Next steps for ecosystem service models: integrating complex interactions and beneficiaries
Abstract
Accounting for ecosystem services (ES)—the ways in which society and people directly benefit from ecological processes and functions—is crucial for developing sustainable landscape management approaches that consider the interrelationship between people and nature. Previous research has produced models that estimate the provision of potential ES by landscapes to help inform policy and stakeholder decision-making. However, most modelling efforts do not consider the delivery of ES to specific human populations or communities, making it difficult to evaluate any possible human welfare implications from alternative land use planning scenarios. In this paper, we first explore the recent state of science of ES modelling from the perspective of ES provision and delivery to the people that benefit from them. Second, we propose the addition of some essential aspects of complexity using the classic social–ecological system framework, crucial for developing models to inform pragmatic decision-making. Our propositions are illustrated using simplified examples inspired by sea otter conservation in the seascapes of British Columbia. Integrating these concepts in future ES models should serve as a baseline for future management approaches that more adequately capture the important implications of landscape scenarios on human well-being.
1. Introduction
Sustainable management of tomorrow’s ecosystems relies on understanding how change, such as climate or land use changes, can affect ecosystem services (ES) provisioning and flow. ES are defined as benefits that humans acquire directly or indirectly from ecosystems (Kremen 2005; Díaz et al. 2015). These services can be classified into four main categories: supporting, provisioning, regulating, and cultural services. The global value of ES was estimated at US$125 trillion per year in 2011 (Costanza et al. 2014). New economic indicators, such as the gross ecosystem product (Ouyang et al. 2020) have emerged to better integrate the valuation of natural systems and their benefits for future planning. The models used to estimate the benefits of ES to people, however, lack many important real-world spatial dynamics and interactions. Landscapes are complex systems composed of a multitude of entities, processes, and interactions across scales of space and time. The network of ES emerging from these systems share the same degree of complexity in structure and behavior. However, ES are often considered individually in models, with little to no interaction among them (Ashagre et al. 2018; Cunningham et al. 2018; Syrbe et al. 2018). At the same time, humans are generally concentrated in urban population clusters considered disjointed from natural systems that, particularly in this era of globalization, lead to spatial mismatches between ES supply and demand (Syrbe and Grunewald 2017; Lindegren et al. 2018). Thus, models that fail to capture ES interactions on the biophysical side and how services flow from the landscape to benefit communities and society on the social side have limited utility for planning and decision-making. In their study, Rieb et al. (2017) identified three key points to improve ES modelling: (i) understanding complex dynamics of ES in space and time, (ii) linking ES provision to human well-being, and (iii) determining the potential technology to substitute for or enhance ES.
Understanding individual ES has been extensively documented in the literature. Comprehensive studies describing the social–ecological processes behind the provisioning of ES have informed models quantifying supply of a vast suite of services such as food provisioning (Li et al. 2019; Grafius et al. 2020), pollination (Lonsdorf et al. 2009; Montoya et al. 2019), flood prevention (Kourgialas and Karatzas 2011; Vojtek and Vojteková 2016), water quality (Su et al. 2008; Chu et al. 2018), and recreation (Byczek et al. 2018; Havinga et al. 2020). These models highlight key processes that can be actively targeted to increase or decrease the supply of a given ES. Nevertheless, leveraging these processes can cause fundamental changes in the functioning of ecosystems through tradeoffs between different services (Rodríguez et al. 2006; Bennett and Balvanera 2007). Some studies aim to understand how landscape changes can jointly impact a suite of ES, notably through the use of “ecosystem bundles” (Raudsepp-Hearne et al. 2010a) and network approaches (Field and Parrott 2017), but few have tackled the complexity of interactions that can exist between services such as feedback effects, thresholds, and time lags. This may lead to a selection of human-induced changes in systems that might have undetected deleterious effects on other important services not central to the problem.
In addition to the lack of interactions in ES modelling, most of these studies have exclusively focused on the biophysical supply of services, mapping them as resources available in given systems (Martínez-Harms and Balvanera 2012). For services to be utilized, supply of services and the demand and utilization by people must be connected by the flow of services to the human populations that benefit from them (Bagstad et al. 2013a). Some studies estimate utilization of ES by overlapping maps of supply and demand. Nevertheless, capturing actual flows between supplying regions and human use is often lacking. This can be challenging because of teleconnections (Liu et al. 2013) through which services move around and among landscapes, even globally, in particular for provisioning services such as food or timber where production and utilization can be separated by thousands of miles.
In this paper, we first present a brief state-of-the-science review on ES modelling, focusing on how models currently deal with ecological and social complexity. Within ecological complexity, we mainly assess how models consider interactions between multiple ES simultaneously. Investigating social–ecological complexity could take many directions, including better understanding human decision-making, governance systems, or policies that protect or provide ES. To keep our scope manageable and focus on what we think are feasibly attainable first steps toward improving ES, we focus our review of social complexity on identifying beneficiaries to model flows and quantifying those flows to beneficiary groups. We end with a simplified case study to both illustrate the risks of not considering complexity and help inform how to move forward to better integrate these notions of complexity.
2. State of science
2.1. Modelling multiple ES
A common approach for modelling multiple ES in a single study has been to consider them as parallel noninteracting processes (Schulp et al. 2012; Schirpke et al. 2013). In this approach, each ES is modelled and mapped individually, allowing identification of areas of overlap where multiple services are provided. This is common at large spatial scales, such as national assessments of ES with examples applied to Germany (Grunewald et al. 2016), the United Kingdom (Bateman et al. 2013), and Israel (Lotan et al. 2018). While these studies offer in-depth assessments of the ecological health of systems and identify which services are available where, not considering interactions between services make predicting responses to changes difficult.
Some studies have started looking at spatial interactions between ES using ES bundles. ES bundles are defined as repeatable positive (synergies) and negative (trade-offs) in ES associations in space and time (Raudsepp-Hearne et al. 2010b). While this approach does not focus on mechanistic dynamics that lead to tradeoffs, it allows detection of spatial associations and disassociations between ES. Usually, ES bundles rely on land use and land cover data (LULC) by associating bundles of services to landscape classes (Queiroz et al. 2015; Baró et al. 2017). While this approach works well at large spatial scales where resolution is low, it presents strong limitations when working at the local scale, where diversity of management and processes within LULC types becomes one of the main drivers of ES bundle diversity. Vannier et al. (2019) proposed a social–ecological conceptual framework, integrating stakeholder and expert knowledge, to analyze ES bundles at small spatial scales. They used high-resolution data in combination with stakeholder-defined ES interests to breakdown LULC categories into relevant subclasses to map bundles across their landscape. While not directly integrating complex interactions between ES in the modelling process, such studies have highlighted the interconnected nature of ES, identifying trade-offs and synergies among services (Turner et al. 2014; Yang et al. 2015). Spake et al. (2017) presented a detailed evaluation of current methods used in ES bundle modelling. The use of ES bundles is a major first step in the integration of complexity in ES models.
Modelling multiple ES has been facilitated by the emergence of simulation platforms offering pre-existing intuitive models to simulate a wide suite of services. These spatially explicit tools allow users to model ES supply at a wide variety of scales. Bagstad et al. (2013b) reviewed 17 decision-support tools for ES quantification and valuation, each evaluated for several key criteria such as time requirements, capacity for independent application, scalability, and generalizability. One of the most popular platforms is InVEST (Integrated Valuation of Ecosystem Services and Tradeoffs, Sharp et al. 2015) developed by Stanford’s Natural Capital Project team. InVEST offers a suite of models to map a wide variety of ES such as crop pollination (Groff et al. 2016), carbon storage (Pechanec et al. 2018), and water yield (Redhead et al. 2016). InVEST relies on geographic information sources and produces maps as outputs to help inform decision-making. While users can model as many as 18+ ES in InVEST, these are considered as independent noninteracting entities and do not account for complex interactions. Another promising tool for modelling multiple ES is ARIES (Artificial Intelligence for Ecosystem Services, Villa et al. 2014). ARIES relies on common semantics that allow users to upload, share, or utilize others’ models and data, effectively reducing the time necessary for new users to be able to run models. ARIES uses artificial intelligence to identify suitable models and data sets available within its system to identify appropriate ES models in any given location or context (Martínez-López et al. 2019). However, ARIES is still limited in the number of ecosystem models already available compared with InVEST. A detailed early comparison between InVEST and ARIES using a case study can be found in Bagstad et al. (2013c), which highlights that while model outcomes between InVEST and ARIES can differ, the direction of change is typically in agreement between the two approaches. The time to develop models in both platforms was roughly equivalent, the time allocated to parameterize, run, and test the models was faster in ARIES.
Several studies have started pushing for integrating more complex interactions in ES modelling to produce more robust models for decision-support. Recently, Field and Parrott (2017) proposed a spatial network theory approach to highlight flow between ES at the local level. By building a network with links between spatial nodes of ES provisioning, they can represent the flow of ES within a landscape and the inter-connections between ES. This approach is a first step towards modelling the interactions among multiple ES on a landscape and permits the identification of spatial locations that may be important because of their role in facilitating connectivity of the network.
A similar approach, more social–ecologically oriented, is the Petri net approach (Rova et al. 2019). Petri nets are graphical and mathematical modelling tools that consist of two kinds of nodes: places and transitions. Places represent conditions, resources, or items (ES, resource systems, governance systems), while transitions represent actions or events (activities, processes, ecosystem functions). Although this model presents promising capabilities for exploring the potential impacts of general scenarios such as climate change on multiple ES within a social–ecological system, the lack of spatial inputs makes it difficult to inform precise spatial decision-making usually considered at smaller scales by stakeholders.
More generally, a wide variety of approaches exist for modelling the actual processes involved in ES and their interactions. Bayesian belief networks are probabilistic graphical models representing random variables as nodes with links connecting them (McVittie et al. 2015; Gonzalez-Redin et al. 2016). Although this probabilistic approach can account for uncertainty that can be overwhelming when modelling complex systems, interactions between ES are only represented as unilateral links and thus cannot integrate feedback effects. Causal loop diagrams and stock-flow models, which are part of wider group of models called system dynamics models, can also be used to model interactions (Robinson et al. 2013; Coletta et al. 2021). These approaches build representations of a system through variables that interact with each other in positive or negative ways. Modellers can integrate feedbacks by creating loops between variables. Still, it can be difficult to integrate nonlinear patterns such as threshold effects or nonlinear relationships more broadly.
2.2. Modelling ES flow from supply to beneficiaries
While most studies focus on mapping and modelling the supply of ES, where and how services are used, consumed, or enjoyed by human populations is often not addressed. How people benefit from an ES is represented most often either as realized use or consumption of an ES, or by economic demand for the service. In general, economic demand is not readily observable to the researcher with simple administrative or observational data. Aggregate economic demand is defined as the quantity of a particular good (service) desired at a particular price. The higher the price, typically the lower the quantity demanded; the lower the price the higher the quantity demanded. This results in a typical downward-sloping demand curve for normal goods. For most ES, we often only observe some aggregate quantity that is consumed at a prevailing “price”, but rarely how consumption or use changes with a (substantial) change in price. Thus, demand in a strict sense is often difficult to observe. A concept closely linked to demand and use is ES flow, which describes how an ES that comes from a landscape (the supply) is captured or flows to recipient beneficiaries. In practice, empirical studies rarely cast their measurement as flow, but functionally this would most likely be similar if not indistinguishable from use or consumption. Therefore, we focus on distinctions between economic demand and use/consumption.
In some cases, specific economic valuation studies are carried out to estimate demand either through travel cost or willingness-to-pay studies. These typically combine individual survey data into an aggregated level of demand. These estimates can be used for valuing changes in ES supply, usually to estimate the economic impacts of land use and land cover changes, for example, on increases in water quality (e.g., Polasky et al. 2011). Castro et al. (2014) focused on a suite of ES (crop production, climate regulation, water flow maintenance, control of erosion and maintenance of habitats) in eastern Andalusia in Spain, aggregating demand at the local level. While aggregation allows easier valuation of changes, such an approach negates the possibility to integrate variance, and thus fine-scale spatial relationships between supply and demand.
From the travel cost literature, aggregate demand has also been estimated from publicly available social media databases to estimate visitation rates of natural areas. For example, Keeler et al. (2015) used data from Flickr, an online photo-sharing platform, to estimate visitations of Minnesota and Iowa lakes, and the travel costs for individual visitors were used to estimate demand for lake-based recreation in this region.
Aggregate demand has limitations, however, especially when the ES of concern are place-based and do not move. Further, disaggregating impacts of ES on populations has become of interest specifically for understanding how different segments of society depend and rely upon various ES, especially for vulnerable populations (Daw et al. 2011; Howe et al. 2012; Mace et al. 2012; Robinson et al. 2019). Several recent studies have spatially disaggregated demand primarily by using land cover maps. For example, Schulp et al. (2014) applied such an approach to estimate pollination services across Europe where demand was modelled using crop cover maps with each crop having its own demand characteristics. Tao et al. (2018) quantified ES demand for different land cover classes through an expert-based matrix model using comparable semi-quantitative units, as first proposed by Burkhard et al. (2012).
Others represent demand spatially with population distribution maps and assuming demand is homogeneous across the population. For example, Baró et al. (2016) examined the relationship between air quality and outdoor recreation demand in the Barcelona metropolitan region. Per-capita demand is assumed constant, and population density maps are then compared to ES supply maps. Others disaggregate demand across populations. For example, Ma et al. (2019) considered demand at the human settlement level, estimating the spatial–temporal variance of supply and demand of water security across the Miyun reservoir near Beijing, China. Including a temporal scale in this study allowed identification of areas that are more (or less) vulnerable to ES changes. By disaggregating demand at fine spatial scales, ES supply and demand can be overlapped to identify which areas have a positive or negative balance regarding specific ES (Wang et al. 2019). Finally, demand has also been modelled at the household level, which then allows for separating ES use and dependency into different groups of beneficiaries. Robinson et al. (2019) conducted a household-level assessment with detailed household-level survey data, analyzing how various beneficiary groups depend on ES for their well-being. Such a fine-scale approach allows one to not only identify vulnerable households but also anticipate how policy decisions would potentially impact each group of beneficiaries.
ES bundles have also been used to simultaneously analyze supply and demand across multiple services (García-Nieto et al. 2013; Baró et al. 2017; Zoderer et al. 2019). These studies map ES supply and demand to analyze the linkages among multiple ES to classify spatial units into ES bundles. Schirpke et al. (2019) used an ES bundle approach, integrating supply, demand, and flows at a municipality level to identify five different ES bundles. They then used a random forest analysis to explain ES bundle distribution and found that 12 socio-ecological variables explained 81% of the municipalities to the ES bundles. Identifying bundles that integrate flow and demand, and thus highlight vulnerable areas, could facilitate policymaking to target specific services and areas.
Human demand and interactions with the ecological system have also been modelled using agent-based models (Bagstad et al. 2013a). Humans are often represented as either groups or individuals that can influence the system either by directly interacting with resources or making decisions that will influence the modelled ecological processes. These approaches have been applied to model a wide variety of ES such as tourism (Balbi et al. 2013), forest timber (Habib et al. 2016), and livestock (Yan et al. 2019). These models allow exploration of the potential consequences of management scenarios on both supply and demand of ES.
Some recent modelling frameworks have emerged with the goal of linking ES supply to beneficiaries. Chaplin-Kramer et al. (2019) proposed a conceptual framework for calculating nature’s contribution to people. By modelling demand using population distributions in relation to ecosystem supply models, their framework identifies areas where benefits are realized (supply meets demand), realized with a gap (demand exceeds supply), and unrealized (absence of supply). This framework was applied at the global scale and highlights the importance of considering demand to identify management scenarios. Johnson et al. (2019) developed a modelling toolkit, “Mapping Ecosystem Services to Human well-being” (MESH) that integrates models from InVEST to estimate the tradeoffs and synergies between five ES and 10 associated sustainable development goals (United Nations 2015). These goals, developed by the United Nations, aim at achieving a better and more sustainable future by addressing global challenges such as poverty, inequality, climate change, and environmental degradation. In the context of ES, this can only be done by modelling flow of ES to beneficiaries (Bagstad et al. 2014).
3. Moving towards increased complexity in ES decision support
A common framework used to describe human–nature interactions in ecosystems is the social–ecological system (SES) framework developed by Elinor Ostrom and colleagues (Ostrom 2009; McGinnis and Ostrom 2014) (Fig. 1). The SES framework consists of four main components and identifies critical relationships among these components. Its generalized structure allows for application to a wide variety of SES and helps homogenize the description of processes and entities across systems. In this framework, the ecological system is broken down into two core components: “resource systems”, which themselves are comprised of a set of “resource units”. The social system consists of “governance systems”, which frames the institutional context (the rules and conditions of the system), and “actors” who participate in the system. Finally, action situations occur at the interface between both systems, representing the choices actors make. Since its conceptualization, this framework has become a keystone representation of SES across the world (see review by Partelow (2018)).
Fig. 1.

Since it was proposed, the SES framework has been subject to debate and propositions for improvement. Thiel et al. (2015) conducted a review of 20 publications using the SES framework and highlighted a lack of consistency of use among these, with the SES framework appearing to be less flexible in fields where it can be usefully applied. The actor-centric vision of the SES framework has also led to discussions about the necessity to better integrate ecological processes within the framework. Epstein et al. (2013) proposed the addition of another core sub-system, ecological rules, to explicitly incorporate knowledge from the natural sciences.
Despite these limitations, the SES framework continues to be widely used as it is one of the simplest frameworks available that effectively represents the key components of a SES focused on natural resource use and governance. We thus use this as a starting point and build upon it to discuss, through a case study, how considering additional system interactions and complexity can help avoid poor decision-making regarding ES management. We also use the SES framework to highlight places where additional details or added complexity may help better conceptualize future models. To demonstrate the possible improvements in modelling outcomes, we draw on a simplified and hypothetical case study inspired by highly complex coastal SES in British Columbia (Watson and Estes 2011; Ling et al. 2015; Lee et al. 2016; Burt et al. 2020). This example centers on the consequences of sea otter (Enhydra lutris) recovery, historically extirpated in the region through hunting, and whose reintroduction and conservation has had a wide variety of impacts on local ES.
3.1. Integrating ES interactions
As illustrated in our review, most ES models that consider interactions do so as either simple spatial relationships (ES bundles) or mechanistic approaches that fail to capture complex interactions such as threshold effects or nonlinear relationships. While simplifying interactions into simple positive or negative relationships facilitates straightforward assessments, overlooking these complex dynamics can lead to problematic decision-making.
In our case study, sea otter populations are known to have clear direct effects to some ES (Fig. 2a) For example, sea otters feed on shellfish and have an overall negative impact on shellfish populations, consequently impacting commercial shellfish fisheries dependent on those resources (Carswell et al. 2015). However, through consumption of sea urchins, otters can help kelp forest recover, thus increasing carbon sequestration (Ling et al. 2015). When left unchecked, sea urchin populations can take over kelp forests and create sea urchin “barrens”—places where nothing else grows (Filbee-Dexter and Scheibling 2014). On the SES framework, these interactions would be represented by direct and indirect interactions between resource units. This simple linear approach to modelling ES would lead to simple decision-support outcomes such as increasing carbon sequestration then protecting sea otter populations or increasing shellfish production then letting sea otter populations decline.
Fig. 2.

However, the relationships among sea otters, carbon sequestration, and shellfish are more complex than the simplified depiction of their interaction implies (Fig. 2b). For example, abalone, a type of sea snail, has historically been important for trade, food, and ceremonial regalia for many British Columbia First Nations (Sloan 2003, 2004). Abalone had been sustainably fished under traditional stewardship by First Nations for at least 2000 years before European colonization. With the introduction of commercial fisheries and under the governance of the federal Department of Fisheries and Oceans Canada (DFO), abalone populations collapsed leading to the closure of abalone fisheries in 1990 (Lee et al. 2019a). Abalone cannot thrive in urchin barrens. They rely on kelp forest as a primary habitat and thus are usually present in places alongside sea otters. Abalone are also a food source for sea otter populations, and high densities of sea otters can drive down abalone populations to near extirpation. Abalone’s relationship in this system is nonlinear and exhibits threshold effects related to sea otter population densities. Too few or too many otters can lead to the extirpation of abalone from the system (Raimondi et al. 2015). Thus, while the simplistic approach might yield a decision to remove sea otters in favor of shellfish as an ES, integrating these complex relationships highlight that this could come at the expense of a more diversified shellfish community that contains abalone (see also Lee et al. 2019b).
As highlighted by our example, integrating these complex interactions in ES modelling would allow for the identification of more nuanced decision-support outcomes. While these might result in the same or similar decisions, policies can be tailored to more precisely identify “losers” among ES outcomes and mobilize ways to counterbalance these losses. These complex interactions between resource units are at the foundation of ES provisioning. Thus, while resource units are often considered individually in the SES framework, when complex interactions are important, we encourage moving beyond this and defining networks of resource interactions in SES (Fig. 2c). This would allow us to consider such complex interactions from the beginning of model conceptualization, moving away from the current dominant approach that tends to envision services as independent systems operating in isolation, which may overlook important complexity.
3.2. Integrating the flow of ES through beneficiaries
As highlighted in our review, the integration of flows to beneficiaries in ES models is still at its infancy. ES models have clearly lagged in representing humans in SES. In the SES framework, humans are integrated into both the governance systems and actors boxes. Governance systems can refer to a complex set of institutions, norms, or rules, and their study has received much attention in the natural resources management literature (Paavola and Hubacek 2013; Baird et al. 2014; Loft et al. 2015). The actors, however, also can include a wide diversity of entities. In the early iterations of the SES framework (e.g., Ostrom, 2009), this component was initially labeled as “users”, referring to individuals who use natural resources in diverse ways for sustenance, recreation, or commercial purposes. This label later referred to actors that not only include users but also expand the category to any potential individual or group participating in the system. This change is more inclusive and allows a wider range of potential applications of the SES framework, adjusting the focus from direct users of a resource to a broader representation of people that interact with the system.
Excluding recognition of beneficiary groups can be problematic when building ES models. Related to the sea otter – sea urchin case, Gregr et al. (2020) estimated the economic benefits of sea otter populations in the region focusing on four key ES and beneficiaries: tourism, invertebrate fisheries, finfish fisheries, and carbon sequestration (Fig. 3a). Overall, while sea otter populations induce economic losses for invertebrate fisheries (CA$−7.3 million per year), Gregr et al. (2020) estimated the economic benefits of sea otters through the three other services to be at around CA$53.6 million per year. These conclusions suggest there are strong benefits to protecting sea otters along the coastal areas of British Columbia since sea otter recovery is reported to be highly valuable in these areas.
Fig. 3.

However, this study does not identify specific winners and losers but estimates the overall social–economic impact of the sea otter population. While the general benefits of sea otter populations may seem uncontestable at the scale of the whole system, identifying the beneficiaries of these services is necessary to better comprehend the potential inequities in the benefits experienced by different groups (Fig. 3b). For example, local Indigenous Peoples in this system presently rely heavily on shellfish fisheries not only as a source of revenue, but also mainly as a food source and may be negatively impacted by decreased shellfish populations. This economic impact may be potentially catastrophic for certain marginalized populations. However, salmon and herring, both cultural keystone species and traditionally significant food sources for Indigenous Peoples, require kelp forests as nurseries and may benefit from sea otter reintroduction, leading to longer-term recovery of inshore fisheries and cultural practices (Gauvreau et al. 2017). Indigenous Peoples also identified erosion protection as a valuable benefit from sea otter presence, which was not incorporated into the Gregr et al. (2020) study. If focusing on other groups of beneficiaries such as commercial shellfish fisheries or commercial finfish fisheries, the overall economic consequences of sea otter populations can vary drastically both in ES of interest and directionality. Identifying these beneficiary-specific impacts can lead to much different management decisions. Further, policies could more effectively target (or not) specific groups or areas when negative impacts are projected. This would be greatly welcomed in this system, where governance is centralized around government agencies such as the DFO and the British Columbia Fish and Wildlife Branch (Pinkerton et al. 2019). Local Indigenous populations, which historically had complex governance protocols to maintain sea otter populations (Salomon et al. 2015), were completely left out of decisions around sea otter reintroductions. Many studies have highlighted the importance of decentralizing governance to actively include these actors in governance (Plagányi et al. 2013; Salomon et al. 2018; Pinkerton et al. 2019; Burt et al. 2020) and the abalone example highlights some of the catastrophic consequences of failing to do so (Chadès et al. 2012; Lee et al. 2019a).
Our example highlights the potential disparities in decision outcomes that can emerge between aggregating or disaggregating beneficiaries within ES models. Representing beneficiaries as explicit groups within the SES framework instead of being aggregated into the actors box (Fig. 3c), can help identify such disparities. Listing all possible beneficiary groups and explicitly recognizing their relationships to ES within the SES framework would be an important first step toward their integration within ES models, leading to more informative outcomes for decision-support. A simple but powerful step further would be to quantify the flows of services to these disparate groups.
4. Discussion and conclusion
Our simplified case study highlights the potential disparities that exist when using ES models integrating different levels of complexity as decision-support tools. While simplified models represent necessary first steps in understanding key concepts and leverage points in ecosystem modelling, integrating higher levels of complexity offers better representation of the intricate and subtle interactions in real systems. As illustrated in our examples, policy recommendations that come without consideration of complex ES interactions or beneficiary populations can lead to suboptimal and problematic decisions that can negatively affect both people, through their livelihoods or well-being, and nature, by disrupting essential ecological processes or reducing key biodiversity. To help guide future ES models, we proposed some key concepts building on the SES framework that aim to serve as a canvas to conceptualize ecosystems while integrating higher levels of complexity.
Among the existing modelling approaches, solutions exist to serve as a basis to integrate the complex interactions between ES, necessary to increase the usefulness and efficiency of ES models for decision support. While an ES bundle approach allows for quick identification of service interactions in relation to land use land cover maps, it lacks the level of detail to describe the complex relationships that exist between two ES. Network approaches, which break down the system into interconnected nodes, are promising for identifying complex effects such as time lags, feedbacks, or thresholds. In terms of modelling methods, system dynamics models allow for integrated interactions between variables and entities, while also integrating feedbacks. Building upon this method and drawing from the field of state-based models, which integrate different states for entities within the model, could potentially allow for modelling threshold effects and curvilinear relationships. Finally, tools such as InVEST (Sharp et al. 2015) and ARIES (Bagstad et al. 2014) include a wide variety of existing ES models that have in large part been validated and applied to a diversity of ecosystems. These are great starting points, and when ES interactions are known to be important, we advocate for developing modules to allow intricate connectedness between separate ES models.
For integrating beneficiaries, we highlight the importance of identifying local actors, how they benefit from the ecological system, and quantifying the flow of ES to each group to identify all the potential consequences of management decisions. Our review underlines beneficiaries as often overlooked within ES models. To avoid this, it is essential to incorporate these actors in the modelling process from the start (Parrott 2017). Not only does having every actor’s voice around the table allow illumination of decisions that may negatively affect communities (Taylor et al. 1990; Gismondi 1997), but management projects that lack integrating local voices in the decision processes exhibit lower chances of stakeholder buy-in and thus higher chances of failure (James 1999; Brechin et al. 2002). Of course, such participatory approaches involve accounting for complex issues that may arise such as power dynamics and conflicts (Twyman 2000; Wallerstein et al. 2019). Nevertheless, integrative workshops and collaboration will aid development of decision-support models that can help identify solutions which maximize benefits for a greater group of local actors. The SES framework is also a great tool for co-constructing a mind-map of the complex interactions that exist between governance systems and ecological systems with each group of beneficiaries (Villamor et al. 2014). This can help highlight which services matter to each group and how these services interact with various actors.
While not considered in this study, governance systems and human interactions are also a major part of SES. Integrating these concepts is the next logical step in developing and using ES models. Much work has been done regarding how policies can affect different communities within a system (Janssen and Ostrom 2006; Lebel et al. 2006; Charles et al. 2020). Conflict between beneficiaries can surely arise from tradeoffs between ES, and such concepts should be integrated when considering SES. Much work has been done around the concept of conflicts, how they arise, and how they should be considered and managed when possible (Skogen 2003; Galaz 2005; Gómez-Vázquez et al. 2009). Much of this may come in how stakeholders are engaged in the use of models as well as the role that ES models may play in decision-making. While we have focused on only some of the next steps to consider in ES modelling, better representation of the governance system and interactions between ES beneficiaries would also improve future models.
Increasing complexity in models is not without costs. Firstly, increasing the complexity of a model can lead to decreased tractability and transparency. Parsimony, the principle of finding the right equilibrium between the number of parameters and predictive power, is essential when developing models (Coelho et al. 2019). Modellers should be careful while constructing their models and follow an iterative process by adding processes individually. Evans et al. (2013) advocated from moving away from the heuristic that simple models are always better and propose key recommendations to integrate complexity in ecological models. Often, they demand increases in data quality, quantity, or complexity. Still, the rapid advances data collection, storage, analysis, and computational capacity will continue to allow for more complex and detailed models. The quality of satellite imagery and its accessibility and progress in methods for field sampling such as telemetry have led to an increase of the quantity and quality of available data. Cloud storage does not only store data but also increases the availability of data to a wide range of potential end users. Finally, computing power through technological advances and the popularization of computing clusters have and will continue to facilitate the analysis of data.
Acknowledgements
We would like to thank the editors for this opportunity to contribute to this special issue. This work was supported by the NSERC ResNet Strategic Network.
References
Ashagre BB, Platts PJ, Njana M, Burgess ND, Balmford A, Turner RK, and Schaafsma M. 2018. Integrated modelling for economic valuation of the role of forests and woodlands in drinking water provision to two African cities. Ecosystem Services, 32: 50–61.
Bagstad KJ, Johnson GW, Voigt B, and Villa F. 2013a. Spatial dynamics of ecosystem service flows: A comprehensive approach to quantifying actual services. Ecosystem Services, 4: 117–125.
Bagstad KJ, Semmens DJ, Waage S, and Winthrop R. 2013b. A comparative assessment of decision-support tools for ecosystem services quantification and valuation. Ecosystem Services, 5: 27–39.
Bagstad KJ, Semmens DJ, and Winthrop R. 2013c. Comparing approaches to spatially explicit ecosystem service modeling: A case study from the San Pedro River, Arizona. Ecosystem Services, 5: 40–50.
Bagstad KJ, Villa F, Batker D, Harrison-Cox J, Voigt B, and Johnson WR. 2014. From theoretical to actual ecosystem services: Mapping beneficiaries and spatial flows in ecosystem service assessments. Ecology and Society, 19(2): 64.
Baird J, Plummer R, and Pickering K. 2014. Priming the governance system for climate change adaptation: The application of a social-ecological inventory to engage actors in Niagara, Canada. Ecology and Society, 19(1): 3.
Balbi S, Giupponi C, Perez P, and Alberti M. 2013. A spatial agent-based model for assessing strategies of adaptation to climate and tourism demand changes in an alpine tourism destination. Environmental Modelling & Software, 45: 29–51.
Baró F, Palomo I, Zulian G, Vizcaino P, Haase D, and Gómez-Baggethun E. 2016. Mapping ecosystem service capacity, flow and demand for landscape and urban planning: A case study in the Barcelona metropolitan region. Land Use Policy, 57: 405–417.
Baró F, Gómez-Baggethun E, and Haase D. 2017. Ecosystem service bundles along the urban-rural gradient: Insights for landscape planning and management. Ecosystem Services, 24: 147–159.
Bateman IJ, Harwood AR, Mace GM, Watson RT, Abson DJ, Andrews B, et al. 2013. Bringing ecosystem services into economic decision-making: Land use in the United Kingdom. Science, 341: 45–50.
Bennett EM, and Balvanera P. 2007. The future of production systems in a globalized world. Frontiers in Ecology and the Environment, 5: 191–198.
Brechin SR, Wilshusen PR, Fortwangler CL, and West PC. 2002. Beyond the square wheel: Toward a more comprehensive understanding of biodiversity conservation as social and political process. Society & Natural Resources, 15: 41–64.
Burkhard B, Kroll F, Nedkov S. and Müller F. 2012. Mapping ecosystem service supply, demand and budgets. Ecological Indicators, 21: 17–29.
Burt JM, Barbara Ḵ, Wilson J, Malchoff T, Mack WA, Davidson SHA, and Salomon AK. 2020. Enabling coexistence: Navigating predator-induced regime shifts in human-ocean systems. People and Nature, 2: 557–574.
Byczek C, Longaretti PY, Renaud J, and Lavorel, S. 2018. Benefits of crowd-sourced GPS information for modelling the recreation ecosystem service. PLoS ONE, 13: e0202645–23.
Carswell LP, Speckman SG, and Gill VA. 2015. Chapter 12 - Shellfish Fishery Conflicts and Perceptions of Sea Otters in California and Alaska. In Sea Otter Conservation. Edited by S. E. Larson, JL Bodkin, and GR VanBlaricom. Academic Press, Boston. pp. 333–368
Castro AJ, Verburg PH, Martín-López B, Garcia-Llorente M, Cabello J, Vaughn CC, and López E. 2014. Ecosystem service trade-offs from supply to social demand: A landscape-scale spatial analysis. Landscape and Urban Planning, 132: 102–110.
Chadès I, Curtis JM, and Martin TG. 2012. Setting realistic recovery targets for two interacting endangered species, sea otter and northern abalone. Conservation Biology, 26: 1016–1025.
Chaplin-Kramer R, Sharp RP, Weil C, Bennett EM, Pascual U, Arkema KK, et al. 2019. Global modeling of nature’s contributions to people. Science, 366: 255–258.
Charles A, Loucks L, Berkes F, and Armitage D. 2020. Community science: A typology and its implications for governance of social-ecological systems. Environmental Science & Policy, 106: 77–86.
Chu H-J, Kong S-J, and Chang C-H. 2018. Spatio-temporal water quality mapping from satellite images using geographically and temporally weighted regression. International Journal of Applied Earth Observation and Geoinformation, 65: 1–11.
Coelho MTP, Diniz-Filho JA, and Rangel TF. 2019. A parsimonious view of the parsimony principle in ecology and evolution. Ecography, 42: 968–976.
Coletta VR, Pagano A, Pluchinotta I, Fratino U, Scrieciu A, Nanu F. and Giordano R. 2021. Causal Loop Diagrams for supporting Nature Based Solutions participatory design and performance assessment. Journal of Environmental Management, 280: 111668.
Costanza R, De Groot R, Sutton P, Van der Ploeg S, Anderson SJ, Kubiszewski I, et al. 2014. Changes in the global value of ecosystem services. Global Environmental Change, 26: 152–158.
Cunningham C, Tyedmers P, and Sherren K. 2018. Primary data in pollination services mapping: Potential service provision by honey bees (Apis mellifera) in Cumberland and Colchester, Nova Scotia. International Journal of Biodiversity Science, Ecosystem Services and Management, 14: 60–69.
Daw TIM, Brown K, Rosendo S, and Pomeroy R. 2011. Applying the ecosystem services concept to poverty alleviation: The need to disaggregate human well-being. Environmental Conservation, 38: 370–379.
Díaz S, Demissew S, Carabias J, Joly C, Lonsdale M, Ash N, et al. 2015. The IPBES Conceptual Framework—connecting nature and people. Current Opinion in Environmental Sustainability, 14: 1–16.
Epstein G, Vogt J, Mincey S, Cox M, and Fischer B. 2013. Missing ecology: Integrating ecological perspectives with the social-ecological system framework. International Journal of the Commons, 7.
Evans MR, Grimm V, Johst K, Knuuttila T, De Langhe R, Lessells CM, et al. 2013. Do simple models lead to generality in ecology? Trends in Ecology & Evolution, 28: 578–583.
Field RD, and Parrott L. 2017. Multi-ecosystem services networks: A new perspective for assessing landscape connectivity and resilience. Ecological Complexity, 32: 31–41.
Filbee-Dexter K, and Scheibling RE. 2014. Sea urchin barrens as alternative stable states of collapsed kelp ecosystems. Marine Ecology Progress Series, 495: 1–25.
Galaz V. 2005. Social-ecological resilience and social conflict: Institutions and strategic adaptation in Swedish water management. AMBIO: A Journal of the Human Environment, 34: 567–572.
García-Nieto AP, García-Llorente M, Iniesta-Arandia I, and Martín-López B. 2013. Mapping forest ecosystem services: From providing units to beneficiaries. Ecosystem Services, 4: 126–138.
Gauvreau AM, Lepofsky D, Rutherford M, and Reid M. 2017. “Everything revolves around the herring” the Heiltsuk–herring relationship through time. Ecology and Society, 22(2): 10.
Gismondi M. 1997. Sociology and environmental impact assessment. Canadian Journal of Sociology/Cahiers Canadiens de Sociologie, 22(4): 457–479.
Gómez-Vázquez I, Álvarez-Álvarez P, and Marey-Pérez MF. 2009. Conflicts as enhancers or barriers to the management of privately owned common land: A method to analyze the role of conflicts on a regional basis. Forest Policy and Economics, 11: 617–627.
Gonzalez-Redin J, Luque S, Poggio L, Smith R, and Gimona A. 2016. Spatial Bayesian belief networks as a planning decision tool for mapping ecosystem services trade-offs on forested landscapes. Environmental Research, 144: 15–26.
Grafius DR, Edmondson JL, Norton BA, Clark R, Mears M, Leake JR, et al. 2020. Estimating food production in an urban landscape. Scientific Reports, 10: 5141.
Gregr EJ, Christensen V, Nichol L, Martone RG, Markel RW, Watson JC, et al. 2020. Cascading social-ecological costs and benefits triggered by a recovering keystone predator. Science, 368: 1243–1247.
Groff SC, Loftin CS, Drummond F, Bushmann S, and McGill B. 2016. Parameterization of the InVEST Crop Pollination Model to spatially predict abundance of wild blueberry (Vaccinium angustifolium Aiton) native bee pollinators in Maine, USA. Environmental Modelling and Software, 79: 1–9.
Grunewald K, Herold H, Marzelli S, Meinel G, Richter B, Syrbe R-U, and Walz U. 2016. Assessment of ecosystem services at the national level in Germany—Illustration of the concept and the development of indicators by way of the example wood provision. Ecological Indicators, 70: 181–195.
Habib TJ, Heckbert S, Wilson JJ, Vandenbroeck AJ, Cranston J, and Farr DR. 2016. Impacts of land-use management on ecosystem services and biodiversity: An agent-based modelling approach. PeerJ, 4: e2814.
Havinga I, Bogaart PW, Hein L, and Tuia D. 2020. Defining and spatially modelling cultural ecosystem services using crowdsourced data. Ecosystem Services, 43: 101091.
Howe J, McMahon ET, and Propst L. 2012. Balancing nature and commerce in gateway communities. Island Press.
James RF. 1999. Public Participation in Environmental Decision–Making–Rhetoric to Reality? Paper presented to. Page the International Symposium on Society and Resource Management. Citeseer.
Janssen MA, and Ostrom E. 2006. Governing social-ecological systems. Handbook of Computational Economics, 2: 1465–1509.
Johnson, JA, Jones SK, Wood SL, Chaplin-Kramer R, Hawthorne PL, Mulligan M, et al. 2019. Mapping Ecosystem Services to Human Well-being: A toolkit to support integrated landscape management for the SDGs. Ecological Applications, 29: e01985.
Keeler BL, Wood SA, Polasky S, Kling C, Filstrup CT, and Downing JA. 2015. Recreational demand for clean water: Evidence from geotagged photographs by visitors to lakes. Frontiers in Ecology and the Environment, 13: 76–81.
Kourgialas NN, and Karatzas GP. 2011. Gestion des inondations et méthode de modélisation sous SIG pour évaluer les zones d’aléa inondation-une étude de cas. Hydrological Sciences Journal, 56: 212–225.
Kremen C. 2005. Managing ecosystem services: What do we need to know about their ecology? Ecology letters, 8: 468–479.
Lebel L, Anderies JM, Campbell B, Folke C, Hatfield-Dodds S, Hughes TP, and Wilson J. 2006. Governance and the capacity to manage resilience in regional social-ecological systems. Ecology and Society, 11(1): 19.
Lee LC, Watson JC, Trebilco R, and Salomon AK. 2016. Indirect effects and prey behavior mediate interactions between an endangered prey and recovering predator. Ecosphere, 7: e01604.
Lee LC, Reid M, Jones R, Winbourne J, Rutherford M, and Salomon AK. 2019a. Drawing on indigenous governance and stewardship to build resilient coastal fisheries: People and abalone along Canada’s northwest coast. Marine Policy, 109: 103701.
Lee LC, Thorley J, Watson J, Reid M, and Salomon AK. 2019b. Diverse knowledge systems reveal social–ecological dynamics that inform species conservation status. Conservation Letters, 12: e12613.
Li Y, Duan X, Li Y, Li Y, and Pan X. 2019. A method for data downscaling in estimations of food-provisioning service in a mountainous region. Soil and Tillage Research, 195: 104379.
Lindegren M, Holt BG, MacKenzie BR, and Rahbek C. 2018. A global mismatch in the protection of multiple marine biodiversity components and ecosystem services. Scientific Reports, 8: 4099.
Ling SD, Scheibling RE, Rassweiler A, Johnson CR, Shears N, Connell SD, et al. 2015. Global regime shift dynamics of catastrophic sea urchin overgrazing. Philosophical Transactions of the Royal Society B: Biological Sciences, 370: 20130269.
Liu J, Hull V, Batistella M, DeFries R, Dietz T, Fu F, et al. 2013. Framing sustainability in a telecoupled world. Ecology and Society, 18.
Loft L, Mann C, and Hansjürgens B. 2015. Challenges in ecosystem services governance: Multi-levels, multi-actors, multi-rationalities. Ecosystem Services, 16:150–157.
Lonsdorf E, Kremen C, Ricketts T, Winfree R, Williams N, and Greenleaf S. 2009. Modelling pollination services across agricultural landscapes. Annals of Botany, 103: 1589–1600.
Lotan A, Kost R, Mandelik Y, Peled Y, Chakuki D, Shamir SZ, and Ram Y. 2018. National scale mapping of ecosystem services in Israel–genetic resources, pollination and cultural services. One Ecosystem, 3: e25494.
Ma S, Smailes M, Zheng H, and Robinson BE. 2019. Who is vulnerable to ecosystem service change? Reconciling locally disaggregated ecosystem service supply and demand. Ecological Economics, 157: 312–320.
Mace GM, Norris K, and Fitter AH. 2012. Biodiversity and ecosystem services: A multilayered relationship. Trends in Ecology and Evolution, 27: 19–26.
Martínez-Harms MJ, and Balvanera P. 2012. Methods for mapping ecosystem service supply: A review. International Journal of Biodiversity Science, Ecosystem Services & Management, 8: 17–25.
Martínez-López J, Bagstad KJ, Balbi S, Magrach A, Voigt B, Athanasiadis I, et al. 2019. Towards globally customizable ecosystem service models. Science of the Total Environment, 650: 2325–2336.
McGinnis MD, and Ostrom E. 2014. Social-ecological system framework: Initial changes and continuing challenges. Ecology and Society, 19.
McVittie A, Norton L, Martin-Ortega J, Siameti I, Glenk K, and Aalders I. 2015. Operationalizing an ecosystem services-based approach using Bayesian Belief Networks: An application to riparian buffer strips. Ecological Economics, 110: 15–27.
Montoya D, Haegeman B, Gaba S, de Mazancourt C, Bretagnolle V, and Loreau M. 2019. Trade-offs in the provisioning and stability of ecosystem services in agroecosystems. Ecological Applications, 29: 1–12.
Nations U. 2015. Transforming our world: The 2030 agenda for sustainable development. United Nations, Department of Economic and Social Affairs, New York.
Ostrom E. 2009. A General Framework for Analyzing Sustainability of Social-Ecological Systems. Science, 325: 419–422.
Ouyang Z, Song C, Zheng H, Polasky S, Xiao Y, Bateman IJ, et al. 2020. Using gross ecosystem product (GEP) to value nature in decision making. Proceedings of the National Academy of Sciences, 117: 14593–14601.
Paavola J, and Hubacek K. 2013. Ecosystem services, governance, and stakeholder participation: An introduction. Ecology and Society, 18(4): 42.
Parrott L. 2017. The modelling spiral for solving ‘wicked’environmental problems: Guidance for stakeholder involvement and collaborative model development. Methods in Ecology and Evolution, 8: 1005–1011.
Partelow S. 2018. A review of the social-ecological systems framework: Applications, methods, modifications, and challenges. Ecology and Society, 23(4): 36.
Pechanec V, Purkyt J, Benc A, Nwaogu C, Štěrbová L, and Cudlín P. 2018. Modelling of the carbon sequestration and its prediction under climate change. Ecological Informatics, 47: 50–54.
Pinkerton E, Salomon AK, and Dragon F. 2019. Reconciling social justice and ecosystem-based management in the wake of a successful predator reintroduction. Canadian Journal of Fisheries and Aquatic Sciences, 76: 1031–1039.
Plagányi ÉE, van Putten I, Hutton T, Deng RA, Dennis D, Pascoe S, et al. 2013. Integrating indigenous livelihood and lifestyle objectives in managing a natural resource. Proceedings of the National Academy of Sciences, 110: 3639–3644.
Polasky S, Nelson E, Pennington D, and Johnson KA. 2011. The impact of land-use change on ecosystem services, biodiversity and returns to landowners: A case study in the state of Minnesota. Environmental and Resource Economics, 48: 219–242.
Queiroz C, Meacham M, Richter K, Norström AV, Andersson E, Norberg J, and Peterson G. 2015. Mapping bundles of ecosystem services reveals distinct types of multifunctionality within a Swedish landscape. Ambio, 44: 89–101.
Raimondi P, Jurgens LJ, and Tinker MT. 2015. Evaluating potential conservation conflicts between two listed species: Sea otters and black abalone. Ecology, 96: 3102–3108.
Raudsepp-Hearne C, Peterson GD, and Bennett EM. 2010a. Ecosystem service bundles for analyzing tradeoffs in diverse landscapes. Proceedings of the National Academy of Sciences, 107: 5242–5247.
Raudsepp-Hearne C, Peterson GD, Tengö M, Bennett EM, Holland T, Benessaiah K, et al. 2010b. Untangling the environmentalist’s paradox: Why is human well-being increasing as ecosystem services degrade? BioScience, 60: 576–589.
Redhead JW, Stratford C, Sharps K, Jones L, Ziv G, Clarke D, et al. 2016. Empirical validation of the InVEST water yield ecosystem service model at a national scale. Science of the Total Environment, 569–570: 1418–1426.
Rieb JT, Chaplin-Kramer R, Daily GC, Armsworth PR, Böhning-Gaese K, Bonn A, et al. 2017. When, where, and how nature matters for ecosystem services: Challenges for the next generation of ecosystem service models. BioScience, 67: 820–833.
Robinson BE, Zheng H, and Peng W. 2019. Disaggregating livelihood dependence on ecosystem services to inform land management. Ecosystem Services, 36: 100902.
Robinson DA, Jackson BM, Clothier BE, Dominati EJ, Marchant SC, Cooper DM, and Bristow KL. 2013. Advances in soil ecosystem services: Concepts, models, and applications for earth system life support. Vadose Zone Journal, 12: vzj2013.01.0027–13.
Rodríguez JP, Beard TD, Bennett EM, Cumming GS, Cork SJ, Agard J, et al. 2006. Trade-offs across Space, Time, and Ecosystem Services. Ecology and Society, 11(1): 28
Rova S, Meire P, Müller F, Simeoni M, and Pranovi F. 2019. A Petri net modeling approach to explore the temporal dynamics of the provision of multiple ecosystem services. Science of the Total Environment, 655: 1047–1061.
Salomon AK, Kii’iljuus Barb JW, White XE, Tanape N. Sr, and Happynook TM. 2015. First Nations perspectives on sea otter conservation in British Columbia and Alaska: Insights into coupled human–ocean systems.In Sea otter conservation, Elsevier. pp. 301–331.
Salomon AK, Lertzman K, Brown K, Wilson KB, Secord D, and McKechnie I. 2018. Democratizing conservation science and practice. Ecology and Society, 23(1): 44.
Schirpke U, Leitinger G, Tasser E, Schermer M, Steinbacher M, and Tappeiner U. 2013. Multiple ecosystem services of a changing Alpine landscape: Past, present and future. International Journal of Biodiversity Science, Ecosystem Services and Management, 9: 123–135.
Schirpke U, Candiago S, Vigl LE, Jäger H, Labadini A, Marsoner T, et al. 2019. Integrating supply, flow and demand to enhance the understanding of interactions among multiple ecosystem services. Science of The Total Environment, 651: 928–941.
Schulp CJE, Alkemade R, Klein Goldewijk K, and Petz K. 2012. Mapping ecosystem functions and services in Eastern Europe using global-scale data sets. International Journal of Biodiversity Science Ecosystem Services and Management, 8: 156–168.
Schulp CJ, Lautenbach S, and Verburg PH. 2014. Quantifying and mapping ecosystem services: Demand and supply of pollination in the European Union. Ecological Indicators, 36: 131–141.
Sharp R, Tallis H, Ricketts T, and Guerry A. 2015. Invest version 3.2. 0 User’s Guide.
Skogen K. 2003. Adapting adaptive management to a cultural understanding of land use conflicts. Society and Natural Resources, 16: 435–450.
Sloan NA. 2003. Evidence of California-area abalone shell in Haida trade and culture. Canadian Journal of Archaeology/Journal Canadien d’Archéologie, 27(2): 273–286.
Sloan NA. 2004. Northern abalone: Using an invertebrate to focus marine conservation ideas and values. Coastal Management, 32: 129–143.
Spake R, Lasseur R, Crouzat E, Bullock JM, Lavorel S, Parks KE, Schaafsma M, et al. 2017. Unpacking ecosystem service bundles: Towards predictive mapping of synergies and trade-offs between ecosystem services. Global Environmental Change, 47: 37–50.
Su Y-F, Liou J-J, Hou J-C, Hung W-C, Hsu S-M, Lien Y-T, et al. 2008. A Multivariate Model for Coastal Water Quality Mapping Using Satellite Remote Sensing Images. Sensors, 8: 6321–6339.
Syrbe RU, and Grunewald K. 2017. Ecosystem service supply and demand–the challenge to balance spatial mismatches. International Journal of Biodiversity Science, Ecosystem Services and Management, 13: 148–161.
Syrbe RU, Schorcht M, Grunewald K, and Meinel G. 2018. Indicators for a nationwide monitoring of ecosystem services in Germany exemplified by the mitigation of soil erosion by water. Ecological Indicators, 94: 46–54.
Tao Y, Wang H, Ou W, and Guo J. 2018. A land-cover-based approach to assessing ecosystem services supply and demand dynamics in the rapidly urbanizing Yangtze River Delta region. Land Use Policy, 72: 250–258.
Taylor CN, Bryan CH, and Goodrich C. 1990. Social assessment: Theory, process and techniques. Centre for Resource Management.
Thiel A, Adamseged ME, and Baake C. 2015. Evaluating an instrument for institutional crafting: How Ostrom’s social–ecological systems framework is applied. Environmental Science & Policy, 53: 152–164.
Turner KG, Odgaard MV, Bøcher PK, Dalgaard T, and Svenning JC. 2014. Bundling ecosystem services in Denmark: Trade-offs and synergies in a cultural landscape. Landscape and Urban Planning, 125: 89–104.
Twyman C. 2000. Participatory conservation? Community-based natural resource management in Botswana. Geographical Journal, 166: 323–335.
Vannier C, Lasseur R, Crouzat E, Byczek C, Lafond V, Cordonnier T, et al. 2019. Mapping ecosystem services bundles in a heterogeneous mountain region. Ecosystems and People, 15: 74–88.
Villa F, Bagstad KJ, Voigt B, Johnson GW, Portela R, Honzák M, and Batker D. 2014. A methodology for adaptable and robust ecosystem services assessment. PloS ONE, 9: e91001.
Villamor GB, Palomo I, Santiago CAL, Oteros-Rozas E, and Hill J. 2014. Assessing stakeholders’ perceptions and values towards social-ecological systems using participatory methods. Ecological Processes, 3: 1–12.
Vojtek M, and Vojteková J. 2016. Flood hazard and flood risk assessment at the local spatial scale: A case study. Geomatics, Natural Hazards and Risk, 7: 1973–1992.
Wallerstein N, Muhammad M, Sanchez-Youngman S, Rodriguez Espinosa P, Avila M, Baker EA, et al. 2019. Power dynamics in community-based participatory research: A multiple–case study analysis of partnering contexts, histories, and practices. Health Education and Behavior, 46: 19S–32S.
Wang L, Zheng H, Wen Z, Liu L, Robinson BE, Li R, et al. 2019. Ecosystem service synergies/trade-offs informing the supply-demand match of ecosystem services: Framework and application. Ecosystem Services, 37: 100939.
Watson J, and Estes JA. 2011. Stability, resilience, and phase shifts in rocky subtidal communities along the west coast of Vancouver Island, Canada. Ecological Monographs, 81: 215–239.
Yan H, Pan L, Xue Z, Zhen L, Bai X, Hu Y, and Huang H-Q. 2019. Agent-Based Modeling of Sustainable Ecological Consumption for Grasslands: A Case Study of Inner Mongolia, China. Sustainability, 11: 2261.
Yang G, Ge Y, Xue H, Yang W, Shi Y, Peng C, et al. 2015. Using ecosystem service bundles to detect trade-offs and synergies across urban-rural complexes. Landscape and Urban Planning, 136: 110–121.
Zoderer BM, Tasser E, Carver S, and Tappeiner U. 2019. Stakeholder perspectives on ecosystem service supply and ecosystem service demand bundles. Ecosystem Services, 37: 100938.
Information & Authors
Information
Published In
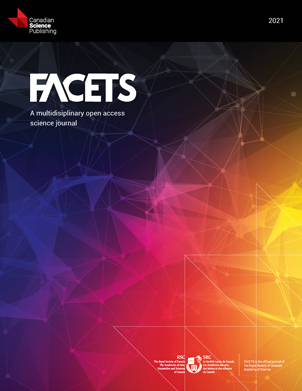
FACETS
Volume 6 • Number 1 • January 2021
Pages: 1649 - 1669
Editor: Evan Fraser
History
Received: 31 December 2020
Accepted: 19 April 2021
Version of record online: 29 September 2021
Notes
This paper is part of a Collection titled “Food, Fiber, Fuel, and Function: Pathways to manage ecosystem services in Canada’s working landscapes”.
Copyright
© 2021 Thierry et al. This work is licensed under a Creative Commons Attribution 4.0 International License (CC BY 4.0), which permits unrestricted use, distribution, and reproduction in any medium, provided the original author(s) and source are credited.
Data Availability Statement
All relevant data are within the paper.
Key Words
Sections
Subjects
Plain Language Summary
Proposing new pathways to improve ecosystem service accounting
Authors
Author Contributions
All conceived and designed the study.
HT performed the experiments/collected the data.
HT analyzed and interpreted the data.
All contributed resources.
All drafted or revised the manuscript.
Competing Interests
The authors have declared that no competing interests exist.
Metrics & Citations
Metrics
Other Metrics
Citations
Cite As
Hugo Thierry, Lael Parrott, and Brian Robinson. 2021. Next steps for ecosystem service models: integrating complex interactions and beneficiaries. FACETS.
6(): 1649-1669. https://doi.org/10.1139/facets-2020-0116
Export Citations
If you have the appropriate software installed, you can download article citation data to the citation manager of your choice. Simply select your manager software from the list below and click Download.
Cited by
1. A functional connectivity approach for exploring interactions of multiple ecosystem services in the context of agricultural landscapes in the Canadian prairies
2. Place-based data justice practices for collaborative conservation research: A critical review
3. Examining policy−institution−program (PIP) responses against the drivers of ecosystem dynamics. A chronological review (1960–2020) from Nepal
4. Systematic review of ecosystem services flow measurement: Main concepts, methods, applications and future directions
5. Modeling multiple ecosystem services and beneficiaries of riparian reforestation in Costa Rica
6. Mapping the functional connectivity of ecosystem services supply across a regional landscape
7. Facing the challenges of using place-based social-ecological research to support ecosystem service governance at multiple scales
8. Managing Canada’s land- and seascapes for multiple ecosystem services in the Anthropocene: introduction to the Food, Fiber, Fuel, and Function collection