bauch et al. 2016; Gonzalez et al. 2017; McCann et al. 2020; Scheffer et al. 2009; Tallis et al. 2012). Network-based monitoring can also be used to inform risk-mitigation strategies that keep SES networks within a sustainable operating space (Gonzalez et al. 2017). Yet, this present work stresses that network-based monitoring designs should be spatially explicit to characterize and quantify spatially heterogeneous responses to drivers of change (e.g., climate warming, increase in ES demand). Monitoring adaptively across spatial networks can account for nonstationary change and interdependencies and provide the information needed to attain short and long-term outcomes through adaptive management practices (Lindenmayer and Likens 2009).
The future of nature and human well-being relies on our capacity to embrace the complexities of SES and provide scalable evidence-based management strategies. One such initiative is NSERC ResNet (Natural Sciences and Engineering Research Council, nsercresnet.ca), a network for managing, modelling, and monitoring ESs across Canada. ResNet is structured across three themes (i.e., management, modelling, and monitoring) and six Canadian working landscapes: agriculture in the Bay of Fundy dykelands, the Prairies, and boreal Northwestern Territories; agriculture and peat mining in Québec; energy development in Northern British Columbia and Alberta; and fisheries in the Pacific coast. Landscapes share common ESs at the regional and federal levels and contribute with unique ESs at the provincial level (Table 1). The goal of maintaining long-term sustainability and resilience of biodiversity and ESs requires integrative monitoring that generates information to support management decisions across spatial and temporal scales. Hence, as a part of ResNet’s monitoring theme, we conceptualize a multilayer and multiscale network framework for monitoring biodiversity and ESs in SES. We illustrate our proposed framework with a conceptual case study on the provisioning ES of maple syrup production in the landscape of Québec. Yet, our framework can be applied to different socio-ecological contexts and sets of ESs across and beyond ResNet’s landscapes. We also outline the need to use monitoring, statistical, and modelling frameworks to integrate quantitative and qualitative expectations derived from experts and stakeholders to generate a causal understanding of change across sites of the SES. The future operationalization of our proposed framework will allow for the detection of unintended spatial and temporal feedback arising from interlinked social and ecological interactions. Establishing monitoring to integrate evidence into decision-making is essential if we are to achieve the long-term sustainability of biodiversity and ESs into an uncertain future, and here we provide a first step towards this goal.
Table 1.
Ecosystem service | Subcategory | Distribution | Interaction | Space | Time |
---|---|---|---|---|---|
Provisioning services | |||||
Food | Crop | Regional | Local | Static | Dynamic |
Fisheries | Provincial | Local | Dynamic | Static | |
Freshwater | — | Federal | Local | Static | Static |
Wild foods | Plants | Provincial | Local | Static | Dynamic |
Animals | Provincial | Local | Dynamic | Dynamic | |
Biological raw materials | Timber | Provincial | Local | Static | Static |
Energy | — | Provincial | Local | Static | Static |
Regulating services | |||||
Climate regulation | Carbon | Federal | Diffuse | Static | Static |
Disease regulation | — | Provincial | Diffuse | Dynamic | Dynamic |
Natural hazard regulation | Flood and storm protection | Federal | Diffuse | Static | Static |
Cultural services | |||||
Recreation | — | Federal | Local | Static | Static |
Hunting | — | Provincial | Local | Dynamic | Dynamic |
Aesthetic/ethical value | — | Federal | Diffuse | Static | Static |
Note
The ecosystem services (ESs) are presented following an adapted version of the framework proposed by MA (2005). Within each ES category (i.e., provisioning, regulating, and cultural services) there can be multiple ESs, which can be further divided into Subcategories. Distribution refers to whether an ES is relevant at all six ResNet landscapes (Federal), at a subset of landscapes (Regional), or at a unique landscape (Provincial). Each ES in its most specific category is characterized relative to its supply interaction as local or diffuse and occurrence across space and time as static or dynamic.
1.1 A spatial network monitoring framework for social–ecological systems
Robust monitoring of biodiversity and ESs relies on rigorous design protocols and analytical methods to detect trends across scales, account for potential sources of error, and be adaptive as knowledge and objectives evolve (Larsen et al. 2001; Lindenmayer and Likens 2009; Yoccoz et al. 2001). Particularly for ESs, monitoring must also rely on a comprehensive description of the SES relative to the monitoring questions or objectives (Kluger et al. 2020; Kühl et al. 2020). Past studies have proposed different frameworks to conceptualize (Ostrom 2007; McGinnis & Ostrom 2014), analyze (Bodin et al. 2019), and operationalize (Dee et al. 2017) ES networks of SES under varying objectives (Table 2). For example, Bodin et al. (2019) discussed types of causal interactions in SES networks to understand how interdependencies drive changes, while Dee et al. (2017) presented steps to build a SES network for ES management. However, the challenge of monitoring the joint change in social and ecological networks has, to our knowledge, not been addressed in previous frameworks. Hence, we build upon former studies to conceptualize a spatial network framework for monitoring ESs in SES.
Table 2.
Previous studies | Objective | Nodes | Links | Spatially explicit | Multilayer | Multiscale | Monitoring |
---|---|---|---|---|---|---|---|
Ostrom 2007 and McGinnis and Ostrom 2014 | Analyse interactions and outcomes | Resource system, Resource units, Governance system, Actors | Action situations, feedbacks | No | No | No | No |
Guerrero et al. 2015 | Assess cross-scale collaboration | Stakeholders | Collaboration | No | No | Yes | No |
Dee et al. 2017 | Assess management alternatives | ES, ES providers, ES beneficiaries | Various ecological and social interactions | No | Meta-network | No | No |
Gonzalez et al. 2017 | Design and protection of sustainable ecological networks | Habitat patches or ecosystem types, and species | Movement, and species interactions | Yes | No or Yes (Meta-network) | Yes | Yes |
Sayles and Baggio 2017 | Analyse social–ecological scale misalignments | Watersheds, Restoration organizations | Relationships across scales | Yes | Yes | Yes | No |
Bodin et al. 2019 | Facilitate cross-case comparison | Ecological and social components or aggregated components | Interactions | No or Yes | No or Yes (Multiplex/Multilevel) | Yes | No |
Keyes et al. 2021 | Predict ES vulnerability to species loss | Species, Ecosystem services | Species interactions, ES provisioning | No | Yes | No | No |
Note
Studies were characterized by their objective, how nodes and links were defined, whether its network components were spatially explicit and its network structure multilayer, and whether multiscale and monitoring implications were discussed.
The spatial network monitoring framework we propose aims to establish a spatially explicit and cross-scale description of how drivers of change may impact biodiversity and ESs in SES. Our framework is envisioned to address monitoring questions pertaining to the sustainability of the SES by providing a comprehensive blueprint of the SES. The steps of our framework are: (step 1) identify the SES and its components relative to the ES(s) of concern (section 2.1); (step 2) define the SES network composition and spatially explicit network structure (section 2.2); (step 3) identify the scale mismatches in the monitoring design of an SES network (section 3.1); (step 4) identify the drivers of change in the SES (section 3.2); (step 5) determine the variables, scales, and frequencies of monitoring needed to capture the range of variability seen across the SES network (section 3.3); and (step 6) select appropriate analytical tools and integrate monitoring with management into an adaptive cycle (section 4). Here, we provide practical examples of how to implement steps 1–4 and discuss future avenues to address steps 5–6.
2. Social–ecological systems and their multilayered spatial network structure
2.1. Identify the social–ecological system
The SES framework proposed by Ostrom (2007) and updated by McGinnis and Ostrom (2014) provides a natural context to identify the components and interactions in an SES that must be monitored in the long-term. At its core, Ostrom’s SES framework (Fig. 1a) is defined by a suite of direct and indirect interactions among the top-tier categories of ecological “Resource systems” and the “Resource units” nested in that system, and social “Governance systems” and the “Actors” they regulate. Central to the framework are the focal “Action situations” that define how these top-tier categories interact to result in social and ecological outcomes, represented as feedbacks from “Action situations” to each of the ecological and social top-tier categories.
Fig 1.

Here, we use the Ostrom (2007) SES framework as foundation in the first step of our framework, which is a coarse level identification of the top-tier categories and its interactions relative to a focal ES (Fig. 2a). The resource system can be identified as the ecological system (e.g., ecosystem, landscape, or ecoregion) that provides the ES, including the species that indirectly contribute to the ES (e.g., interacting species); the resource units can be identified as the species that directly provide the ES; the Governance system can be identified as those who elaborate and maintain the rules and regulations that influence how the social system interacts with the ES; and the Actors can be identified as those who interact with the ES (e.g., through its production, extraction, distribution, use, or consumption).
Fig 2.

2.2. The spatial multilayer network of a social–ecological system
In the second step of our framework, we further extend the Ostrom’s SES framework to represent the top-tier categories described above as layers in a multilayered network, and the action situations as links within and across layers, occurring over space and time (Fig. 2b-c). While a single layer network describes interactions between nodes (e.g., competition among species), a multilayer network represents different node types, organized in separate layers, and connected through different types of interactions (e.g., ecological and social). Here, we represent the resource system as the ecological ecosystem layer, the resource units as the ecological resource layer, the governance system as the social governance layer, and the actors as the social actors layer. We propose an additional ecological layer to represent the ES.
Once the network layers have been determined, the spatial location of the respective nodes within each layer must be defined (i.e., ecosystem, resource, ES, governance, and actor nodes). The presence of particular node types and interactions between nodes, as well as their scales will be SES specific and time dependent. Hence, a well-designed SES monitoring scheme requires that we adapt the monitoring of the SES as the network components and its spatial structure change over time. Similar to mentioned above, the network nodes are defined relative to the focal ES. The ecosystem nodes (e.g., forest stands) indirectly contribute to ESs through, for example, habitat provisioning and species interactions. The resource nodes represent the resource units producing sets or bundles of ESs directly or through ecosystem functions. In turn, the ES nodes represent the ES stocks, or natural capital stocks, of various types and values to human actors in the system. In defining the nodes, the spatial scale, location, and extent of individual nodes should be identified. For example, in the case of the provisioning ES of a fishery, ecosystem nodes may be represented as coastal regions and the resource nodes as harvestable stocks of fish present in different locations. Some of these stocks are viable and harvestable and so can be defined as ES nodes. Representing the ES stock this way is particularly advantageous when multiple resource species interact to contribute to a single ES as pointed out by Dee et al. (2017), and when ES stock covaries with ecosystem nodes across locations and contexts. Another conceptual example we develop below considers multiple ecosystem nodes representing patches of forest stands composed of different tree species, some of which provide a resource (i.e., sap) that can be defined as an ES node to a particular set of actors. Altogether, the ecological layers form a nested hierarchy, in which ecological nodes interact within and across ecological layers in a variety of ways, including species interactions, ecosystem functions, spatial connectivity, or species movement between nodes.
The ecological network layers are linked to the social network layers through interactions with its governance and actor nodes. The governance nodes regulate how social and ecological systems interact and can represent a variety of governance modes (Metzger et al. 2020), such as nodes that directly manage ecological systems (e.g., through protected areas), provide financial incentives for ES (e.g., payments for ES), or represent collective agreements on ES extraction limits (i.e., community-based interventions). The actor nodes are as diverse as the values provided by the focal ES. For instance, actor nodes may represent farmers who produce food crops or fishers and loggers who harvest natural resources. In this case, the interactions between actor and ES nodes represent a rate of ES flow or supply, as in Dee et al. (2017). Alternatively, in the case of cultural ESs, actor nodes may represent the users of a recreational area and the actor–ES interaction may represent relational values (Chan et al. 2018). The social interactions between governance and actors as well as within its respective layers will vary with the social processes relevant to the focal ES. The typology of social interactions is diverse, and may include collaborations, common agreements, kinship or group membership, knowledge or information exchange, as well as power and conflict of interests (Barnes et al. 2019; Bodin 2017; Bodin and Tengö 2012; Minnes et al. 2020).
Our framework shares features of other network frameworks for SES that have been described recently (Table 2). For example, Dee et al. (2017) also proposed to represent ESs as network nodes linked to ecological and social nodes which directly and indirectly interact with the ES. Along with Bodin et al. (2019), both approaches emphasize the need to integrate ecological and social networks when studying ESs. Moreover, Bagstad et al. (2013) and Gonzalez et al. (2017) explicitly discussed the importance of spatial dynamics for ESs and sustainability. Despite the important contributions of previous work, the matter of providing a systematic framework to guide ES monitoring across scales has not yet been addressed. Here, we provide the first step towards the development of monitoring guidelines, which is to establish the conceptual foundation that will guide the operationalization of monitoring systems. We emphasize that this is an important step as a comprehensive description of the SES is necessary if we are to be transparent about future simplifying assumptions on the SES complexity.
3. Monitoring to detect change in multilayer and multiscale social–ecological systems
Detecting change in ESs variables across an SES network relies on monitoring the states of the ecological and social nodes and the interactions that connect nodes within and across network layers. For example, the relative species composition of a forest stand can be representative of different ecological states (e.g., high or low richness, diversity, or evenness), which will influence both ecological interactions and the ES supply to the social network. Not all nodes and links will be “active” at any one time, and the spatial pattern of nodes and links can be dynamic through time. Thus, network-based monitoring can be viewed as an adaptive layer of observations and measurements designed to infer the changing relationships mediating the dynamics of the SES network over space and time. Because network components occur and interact across different scales of space and time and change according to different drivers, monitoring requires identifying the scale mismatches in an SES network and the drivers of ES change.
3.1. Scale mismatches in the monitoring design of an SES network
Properly monitoring an SES network requires that we identify its cross-scale dynamics and the potential scale mismatches occurring among ecosystem, resource, ES, governance, and actor nodes (Raudsepp-Hearne and Peterson 2016; Lindborg et al. 2017; Sun et al. 2019), all of which have their own “shed” (i.e., spatial zone of influence and temporal dynamics). Scale mismatches are typically referred to as misalignments between SES components (e.g., poor overlap between wild bee populations and crop or jurisdiction and management area; González-García et al. 2020), which may compromise interactions and ES provisioning (Winkler et al. 2021). In the case of monitoring, scale mismatches are particularly relevant as they may give rise to misalignments between the units of measurement of the different processes or entities. Thus, the presence of scale mismatches should be determined to inform the design of a monitoring system and selection of the appropriate indicator variables.
Here, we focus on two dimensions of scale mismatches—spatial and temporal—and discuss three key scale mismatches: network structure, ES properties, and network dynamics. A network structure mismatch may occur when network nodes operating at different scales seek to interact. In the absence of a network structure mismatch, actor nodes may interact locally with specific ES nodes (e.g., food production, recreation). A network structure mismatch arises when for instance, actor nodes perceive the ES benefits diffusely (e.g., climate regulation, ethical value of biodiversity). Similarly, a governance node may need to manage an ecological system regionally, hence its decisions must overcome a network structure mismatch to take local dynamics into account. For example, the establishment of regional level management guidelines for hunting (e.g., hunting season length, kill limit) relies on local level information about the state of the populations of a given hunted animal. Similarly, network structure mismatches can arise when SES components vary in their temporal scale. For instance, forest growth and carbon storage can be measured over decadal scales, while recreation at the same location may be best assessed at an annual time scale.
In addition to cross-scale differences among single nodes, mismatches must also be evaluated at the network level. The benefits provided by an ES rely on interactions extended over multiple nodes, which must all overcome potential network structure mismatches to supply a given ES. Consider as an example SES components associated with the cultural ES of aesthetic value of a landscape, where the ecosystem nodes can be monitored with land-cover data measured at the watershed level, resource nodes can be monitored locally with field data on species abundances and percent cover, governance can be monitored with municipal level census, and actors perceptions of aesthetic value can be monitored with local user interviews (Johnson et al. 2019). In this case, beauty and appeal depend on the surrounding landscape, and mismatches can arise if management is not undertaken at a greater scale than the scale of ES consumption (Raudsepp-Hearne and Peterson 2016).
A special case of network structure mismatch can occur among the ES properties. All ESs can be characterized as stock (i.e., potential flow) and supply (i.e., actualized flow) and have specific measures of benefit, demand, value (i.e., biophysical, socio-cultural and monetary) and incentives (Tallis et al. 2012; Geijzendorffer et al. 2017a). While trends in these ES properties are mediated by patterns of supply and demand across the SES network, each ES property can be monitored at specific network nodes or links. The ES stock is directly monitored at the ES node (e.g., carbon stocked by a tree or a forest stand). The ES supply is monitored as the interaction among ES and actor nodes (individuals or groups), and its scale will depend on those of the interacting nodes. The ES benefit (e.g., number of people protected from flood, percentage of income supported by food production) is monitored at the actor node. The ES demand is context dependent and can either be monitored by changes in the state of actor or governance nodes. Alternatively, both ES values (e.g., market value of timber) and ES incentives (e.g., subsidies for green energy) are monitored at the governance node. The ES properties associated with an ES can occur at different scales, which may lead to scale mismatches. For example, carbon is stocked and valued locally; however, its benefits for climate mitigation are accounted for at larger scales (Duchelle et al. 2018). Additionally, mismatches within an ES property can arise from how stakeholders operating at different scales value the ES, such as mismatches in the value of recreation at the municipal and provincial levels (Hein et al. 2006).
Social and ecological boundaries are often fuzzy, such that network components may be manifest dynamically across scales of space and time, constituting a network dynamic mismatch. Beyond identifying the appropriate monitoring design for trend detection of a given ES (Larsen et al. 2001), one should also account for whether the spatial and temporal scales of network components are static or dynamic (Table 1, Fig. 2d). For example, certain ESs such as crop production and recreation can be monitored at a static spatial unit (i.e., fields and parks), while the case of fisheries and migratory hunted animals (e.g., mallard ducks) are better monitored as a dynamic spatial distribution of variable location and extent. Likewise, the temporal occurrence or availability of ESs can be static (e.g., timber) or temporally dynamic (e.g., fish stocks, crop yield, disease outbreaks). The same concepts apply to other network nodes and interactions.
Once the potential scale mismatches in the network structure, ES properties, and network dynamics have been identified, monitoring should be done at the spatial and temporal scales and frequencies that capture the identified patterns of variability inherent to the SES network. Failure to monitor the network components and ES at its proper scales can affect trend detection, but it can also affect the assessment of trade-offs in the supply of different ESs (Raudsepp-Hearne and Peterson 2016). Accounting for scale mismatches is also important for the detection of spatially synchronized increases and collapses (e.g., in fish stocks due to exploitation; Frank et al. 2016), cycles of variability (e.g., in pollination due to surrounding habitat; Kremen et al. 2004) and the emergence of risks at the landscape-level (e.g., fire-driven vegetation shifts; Henderson et al. 2016). Our multilayer network framework explicitly addresses such differences in scale among network components. Depending on the nature of the SES, the coarse layers defined by the top-tier categories (i.e., ecosystem, resource, governance, and actors) can be further decomposed to represent nodes and interactions occurring at smaller scales (e.g., individual populations or producer). Alternatively, nodes can be aggregated to represent components occurring at larger spatial scales (e.g., ecoregion, cooperative) or to represent interactions among nodes that are not anchored in space (i.e., diffuse).
3.2. The drivers of change in SES networks
The fourth framework step pertains to identifying the endogenous or exogenous drivers of ESs change, which define the long-term state of social and ecological nodes and can mediate the joint response of multiple ESs. Endogenous drivers are those already represented as components in the conceptualized SES network. For example, high ES demand might cascade to increase the frequency and magnitude of ES–actor interactions, promote gains and losses of network nodes, and have long-term consequences for ES supply. Exogenous drivers of change are external to the SES network, and may include environmental drivers such as climate change (Locatelli et al. 2013) or social drivers such as trade-offs across regions in ES valuation (Vallet et al. 2016) and global teleconnections (Cord et al. 2017b). Exogenous drivers of change are important and provide context that may explain outcomes occurring in the focal SES from place to place over time. The scales of exogenous drivers of change can be larger or smaller than that of the focal SES, and can emerge from interaction between the focal system and another multilayer network (e.g., global market forces). While endogenous drivers directly and indirectly interact with network nodes, exogenous drivers may impose a diffuse effect across the network or network layers (e.g., changes in policy) but nevertheless can still be measured at specific network components (e.g., change in ES demand and value). It is important to determine the vulnerability of the SES network to both endogenous and exogeneous drivers. Adaptive monitoring that takes a forward-looking view of system change can also support models designed to assess the vulnerability and resilience of the SES network and allow for the identification and evaluation of alternative adaptive management strategies (Laurila-Pant et al. 2019).
3.3. Connecting SES monitoring to existing standardized monitoring frameworks
The scale mismatches and drivers of change need to be reflected in the choice of variables and indicator metrics used to quantify how they change. Thus, in the fifth step of our framework we discuss the crucial need for reference baselines, repeated measurement of standardized variables across locations and scales, and robust statistical methods to detect shifts in patterns of variability along with systematic trends in ES supply over space and time (Lindenmayer and Likens 2009).
There is currently great interest in standardized monitoring frameworks for biodiversity and ESs given their importance for evaluating collective progress towards the post-2020 biodiversity goals and Sustainable Development Goals (Reyers and Selig 2020). To build upon ongoing efforts, we encourage the application of the GEO BON (Group on Earth Observations Biodiversity Observation Network) and SEEA (System of Environmental-Economic Accounting) frameworks for the monitoring of change in networked SES (GEO BON 2017; Schmeller et al. 2018). GEO BON, in conjunction with other networks in the Group on Earth Observations, stresses the value of a standardized suite of essential biodiversity, ecosystem, and social variables (Fernández et al. 2020). These essential variables can be estimated from measurements taken remotely from the air, on the ground, or from census data at specific network components (Fig. 2e), and they should be embedded in standardized workflows to make them open and interoperable among methods and users (Walters and Scholes 2017). These variables can then be compared at multiple aggregated scales consistent with the network layers, to provide regional, national, and international indicators. The same GEO BON process of standardized and interoperable workflows can be applied to a choice of variables used for ecosystem and economic accounts, designed to support the monitoring and reporting of change in natural capital (e.g., SEEA; Hein et al. 2020). Discussing which candidate indicators and proxy variables can be appropriately monitored is beyond the scope of this paper, but overviews are available (Layke et al. 2012; Tallis et al. 2012; Geijzendorffer et al. 2017a; GEO BON 2017). Nonetheless, we highlight that combining our SES network-based monitoring framework to larger integrative frameworks offered by GEO BON and SEEA will provide flows of data cognizant of complex social–ecological interactions occurring across space and time.
4. Integrative and adaptive approach for detecting and attributing change across a networked social–ecological system
Monitoring the ecological and social variables reflecting patterns of change in biodiversity and ESs is not sufficient to ensure effective interventions for sustainability (Metzger et al. 2020). First, monitoring schemes must be designed to support inference of the causal structure underlying the trends and fluctuations the SES network exhibits. Second, monitoring of the SES network needs to be adaptive, that is incorporate new information as it becomes available, whether information is developed independently of the monitoring design or by directly probing the system (Lindenmayer and Likens 2009). This approach is necessary to capture shifting trends in ES supply and demand over space and time and that may arise under novel environmental (e.g., climate regimes) and socio-economic conditions (e.g., prospective urban development). Thus, in the sixth step of our framework, we highlight how adaptive monitoring for causal inference can be achieved when monitoring is integrated with modelling and management.
The SES network provides a comprehensive and spatially explicit description of the system that serves as a blueprint of what to monitor and its spatial distribution and scales. But detecting and assessing change requires statistical and modelling methodologies to determine the causes of change at different scales, across the network’s nodes and links, within and across layers. We advocate for the use of causal inference analyses to discover and quantify the causal interdependencies in a system, that is to identify which components of a system cause others to change and by how much (Bodin et al. 2019; Ferraro et al. 2019). The network monitoring framework we emphasize here lends itself to new methods for causal network analysis that go beyond simple correlations among variables by employing time series of essential variables monitored across a SES network (Runge et al. 2019). In addition to estimating causal links, causal discovery methods can also account for spatial and temporal lags in complex systems (e.g., delayed effects of policy or climate change; Runge et al. 2019). Cause and effect relationships in SES networks are challenging to assess particularly when they rely on observational data, because they may involve many interacting variables (with direct and indirect effects) playing out over different dimensions of space and time. As presented in Ferraro et al. (2019), addressing human and ecological confounding variables will be important in identifying causal relationships and ruling out rival explanations. The advances in causal inference are promising, but given the limitations of different methods and one’s specific research objectives, simplifications about the SES can be made but their assumptions need to be clearly stated.
Through the integration of monitoring and modelling, statistically parsimonious models and competing models can be used to infer the changing strength of causality and support prediction of outcomes across the SES network (Runge et al. 2019). For instance, monitoring and modelling used to detect nonlinear feedbacks and lagged effects in a system can support the identification of early warnings (Bauch et al. 2016) or forecast the transition to alternative states (Nelson et al. 2009). Another example of a well-established method for causal inference is Bayesian belief networks (BBNs). BBNs are multivariate statistical models based on conceptualized causal webs of influence that encode conditional transition probabilities for each node state (Landuyt et al. 2013). A BBN model should be informed by the multilayer network, where the BBN nodes can depict subsets or aggregates of the SES network components (e.g., a group of beneficiaries of ES at the local scale). In turn, the BBN links convey hypothesized causal relationships between the BBN nodes and competing models can be contrasted (e.g., Alexander et al. 2020). In addition to its network properties, BBNs are capable of combining qualitative and quantitative knowledge from a diverse set of stakeholders and experts, supporting, for example, tests of alternative BBN models and its impact on predictions about the changing state of network nodes given a set of initial conditions and hypothesized causal constraints (Marcot et al. 2006). The growing accessibility to and flexibility of software for implementing Bayesian networks makes them suitable candidates for ESs monitoring and mapping across SES networks (Stritih et al. 2020). An important weakness of BBNs, however, is their limitation to handle feedback and time series. To model changes over time, others have suggested the use of dynamic BBNs where the model structure is replicated to depict feedback and time steps (Nyberg et al. 2006; Uusitalo et al. 2018). Nevertheless, dynamic BBNs often require large amounts of data for parameterization. Alternative approaches to dynamic BBNs include the addition of nodes to account for the time frame (e.g., Liedloff and Smith 2010) or representing a BBN as a difference model where each node is the difference between two time points and the links are the relationships between the differences (see section 5 for a conceptual example; Scutari et al. 2017).
Monitoring and modelling should furthermore be closely linked to management through an adaptive cycle. Adaptive monitoring schemes (Lindenmayer and Likens 2009) allow for the iterative adjustment of measurement effort, frequency, and coverage so that new information can be used to update competing causal models and decision-making (Dee et al. 2017; Lindenmayer and Likens 2009). Through the feedback between monitoring, model-based inference, and management we can iteratively learn about the outcomes we observe on the SES and that mediate the status of the ecosystems and the livelihoods of actors living within it. Altogether, it is only when we consider the interactions between ecological and social systems that monitoring, modelling, and management can truly assess the risks associated with the unintended consequences of human activities and identify management opportunities to safeguard the long-term sustainability of the SES.
5. A conceptual case study for monitoring social–ecological networks
To illustrate the networked SES monitoring framework described above and present a walkthrough of the framework steps, we conceptualize a case study based on an SES system of maple syrup provisioning in southern Québec, Canada (Fig. 1b). In the first framework step, the SES top-tier categories are identified relative to the focal ES, in this case maple syrup production. The ecological system is defined by mixed-deciduous forest stands (resource system) and sugar maple trees part of the system (resource units); the social system is defined as the Québec Maple Syrup Producers (governance system) and the maple syrup producers (actors). The mixed-deciduous forest stands interact with the sugar maple trees, which directly supply sap. The Québec Maple Syrup Producers establish the regulations by which the maple syrup producers can extract sap from the sugar maple trees to produce the ES of maple syrup. In turn, how much sap is extracted is expected to influence the sustainability of the ecological system, and cascade to impact the dynamics of supply and demand in the social system.
While the conceptual SES offers an aspatial description of the system (Fig. 3a), its monitoring requires the spatially explicit identification of the SES network configuration, achieved in the second framework step (Fig. 3b). In the maple syrup case study, land cover and field data can help identify the ecosystem nodes representing forest patches with productive maple trees, patches where sugar maple trees are absent altogether, and patches of sugar maple trees that are too young or old to be tapped for sap extraction. Nested within the ecosystem layer are the resource nodes, represented by patches of mature sugar maple trees which are themselves connected to the nested ES nodes of sap production. The state of both resource and ES nodes can be informed by field and census data. The ecological network layers are coupled to the social network layers through local interactions of sap extraction, linking ES nodes to maple syrup producer nodes. Maple syrup production arising from the ES–actor interactions can be monitored with census data and production data from the Québec Maple Syrup Producers. Alternatively, the Québec Maple Syrup Producers is a governance organization that does not necessarily have a spatially explicit definition (i.e., spatially diffuse), and can be represented as a layer.
Fig 3.

In the third framework step the potential for scale mismatches in the SES network is explicitly assessed, highlighting the scales at which ecological and social nodes are not spatially and (or) temporally aligned (i.e., mismatched). Highlighting these scale mismatches helps understand the spatial scales and temporal frequencies over which monitoring needs to be done (i.e., yearly, with producer-level resolution). In this case study, an important source of scale mismatch is a driver of change, and its explicit identification is achieved in the fourth framework step. For instance, maple syrup production has increased over time, both at the local and regional scales (Fig. 3c, data available from Statistics Canada (2017) and Fédération des producteurs acéricoles du Québec (2016, 2019). However, inter-annual climate variability and warming trends have impacted sugar maple differentially across the region (Legault et al. 2019). In particular, potential geographic shifts in climate conditions and changes in the beginning of the tap season are expected to negatively impact the production of maple syrup in southern Québec, while expanding production and the tree line northwards (Rapp et al. 2019). Thus, the impact of climate change on ecological nodes is expected to cascade through the network affecting the social system differentially across space. For example, a local decline in maple syrup production would have negative implications for the local actor nodes who currently benefit from it (e.g., reduction in income), leading to a scale mismatch. Moreover, the decline in ES supply would lead to a growth in market value. Demand from long-distance markets (national or international) would result in a telecoupling system (Liu et al. 2013) that may not only drive an increase in the exploitation of currently extracted maple resource nodes, but also lead to the exploitation of new resource nodes not previously harvested. As a consequence, spatial variation in ES supply could result in geographically variable social outcomes and inequities in market access among actors (Pascual et al. 2014). Thus, the network-based monitoring of these interdependencies would support ecological management and social governance that are attune to cross-scale trends in maple syrup production and its feedback effects across the SES.
The SES network and its drivers of change provide a blueprint of what to monitor and its spatial distribution and scale, so that on the fifth framework step appropriate variables are selected and monitored. For instance, to monitor the effects of climate change on maple syrup production the ES supply of maple syrup production (pounds/ha) can be estimated by multiplying the maple syrup yield data at the interaction between ES and actor nodes (pounds/tap, available by administrative region from (Fédération des producteurs acéricoles du Québec 2016)) with the number of maple taps at the ES nodes (available by census consolidated subdivision from Statistics Canada (2017)). Data can be aggregated to a unit relevant to monitored SES network components. For example, we mapped maple syrup production (Fig. 4a) by aggregating data to hydroshed level 10 (Lehner and Grill 2013). The effect of climate change can be monitored at the ecosystem and resource nodes, using remote sensing data on precipitation, temperature, number and duration of freeze–thaw cycles and phenology data on day of year of green up. Dynamics at the ecosystem nodes can also be monitored remotely with data on forest cover, land use, and total above ground biomass. The resource nodes can be monitored with field data on sugar maple cover, stand age, and percent contribution to community composition. The social nodes can be monitored with census and national statistics. Actor nodes can be defined by the number and location of maple syrup producers and monitored with data on production values. Additionally, the percentage of the population reliant on maple syrup production for income can provide an estimate of the ES benefit. The governance nodes can be assessed by monitoring maple syrup demand and market value. The ES interest can be monitored with economic data on investment in maple syrup companies, either at the actor or governance nodes. The ES nodes can be assessed with census data on number of maple taps, providing an estimate of ES stock. The ES–actor interactions measuring ES supply can also be assessed regionally by the number of tapped trees and annual production of maple syrup.
Fig. 4.

On the sixth and final framework step, a modelling approach such as a BBN model can be used to understand the effect of climate change on maple syrup production and the overall SES network. In this case, the nodes and links of the SES network can be aggregated and then conceptualized as a BBN (Fig. 4b) describing the hypothesized causal relationships between climatic, ecological, social, and ES variables, where nodes depict the difference between two time points (Fig. 4a). Monitoring data can provide the values needed to determine the state of BBN nodes and the strength of causality links between BBN nodes (i.e., conditional transition probabilities), and qualitative knowledge from experts can also be incorporated to describe the conditional transition probabilities between BBN node states. Bayesian inference then propagates probabilities of network states through the Bayesian network to generate quantitative outputs for alternative management scenarios for ESs. Monitoring by purely mapping and assessing deviations from historical climate baselines do provide a relevant measure of change. Yet, an understanding of the causes of change relies on the pairing of cross-scale monitoring and causal inference modelling, resulting in more robust management and decision-making (e.g., Olander et al. 2018).
6. Towards integration in social–ecological systems monitoring
Here, we conceptualized a framework to monitor ESs across an SES, mainly focusing on the provisioning of a single ES. However as discussed, landscapes supply bundles of ESs and SES dynamics often result in trade-offs between ESs (Cord et al. 2017a; Galafassi et al. 2017). Hence, in the context of ResNet, our future work will operationalize our proposed ESs monitoring network, including a list of appropriate indicator variables and analytical tools designed to address (i) one or more ES(s) within a landscape, (ii) a common ES across landscapes, and finally (iii) multiple distinct ESs across landscapes. This approach will allow us to estimate the range of variability seen across ResNet’s landscapes in terms of their varying levels of human modification and complex dynamics of ES trade-offs occurring across spatial and temporal scales (Bennett et al. 2009). For example, the ES of maple syrup production interacts with tourism, carbon storage, water quality, agriculture, forestry and peat extraction, to mention a few. Moving towards a Canada-wide monitoring network also requires accounting for how SESs differ in their structures. For instance, the SES of energy development in British Columbia and Alberta is characterized by strong trade-offs between the components of the ecological and social system due to conflicts between conservation and development. Another case in the Bay of Fundy involves budgetary constraints for Dykelands restoration and other land use outcomes. Finally, we emphasize the need to integrate knowledge sources to produce relevant monitoring that supports local and regional decisions and incorporates the range of values, priorities, and concerns of multiple stakeholders in the present and into the future (Kühl et al. 2020).
7. Conclusions
Managing for the long-term sustainability of biodiversity and ESs requires integrated strategies for adaptive monitoring. We proposed a spatially explicit multilayer and multiscale framework to guide monitoring of networked SES while accounting for the different levels and types of variability in ES states seen in and across ecosystems. When operationalized, our proposed framework has the potential to identify change in SES network structure at different scales, while accounting for mismatches in the interactions (i.e., patterns of supply and demand) among network components. Monitoring can improve causal models over time, which in turn can support forecasts and adaptive adjustments to future monitoring activities. This iterative approach will be implemented within ResNet and its performance compared among ESs embedded in SES networks found in each of ResNet’s landscapes. Our framework provides the foundations to establish the well-designed long-term monitoring programmes required to achieve the targets for biodiversity and ESs sustainability and to inform policy and governance at several scales within and across landscapes in Canada and elsewhere.
Acknowledgements
We would like to thank the editors for the opportunity to contribute to this special issue, and the reviewers for the thoughtful feedback. We acknowledge the support of the Natural Sciences and Engineering Research Council of Canada (NSERC); (funding reference number NSERC NETGP 523374-18). Cette recherche a été financée par le Conseil de recherches en sciences naturelles et en génie du Canada (CRSNG); (numéro de référence NSERC NETGP 523374-18). M-JF is thankful for a NSERC of Canada Discovery Grant (#5134) and the NSERC Canada Research Chair (CRC). AG acknowledges the support of the Liber Ero Chair in Biodiversity Conservation, and the NSERC Discovery program.
References
Alexander SM, Staniczenko PPA, and Bodin Ö. 2020. Social ties explain catch portfolios of small-scale fishers in the Caribbean. Fish and Fisheries, 21: 120–131.
Bagstad KJ, Johnson GW, Voigt B, and Villa F. 2013. Spatial dynamics of ecosystem service flows: a comprehensive approach to quantifying actual services. Ecosystem Services, 4: 117–125.
Barnes ML, Bodin Ö, McClanahan TR, Kittinger JN, Hoey AS, Gaoue OG, et al. 2019. Social-ecological alignment and ecological conditions in coral reefs. Nature Communications, 10.
Bauch CT, Sigdel R, Pharaon J, and Anand M. 2016. Early warning signals of regime shifts in coupled human-environment systems. Proceedings of the National Academy of Sciences of the United States of America, 113: 14560–14567.
Bennett EM, Peterson GD, and Gordon LJ. 2009. Understanding relationships among multiple ecosystem services. Ecology Letters, 12: 1394–1404.
Bodin Ö. 2017. Collaborative environmental governance: Achieving collective action in social-ecological systems. Science, 357.
Bodin Ö, and Tengö M. 2012. Disentangling intangible social-ecological systems. Global Environmental Change, 22: 430–439.
Bodin Ö, Alexander SM, Baggio J, Barnes ML, Berardo R, Cumming GS, et al. 2019. Improving network approaches to the study of complex social–ecological interdependencies. Nature Sustainability, 2: 551–559.
Cardinale BJ, Matulich KL, Hooper DU, Byrnes JE, Duffy E, Gamfeldt L, et al. 2011. The functional role of producer diversity in ecosystems. American Journal of Botany, 98: 572–592.
Ceballos G, Ehrlich PR, Barnosky AD, García A, Pringle RM, and Palmer TM. 2015. Accelerated modern human-induced species losses: entering the sixth mass extinction. Science Advances, 1: 9–13.
Chan KM, Gould RK, and Pascual U. 2018. Editorial overview: Relational values: what are they, and what’s the fuss about?. Current Opinion in Environmental Sustainability, 35: A1–A7.
Cord AF, Bartkowski B, Beckmann M, Dittrich A, Hermans-Neumann K, Kaim A, et al. 2017a. Towards systematic analyses of ecosystem service trade-offs and synergies: main concepts, methods and the road ahead. Ecosystem Services, 28: 264–272.
Cord AF, Brauman KA, Chaplin-Kramer R, Huth A, Ziv G, and Seppelt R. 2017b. Priorities to advance monitoring of ecosystem services using earth observation. Trends in Ecology and Evolution, 32: 416–428.
Dee LE, Allesina S, Bonn A, Eklöf A, Gaines SD, Hines J, et al. 2017. Operationalizing network theory for ecosystem service assessments. Trends in Ecology and Evolution, 32: 118–130.
Díaz S, Settele J, Brondízio ES, Ngo HT, Agard J, Arneth A, et al. 2019. Pervasive human-driven decline of life on Earth points to the need for transformative change. Science, 366: eaax3100.
Duchelle AE, Simonet G, Sunderlin WD, and Wunder S. 2018. What is REDD+ achieving on the ground? Current Opinion in Environmental Sustainability, 32: 134–140.
Duncan C, Thompson JR, and Pettorelli N. 2015. The quest for a mechanistic understanding of biodiversity–ecosystem services relationships. Proceedings of the Royal Society B: Biological Sciences, 282: 20151348.
Fédération des producteurs acéricoles du Québec. 2016. Statistiques Acéricoles 2016.
Fédération des producteurs acéricoles du Québec. 2019. 30 ans déjà plan conjoint: Statistiques acéricoles.
Fernández N, Ferrier S, Navarro LM, and Pereira HM. 2020. Essential biodiversity variables: integrating in-situ observations and remote sensing through modeling, In J. Cavender-Bares and JA Gamon, and PA Townsend. Remote Sensing of Plant Biodiversity. Springer Nature, pp. 1–581.
Ferraro PJ, Sanchirico JN, and Smith MD. 2019. Causal inference in coupled human and natural systems. Proceedings of the National Academy of Sciences of the United States of America, 116: 5311–5318.
Frank KT, Petrie B, Leggett WC, and Boyce DG. 2016. Large scale, synchronous variability of marine fish populations driven by commercial exploitation. Proceedings of the National Academy of Sciences of the United States of America, 113: 8248–8253.
Galafassi D, Daw TM, Munyi L, Brown K, and Barnaud C. 2017. Learning about social-ecological trade-offs. Ecology and Society, 22: 2.
Geijzendorffer IR, Cohen-Shacham E, Cord AF, Cramer W, Guerra C, and Martín-López B. 2017a. Ecosystem services in global sustainability policies. Environmental Science and Policy, 74: 40–48.
Geijzendorffer IR, and Roche PK. 2013. Can biodiversity monitoring schemes provide indicators for ecosystem services? Ecological Indicators, 33: 148–157.
Geijzendorffer IR, van Teeffelen AJA, Allison H, Braun D, Horgan K, Iturrate-Garcia M, et al. 2017b. How can global conventions for biodiversity and ecosystem services guide local conservation actions? Current Opinion in Environmental Sustainability, 29: 145–150.
GEO BON. 2017. GEO BON Implementation Plan 2017-2020. Leipzig.
González-García A, Palomo I, González JA, López CA, and Montes C. 2020. Quantifying spatial supply-demand mismatches in ecosystem services provides insights for land-use planning. Land Use Policy, 94: 104493.
Gonzalez A, Thompson PL, and Loreau M. 2017. Spatial ecological networks: planning for sustainability in the long-term. Current Opinion in Environmental Sustainability, 29: 187–197.
Guerrero AM, Mcallister RRJ, and Wilson KA. 2015. Achieving cross-scale collaboration for large-scale conservation initiatives. Conservation Letters, 8: 107–117.
Hein L, Bagstad KJ, Obst C, Edens B, Schenau S, Castillo G, et al. 2020. Progress in natural capital accounting for ecosystems. Science, 367: 514–515.
Hein L, van Koppen K, de Groot RS, and van Ierland EC. 2006. Spatial scales, stakeholders and the valuation of ecosystem services. Ecological Economics, 57: 209–228.
Henderson KA, Bauch CT, and Anand M. 2016. Alternative stable states and the sustainability of forests, grasslands, and agriculture. Proceedings of the National Academy of Sciences of the United States of America, 113: 14552–14559.
IPBES. 2019. Summary for policymakers of the global assessment report on biodiversity and ecosystem services of the Intergovernmental Science-Policy Platform on Biodiversity and Ecosystem Services. IPBES secretariat, Bonn, Germany.
Johnson ML, Campbell LK, Svendsen ES, and McMillen HL. 2019. Mapping urban park cultural ecosystem services: a comparison of twitter and semi-structured interview methods. Sustainability (Switzerland), 11.
Keyes AA, Mclaughlin JP, Barner AK, and Dee LE. 2021. An ecological network approach to predict ecosystem service vulnerability to species losses. Nature Communications, 12: 1586.
Kluger LC, Gorris P, Kochalski S, Mueller MS, and Romagnoni G. 2020. Studying human-nature relationships through a network lens: a systematic review. People and Nature, 00: 1–17.
Kremen C, Williams NM, Bugg RL, Fay JP, and Thorp RW. 2004. The area requirements of an ecosystem service: crop pollination by native bee communities in California. Ecology Letters, 7: 1109–1119.
Kühl HS, Bowler DE, Bösch L, Bruelheide H, Dauber J, Eichenberg D, et al. 2020. Effective biodiversity monitoring needs a culture of integration. One Earth, 3: 462–474.
Landuyt D, Broekx S, D’hondt R, Engelen G, Aertsens J, and Goethals PLM. 2013. A review of Bayesian belief networks in ecosystem service modelling. Environmental Modelling and Software, 46: 1–11.
Larsen DP, Kincaid TM, Jacobs SE, and Urquhart NS. 2001. Designs for evaluating local and regional scale trends. BioScience, 51: 1069–1078.
Laurila-Pant M, Mäntyniemi S, Venesjärvi R, and Lehikoinen A. 2019. Incorporating stakeholders’ values into environmental decision support: a Bayesian Belief Network approach. Science of the Total Environment, 697: 134026.
Layke C, Mapendembe A, Brown C, Walpole M, and Winn J. 2012. Indicators from the global and sub-global Millennium Ecosystem Assessments: an analysis and next steps. Ecological Indicators, 17: 77–87.
Legault S, Houle D, Plouffe A, Ameztegui A, Kuehn D, Chase L, et al. 2019. Perceptions of U.S. and Canadian maple syrup producers toward climate change, its impacts, and potential adaptation measures. PLoS ONE, 14: 1–27.
Lehner B, and Grill G. 2013. Global river hydrography and network routing: baseline data and new approaches to study the world’s large river systems. Hydrological Processes, 27: 2171–2186.
Liedloff AC, and Smith CS. 2010. Predicting a “tree change” in Australia’s tropical savannas: Combining different types of models to understand complex ecosystem behaviour. Ecological Modelling, 221: 2565–2575.
Lindborg R, Gordon LJ, Malinga R, Bengtsson J, Peterson G, Bommarco R, et al. 2017. How spatial scale shapes the generation and management of multiple ecosystem services. Ecosphere, 8: e01741.
Lindenmayer DB, and Likens GE. 2009. Adaptive monitoring: a new paradigm for long-term research and monitoring. Trends in Ecology and Evolution, 24: 482–486.
Liu J, Hull V, Batistella M, DeFries R, Dietz T, Fu F, et al. 2013. Framing sustainability in a telecoupled world. Ecology and Society, 18: 26.
Locatelli B, Imbach P, and Wunder S. 2013. Synergies and trade-offs between ecosystem services in Costa Rica. Environmental Conservation, 41: 27–36.
MA. 2005. Ecosystems and human well-being. Synthesis. Island Press, Washington, DC.
Marcot BG, Steventon JD, Sutherland GD, and McCann RK. 2006. Guidelines for developing and updating Bayesian belief networks applied to ecological modeling and conservation. Canadian Journal of Forest Research, 36: 3063–3074.
Martín-López B, Gómez-Baggethun E, García-Llorente M, and Montes C. 2014. Trade-offs across value-domains in ecosystem services assessment. Ecological Indicators, 37: 220–228.
McCann KS, Cazelles K, MacDougall AS, Fussmann GF, Bieg C, Cristescu M, et al. 2020. Landscape modification and nutrient-driven instability at a distance. Ecology Letters, 24: 398–414.
McGinnis MD, and Ostrom E. 2014. Social-ecological system framework: initial changes and continuing challenges. Ecology and Society, 19: 30.
Metzger JP, Fidelman P, Sattler C, Schroter B, Maron M, Eigenbrod F, et al. 2020. Connecting governance interventions to ecosystem services provision: a social-ecological network approach. People and Nature, 00: 1–15.
Minnes S, Gaspard V, Loring PA, Baulch H, and Breen S. 2020. Transforming conflict over natural resources : a socio-ecological systems analysis of agricultural drainage. Facets, 5: 864–886.
Nelson E, Mendoza G, Regetz J, Polasky S, Tallis H, and Cameron DR. et al. 2009. Modeling multiple ecosystem services, biodiversity conservation, commodity production, and tradeoffs at landscape scales. Frontiers in Ecology and the Environment, 7: 4–11.
Nyberg JB, Marcot BG, and Sulyma R. 2006. Using Bayesian belief networks in adaptive management. Canadian Journal of Forest Research 36: 3104–3116.
Olander LP, Johnston RJ, Tallis H, Kagan J, Maguire LA, Polasky S, et al. 2018. Benefit relevant indicators: ecosystem services measures that link ecological and social outcomes. Ecological Indicators, 85: 1262–1272.
Ostrom E. 2007. A diagnostic approach for going beyond panaceas. PNAS, 104: 15181–15187.
Pascual U, Phelps J, Garmendia E, Brown K, Corbera E, Martin A, et al. 2014. Social equity matters in payments for ecosystem services. BioScience, 64: 1027–1036.
Rapp JM, Lutz DA, Huish RD, Dufour B, Ahmed S, Morelli TL, et al. 2019. Finding the sweet spot: shifting optimal climate for maple syrup production in North America. Forest Ecology and Management 448: 187–197.
Raudsepp-Hearne C, and Peterson GD. 2016. Scale and ecosystem services: how do observation, management, and analysis shift with scale - lessons from Québec. Ecology and Society, 21: 16.
Reyers B, Selig ER. 2020. Global targets that reveal the social–ecological interdependencies of sustainable development. Nature Ecology and Evolution, 4: 1011–1019.
Runge J, Bathiany S, Bollt E, Camps-Valls G, Coumou D, Deyle E, et al. 2019. Inferring causation from time series in Earth system sciences. Nature Communications, 10: 1–13.
Sayles JS, and Baggio JA. 2017. Social-ecological network analysis of scale mismatches in estuary watershed restoration. Proceedings of the National Academy of Sciences of the United States of America, 114: E1776–E1785.
Scheffer M, Bascompte J, Brock WA, Brovkin V, Carpenter SR, Dakos V, et al. 2009. Early-warning signals for critical transitions. Nature, 461: 53–59.
Schmeller DS, Weatherdon L V, Loyau A, Bondeau A, Brotons L, Brummitt N, et al. 2018. A suite of essential biodiversity variables for detecting critical biodiversity change. Biological Reviews, 93: 55–71.
Scutari M, Auconi P, Caldarelli G, and Franchi L. 2017. Bayesian Networks Analysis of Malocclusion Data. Scientific Reports, 7: 1–11.
Statistics Canada, 2017. Table 32-10-0423-01 Maple taps [online]: Available from www150.statcan.gc.ca/t1/tbl1/en/tv.action?pid=3210042301.
Stritih A, Rabe SE, Robaina O, Grêt-Regamey A, and Celio E. 2020. An online platform for spatial and iterative modelling with Bayesian Networks. Environmental Modelling and Software, 127.
Sun W, Li D, Wang X, Li R, Li K, and Xie Y. 2019. Exploring the scale effects, trade-offs and driving forces of the mismatch of ecosystem services. Ecological Indicators, 103: 617–629.
Tallis H, Mooney H, Andelman S, Balvanera P, Cramer W, Karp D, et al. 2012. A global system for monitoring ecosystem service change. BioScience, 62: 977–986.
Uusitalo L, Tomczak MT, Müller-Karulis B, Putnis I, Trifonova N, and Tucker A. 2018. Hidden variables in a Dynamic Bayesian Network identify ecosystem level change. Ecological Informatics, 45: 9–15.
Vallet A, Locatelli B, Levrel H, Pérez CB, Imbach P, Carmona NE, et al. 2016. Dynamics of ecosystem services during forest transitions in Reventazón, Costa Rica. PLoS ONE, 11: e0158615.
Walters M, and Scholes RJ. 2017. The GEO handbook on biodiversity observation networks. Springer Open.
Winkler KJ, Dade MC, and Rieb JT. 2021. Mismatches in the ecosystem services literature — A review of spatial, temporal, and functional-conceptual mismatches. Current Landscape Ecology Reports, 6: 23–34.
Yoccoz NG, Nichols JD, and Boulinier T. 2001. Monitoring of biological diversity in space and time. Trends in Ecology and Evolution, 16: 446–453.
Information & Authors
Information
Published In
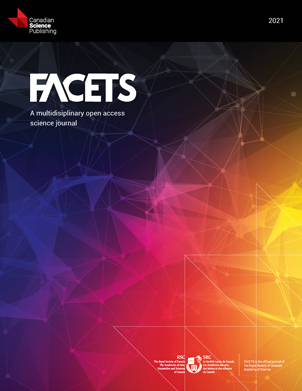
FACETS
Volume 6 • Number 1 • January 2021
Pages: 1670 - 1692
Editor: Elena M. Bennett
History
Received: 21 December 2020
Accepted: 26 June 2021
Version of record online: 7 October 2021
Notes
This paper is part of a Collection titled “Food, Fiber, Fuel, and Function: Pathways to manage ecosystem services in Canada’s working landscapes”.
Copyright
© 2021 Firkowski et al. This work is licensed under a Creative Commons Attribution 4.0 International License (CC BY 4.0), which permits unrestricted use, distribution, and reproduction in any medium, provided the original author(s) and source are credited.
Data Availability Statement
All relevant data are within the paper.
Key Words
Sections
Subjects
Authors
Author Contributions
CRF, M-JF, and AG conceived and designed the study.
All contributed resources.
All drafted or revised the manuscript.
CRF produced all the figures.
AMS generated the maps presented in the manuscript.
Competing Interests
The authors have declared that no competing interests exist.
Metrics & Citations
Metrics
Other Metrics
Citations
Cite As
Carina Rauen Firkowski, Amanda M. Schwantes, Marie-Josée Fortin, and Andrew Gonzalez. 2021. Monitoring social–ecological networks for biodiversity and ecosystem services in human-dominated landscapes. FACETS.
6: 1670-1692.
https://doi.org/10.1139/facets-2020-0114
Export Citations
If you have the appropriate software installed, you can download article citation data to the citation manager of your choice. Simply select your manager software from the list below and click Download.
Cited by
1. Monitor social–ecological systems to achieve global goals for biodiversity and nature's contributions to people
2. Monitoring ecosystem services with essential ecosystem service variables
3. Dynamics in the landscape ecology of institutions: lags, legacies, and feedbacks drive path-dependency of forest landscapes in British Columbia, Canada 1858–2020
4. A framework for the detection and attribution of biodiversity change
5. Analysis and Evaluation of Ecological Environment Monitoring Based on PIE Remote Sensing Image Processing Software
6. Ecosystem Services and Sustainability: A Framework for Improving Decision-Making in Urban Areas
7. Conceptualizing ecosystem services using social–ecological networks
8. Facing the challenges of using place-based social-ecological research to support ecosystem service governance at multiple scales
9. Managing Canada’s land- and seascapes for multiple ecosystem services in the Anthropocene: introduction to the Food, Fiber, Fuel, and Function collection