Amplicon-based and metagenomic approaches provide insights into toxigenic potential in understudied Atlantic Canadian lakes
Abstract
Cyanobacterial blooms and their toxigenic potential threaten freshwater resources worldwide. In Atlantic Canada, despite an increase of cyanobacterial blooms in the last decade, little is known about the toxigenic potential and the taxonomic affiliation of bloom-forming cyanobacteria. In this study, we employed polymerase chain reaction (PCR) and metagenomic approaches to assess the potential for cyanotoxin and other bioactive metabolite production in Harvey Lake (oligotrophic) and Washademoak Lake (mesotrophic) in New Brunswick, Canada, during summer and early fall months. The PCR survey detected the potential for microcystin (hepatotoxin) and anatoxin-a (neurotoxin) production in both lakes, despite a cyanobacterial bloom only being visible in Washademoak. Genus-specific PCR associated microcystin production potential with the presence of Microcystis in both lakes. The metagenomic strategy provided insight into temporal variations in the microbial communities of both lakes. It also permitted the recovery of a near-complete Microcystis aeruginosa genome with the genetic complement to produce microcystin and other bioactive metabolites such as piricyclamide, micropeptin/cyanopeptolin, and aeruginosin. Our approaches demonstrate the potential for production of a diverse complement of bioactive compounds and establish important baseline data for future studies of understudied lakes, which are frequently affected by cyanobacterial blooms.
Introduction
Cyanobacteria have the capacity to produce a myriad of secondary metabolites, some of them with potent toxic effects (i.e., microcystin, anatoxin-a, nodularins, cylindrospermopsins, and saxitoxins) affecting humans and diverse types of animals (Calteau et al. 2014; Chorus and Welker 2021). These toxic compounds, generically called cyanotoxins, become a major concern when produced by high-density blooms or dense benthic mats, as they threaten aquatic ecosystems, human health, and activities (Codd et al. 2005).
In recent years, reports of cyanobacterial blooms in freshwater systems have been increasing across Canada, although their detection relies mostly on provincial agencies that use dissimilar approaches, complicating direct comparisons (Pick 2016; Smith 2019). Blooms dominated by species of Microcystis are the most concerning due to their large-scale occurrence and their potential to produce microcystins, which have been detected in freshwater systems of every Canadian province at concentration levels of concern (Orihel et al. 2012). Most cyanobacteria and cyanotoxin studies have focused on conspicuous blooms occurring in large inland waters on the edge of the Canadian Shield (Pick 2016), leaving more than one million lakes distributed across Canada understudied.
In New Brunswick (Atlantic Canada), the provincial government (via the Office of the Chief Medical Officer of Health) reported that 19 water bodies have experienced cyanobacterial blooms, with 14 of them first reported in the last 5 years. However, the vast majority of these provincial reports have relied on morphological identification via microscopy (New Brunswick Department of Environment and Local Government 2019), which complicates accurate taxonomic identification and makes it impossible to determine the toxigenic potential of the cyanobacteria forming the blooms (Vezie et al. 1998; Al-Tebrineh et al. 2012). The increased reporting of cyanobacterial blooms and lack of toxicity data in this region highlights the necessity for further studies into the toxigenic potential of cyanobacteria in these freshwater systems. In addition to surface blooms, the proliferation of benthic cyanobacteria has recently been associated with toxicity in New Brunswick freshwaters. The ingestion of anatoxin-producing cyanobacterial mats from the Wolastoq/Saint John River has caused the intoxication and death of at least three dogs in Fredericton (McCarron et al. 2019).
Polymerase chain reaction (PCR)-based methods have been used extensively to detect and evaluate the abundance of toxigenic cyanobacteria in freshwater systems by amplifying sequences of genes involved in toxin biosynthesis (Baker et al. 2002; Humbert et al. 2010; Weller 2011). The inherent specificity of PCR is key for sensitivity, but it is also a disadvantage because it requires prior knowledge of the target sequence for efficient primer design (Weller 2011). Additionally, PCR-based surveys are not adequate to explore and predict broad toxigenic potentials (i.e., genetic capacity to produce a wide-range of bioactive secondary metabolites) of cyanobacteria and other microorganisms present in environmental samples. The use of shotgun DNA sequencing of environmental samples (i.e., metagenomics) and the development of bioinformatic tools have enabled the recovery of almost complete microbial genomes (McMahon 2015; Chen et al. 2020; Frioux et al. 2020) and detection of complete biosynthetic gene clusters (Cuadrat et al. 2018; Hannigan et al. 2019), overcoming the limitations of PCR-based methods (Dittmann et al. 2015; Micallef et al. 2015; Zhang and Zhang 2015).
Harvey and Washademoak Lakes (New Brunswick, Canada) have experienced seasonal cyanobacterial blooms for the past 6 years. These lakes represent different tropic regimes; Harvey Lake is categorized as oligotrophic, whereas Washademoak Lake is mesotrophic (New Brunswick Department of Environment and Local Government 2019). In this study, we aimed to determine if the cyanobacterial populations we observed during the summer–fall period of 2018 have the potential to produce cyanotoxins. We used PCR-based detection to investigate the presence of cyanobacterial genes associated with the biosynthesis of both microcystin and anatoxin-a in these lakes. We also used metagenomics to explore the toxigenic potential in both lakes and were able to assemble a near-complete genome of a Microcystis aeruginosa strain from the Washademoak with the gene repertoire to produce microcystin and other bioactive secondary metabolites.
Materials and methods
Field sampling and processing
Samples were collected bi-weekly from three sites around each of Harvey Lake and Washademoak Lake from 30 July to 10 November 2018 (Fig. 1). Harvey Lake is located in York County, New Brunswick, and is approximately 6 km long and 1.25 km wide, with a maximum depth of 13.6 m. It is classified as an oligotrophic lake, based on a median total phosphorus concentration of 5 μg·L−1 from 2005 to 2016 (New Brunswick Department of Environment and Local Government 2019). It is included in the Government of New Brunswick fish-stocking program, receiving landlocked Atlantic salmon. Washademoak Lake is a widening of the Canaan River upstream of the Saint John River in Queen’s County, New Brunswick. It has a maximal depth of 28.7 m, and is approximately 22 km long and 2.5 km at the widest point. It is mesotrophic, based on a median total phosphorus concentration of 17 μg·L−1 from 2005 to 2016 (New Brunswick Department of Environment and Local Government 2019).
Fig. 1.

Surface water temperature was recorded, and surface samples were collected in 4-L jugs by wading, then transported back to the lab at in situ temperatures for immediate processing. Maximal volumes of each sample were filtered through 3-μm nominal pore-size polycarbonate filters (MilliporeSigma, Burlington, MA, USA) (Table S1). Total DNA was extracted from filters using MoBio’s PowerWater DNA Isolation Kit (now QIAGEN, Germantown, MD, USA) following the manufacturer’s instructions. Extracts were eluted in 100 μL Tris and stored at −20 °C until further analysis.
Culturing toxic strains for PCR controls
Three strains of cyanobacteria were used as positive controls for the PCR-based assays. Microcystis aeruginosa CPCC 300 (Canadian Phycological Culture Centre, Waterloo, Canada) was grown on a 14:10 light–dark cycle in BG11 medium at 20 °C. Dolichospermum lemmermanii NIVA-CYA83/1 (syn. Anabaena lemmermanii NIVA-CYA83/1) and Planktothrix agardhii NIVA-CYA126 (Norwegian Institute for Water Research, Oslo, Norway) were grown on a 14:10 light–dark cycle in Z8 medium at 18 °C. Cultures were either extracted using MoBio’s Microbial DNA Isolation Kit and eluted in 100 μL of TrisEDTA (Microcystis), or MilliporeSigma’s Gene Elute Bacterial Extraction Kit and eluted in 200 μL of TrisEDTA (Dolichospermum and Planktothrix). DNA extracts were stored at −20 °C until further analysis.
Detection of the microcystin synthetase E and anatoxin-a C genes
The presence/absence of the general microcystin synthetase E gene (mcyE) was determined using PCR-based detection with mcyE-F2 (Vaitomaa et al. 2003) and mcyE-R4 (Rantala et al. 2004) primers (Table S2). All reactions were performed in 20-μL volumes with 1 μL of DNA template per reaction, and positive (appropriate culture extracts) and negative (ddH2O) controls were included in each PCR run. For the initial screening with mcyE-F2 and mcyE-R4, the PCR mixture contained 1 × Mg (+) PCR buffer, 250 μM dNTPs, 0.5 μM of forward and reverse primers, 0.5 U of Ex Taq DNA (Takara Bio, San Jose, CA) polymerase and sterile water. An MJ Mini Personal Thermal Cycler (BioRad, Hercules, CA, USA) was used for the PCRs cycles with initial denaturation step at 95 °C for 3 min, followed by 24 cycles of 94 °C for 30 s, 60 °C for 30 s, and 72 °C for 1 min, and a final extension at 72 °C for 10 min. Samples that tested positive for mcyE were then assessed with genus-specific assays to identify the source of the toxin genes. For Microcystis-specific mcyE, 20-μL PCRs were set-up with 1X Ex Taq Buffer Mg2+ plus (Takara Bio), 250 μM dNTPs, 0.5 μM of mcyE-F2 and MicmcyE-R8 primers (Table S2), 1.25 U of Ex Taq polymerase (Takara Bio), and sterile water (Vaitomaa et al. 2003). The PCR amplification included an initial denaturation step at 95 °C for 3 min, followed by 38 cycles of 94 °C for 30 s, 60 °C for 30 s, 72 °C for 60 s, and a final extension at 72 °C for 10 min (Vaitomaa et al. 2003). For Planktothrix-specific mcyE, PCRs were set-up with 1X Standard Taq Reaction Buffer (New England Biolabs, Ipswich, MA, USA), 62.5 μM dNTPs, 0.5 μM of mcyE-F2 and PlamcyE-R3 primers (Table S2), 1.25 U of Taq DNA polymerase (New England Biolabs), and sterile water to a final volume of 20 μL (Rantala et al. 2006). The PCR amplification was as follows: initial denaturation step at 95 °C for 3 min, followed by 35 cycles of 94 °C for 30 s, 55 °C for 45 s, 68 °C for 90 s, and a final extension at 68 °C for 5 min (Rantala et al. 2006). The Dolichospermum-specific mcyE reaction was set-up in 20 μL 1X Ex Taq Buffer Mg2+ plus (Takara Bio Clontech), 250 μM dNTPs, 5.0 μM of mcyE-F2 and 0.5 μM AnamcyE-R12 primers (Table S2), 1.25 U of Ex Taq polymerase (Takara Bio), and sterile water (Doblin et al. 2007). A touchdown PCR program was used, consisting of an initial denaturation step at 98 °C for 3 min, followed by 25 cycles of 94 °C for 30 s, 65 °C for 30 s decreasing by 0.5 °C per cycle, 72 °C for 60 s, and a final extension at 72 °C for 5 min (Doblin et al. 2007). PCR products were run at 70 V for 40 min on 1–1.5% TrisBorateEDTA agarose gels, stained with 1X SYBRSafe Gel stain, (Invitrogen, Waltham, MA, USA), and visualized using a BioRad gel documentation system.
The presence/absence of the anatoxin-a biosynthesis C gene (anaC) was assessed by PCR amplification with the anaC-genF and anaC-genR primers (Table S2; Rantala-Ylinen et al. 2011) as follows: a 20 μL PCR was set-up with 1 μL of DNA, 1X Ex Taq Buffer Mg2+ plus (Takara Bio), 200 μM dNTPs, 0.5 μM of anaC-genF and anaC-genR primers (Table S2), 1.25 U of Ex Taq polymerase (Takara Bio), and sterile water (Rantala-Ylinen et al. 2011). PCR conditions for this reaction started with an initial denaturation step at 94 °C for 2 min, followed by 35 cycles of 94 °C for 30 s, 55.5 °C for 30 s, 72 °C for 30 s, and a final extension at 72 °C for 5 min (Rantala-Ylinen et al. 2011).
All samples negative for mcyE and anaC were assessed for PCR inhibition using 16 S rRNA gene amplification with the universal bacterial primers 27 F and 1492 R (Lane 1991). The PCR reaction mix (25 μL) consisted of: 1 μL of extracted genomic DNA, 1x reaction buffer, 2 mM MgCl2, 0.2 mM dNTP, 1 μM of each primer, Taq polymerase (1 U, New England Biolabs) and sterile water. The cycling conditions were as follows: initial denaturation at 94 °C for 2 min followed by 30 cycles of 94 °C for 1 min, 55 °C for 2 min, and 72 °C for 2 min. The final cycle was followed by extension at 72 °C for 5 min.
Shotgun sequencing of total DNA from environmental samples
To investigate seasonal changes in the microbial community composition and its potential to produce cyanotoxins and other bioactive metabolites, we selected 8 DNA samples from each lake (Harvey (site A) and Washademoak (site B)) for shotgun Illumina sequencing (see Table S1 for sampling dates details). The 16 libraries (∼450 base pairs insert length) were prepared with the Illumina Nextera Flex kit (Illumina, San Diego, CA, USA) for MiSeq+NextSeq platforms and sequenced (150 base pairs paired-end reads) at the Integrated Microbiology Resource of Dalhousie University (imr.bio/) using the Illumina NextSeq 550 system.
Read annotation and community composition analysis
Raw Illumina reads were uploaded to MG-RAST 4.0.3 (Meyer et al. 2008) (mg-rast.org) for quality control. Then, reads were assigned to taxonomic units using BLAT (Kent 2002) and the M5NR database (Wilke et al. 2012) as reference, considering 60% sequence similarity in a protein sequence (conceptual translations) of at least 15 amino acids and considering an E-value cut-off ≤ 1e–5.
Read processing and assembly variants
We processed the raw Illumina reads for quality control with bbduk (jgi.doe.gov/data-and-tools/bb-tools/) considering the parameters ktrim = r k = 23 mink = 11 hdist = 1. Sanitized reads were then initially assembled with the metaSPAdes pipeline (SPAdes v3.12.0) (Bankevich et al. 2012) considering the k-mer options -k21,33,55,77,99. Then we used SqueezeMeta v1.1.0 (Tamames and Puente-Sánchez 2019) to identify and retrieve individual metagenome-assembled genomes (MAGs) from each DNA sample. The SqueezeMeta pipeline first bins the assembled sequences with both MaxBin (Wu et al. 2015) and MetaBat (Kang et al. 2015), then merges equivalent pairs of binned sequences with DAS Tool (Sieber et al. 2018), and evaluates for quality assembly with CheckM (Parks et al. 2015). Each merged bin represents an individual MAG.
To investigate the phylogenetic affiliation of the recovered good quality MAGs (i.e., completeness ≥ 90% and contamination < 5%), we used the Insert Set of Genomes Into Species Tree 2.1.10 application of KBase (Arkin et al. 2018), which estimates an approximately maximum-likelihood phylogenetic tree with FastTree-2 (Price et al. 2010) considering a reference of 49 Clusters of Orthologs Groups from closely related genomes selected based on sequence identity. The phylogenetic analysis allowed the preliminary identification of good quality cyanobacterial MAGs unambiguously affiliated to the genus Microcystis (details provided in the Results section).
Assembly and annotation of the genome of a microcystin-producing cyanobacteria from Washademoak Lake
To improve the assembly (scaffolding) of the Microcystis MAG, likely linked to microcystin production (e.g., 27 August 2018 sample) in Washademoak Lake, we first performed independent assemblies of the 27 August read collection with MEGAHIT v1.2.9 (de novo assembly) (Li et al. 2015), MetaCompass v2.0.0 (reference-guided assembly with the complete genome of Microcystis aeruginosa NIES-843 (accession number NC_010296) as reference) (Cepeda et al. 2017), and SPAdes v3.12.0 (with the “trusted contigs” option, using a reference-guided assembly) (Bankevich et al. 2012). Then, we used Metassembler v1.5 (Wences and Schatz 2015) to merge the Microcystis MAGs recovered from our four alternative independent assembling tries, which included the initial metaSPAdes try plus the MEGAHIT, MetaCompass, and SPAdes trusted-contigs assemblies. After merging the four Microcystis MAGs, we used GenomeFinisher v1.4 (Guizelini et al. 2016) and the complete genome of M. aeruginosa NIES-843 as reference for a final scaffolding step.
We used the stand-alone software package of the NCBI Prokaryotic Genome Annotation Pipeline (Tatusova et al. 2016) to annotate the resulting Microcystis scaffolds. Then, to identify homologous genes shared between our Microcystis scaffolds and the complete genomes of the closely related M. aeruginosa strains NIES-843 and NIES-2549 (Kaneko et al. 2007; Yamaguchi et al. 2015), we used the software GET_HOMOLOGUES v3.3.2 (Contreras-Moreira and Vinuesa 2013) with the orthoMCL algorithm (75% minimum coverage in BLAST pairwise alignments and max E-value 1e–05) (Li et al. 2003). The sequence Average Nucleotide Identity (ANI) between our scaffolded Microcystis genome and data from different Microcystis species, including isolates from freshwater bodies in Canada (Pérez-Carrascal et al. 2019), was calculated with OrthoANI v1.2 with default parameters (Lee et al. 2016). Contig mapping to reference sequences was carried out with BWA-MEM (Li 2013) with default parameters.
Prediction of biosynthetic gene clusters in the Microcystis genome
To investigate the genetic potential of the Microcystis MAG from Washademoak Lake to produce secondary metabolites, we used the DeepBGC v0.1.18 tool (Hannigan et al. 2019) with a 0.9 threshold score to predict the presence of biosynthetic gene clusters (BGCs) and antiSMASH online bacterial version (Blin et al. 2019) to get the most similar known BGC according to MIBiG 2.0 database (Kautsar et al. 2019). We also used DeepBGC to predict the “activity” and “product class” associated to each detected BGC.
Results
Field observations
Chalky blue-green clumps of cyanobacteria (∼1 mm in diameter) were visible in Washademoak Lake from 30 July (the first day of sampling) through to 23 October 2018, with an intense bloom visible at site B on 30 July. Dolichospermum sp. was identified via microscopy as the dominant species in the 30 July sample. Similar clumps were also observed at Harvey Lake from 13 August to 25 September, though their abundance was very low relative to Washademoak Lake, and this water body was not considered to be blooming during our study period.
Surface water temperature was highest in Washademoak Lake on 30 July (31 °C) and on 13 August in Harvey Lake (28 °C) (Fig. S1). By 10 November, the temperature had decreased to 8 °C in Washademoak, and 6 °C in Harvey, with a brief warm period elevating the temperature/decreasing cooling around 8 October.
Potential for cyanotoxin production: PCR detection of mcyE and anaC sequences
More than 70% (34/48) of the surface water samples from Harvey and Washademoak lakes were PCR positive for the presence of the mcyE gene, with positive samples obtained from the beginning of the sampling period in late July through late October. No mcyE-positive samples were detected in November. Interestingly, the mcyE assay was negative for Washademoak site B on 30 July, when an intense cyanobacterial bloom was observed. All mcyE-positive samples were also positive for the Microcystis-specific mcyE primers. Only one sample (Humphrey’s Wharf (site B), Washademoak Lake) was positive for mcyE amplification using the Planktothrix-specific primers, and all evaluated samples were negative for the Dolichospermum-specific mcyE priming sequences (summary of PCR results in Table 1).
Table 1.
Date | |||||||||
---|---|---|---|---|---|---|---|---|---|
Site | PCR Assay | 30.07 | 13.08 | 27.08 | 11.09 | 25.09 | 08.10 | 23.10 | 10.11 |
Harvey Lake | |||||||||
A | mcyE | + | − | − | + | + | + | + | − |
Mic/Dol/Plank | +/−/− | +/−/− | +/−/− | +/−/− | +/−/− | ||||
anaC | + | + | + | + | + | + | − | − | |
B | mcyE | + | + | − | + | + | + | + | − |
Mic/Dol/Plank | +/−/− | +/−/− | +/−/− | +/−/− | +/−/− | +/−/− | |||
anaC | − | − | − | − | − | − | − | − | |
C | mcyE | + | + | + | + | + | + | + | − |
Mic/Dol/Plank | +/−/− | +/−/− | +/−/− | +/−/− | +/−/− | +/−/− | +/−/− | ||
anaC | + | − | − | − | + | − | − | − | |
Washademoak Lake | |||||||||
A | mcyE | + | + | + | + | − | + | + | − |
Mic/Dol/Plank | +/−/− | +/−/− | +/−/− | +/−/− | +/−/− | +/−/− | |||
anaC | + | + | − | + | + | − | − | − | |
B | mcyE | − | + | + | + | + | + | − | − |
Mic/Dol/Plank | +/−/− | +/−/− | +/−/− | +/−/+ | +/−/− | ||||
anaC | − | − | − | − | + | + | − | − | |
C | mcyE | + | − | − | + | + | + | + | − |
Mic/Dol/Plank | +/−/− | +/−/− | +/−/− | +/−/− | +/−/− | ||||
anaC | + | − | − | − | + | + | + | − |
PCR screening for the presence of the anaC gene resulted in positives for 18 of the 48 surface water samples from Harvey and Washademoak Lakes (Table 1) collected throughout the summer and fall. No samples tested positive in November.
Samples that tested negative for the mcyE and anaC gene were assayed with a universal primer pair (27F1 and 1492Rl; Table S2) that amplifies the bacterial 16S rRNA gene to determine if the negative results were due to PCR inhibition. All tested samples were positive for 16S rRNA amplicons.
The bacterial community of Washademoak and Harvey lakes
The Illumina reads produced from the last sampling date (10 November 2018) from both lakes were discarded because of low quality. Taxonomic binning of the raw reads with MG-RAST indicates that the bacterial communities in samples collected in Washademoak and Harvey lakes were mainly composed of Proteobacteria, Cyanobacteria, and Actinobacteria, which together account for at least 71% of all recovered major taxonomic groups in any DNA sample (Fig. 2a).
Fig. 2.

Cyanobacteria dominated in Washademoak Lake on 30 July and 27 August, where their relative abundance was more than 88%. This coincides with peak surface water temperatures (29–31 °C), and an intense cyanobacterial bloom that was visible on 30 July at this location. Bacteroidetes, Firmicutes, Verrucomicrobia, Planctomycetes, Chloroflexi, and Acidobacteria were other bacterial lineages present, but their accumulated relative abundance was not higher than 19% and 26% in Washademoak and Harvey lakes, respectively (Fig. 2a).
The MG-RAST analysis also revealed that members of the Synechococcus, Cyanothece, Microcystis, Nostoc, and Dolichospermum (planktonic Anabaena species have been transferred to the genus Dolichospermum (Wacklin et al. 2009)) genera were the more abundant cyanobacteria in both lakes (65%–79% in Washademoak and 65%–68% in Harvey) (Fig. 2b). Well-known bloom-forming genera (Microcystis, Nostoc, and Dolichospermum) were more prevalent in Washademoak with higher relative abundances (>45% in 4 samples) than in Harvey Lake (not higher than 29% in any sample) (Fig. 2b). In particular, the Washademoak Lake sample collected on 30 July was dominated by Dolichospermum and Nostoc, accounting for circa 70% of the total cyanobacterial abundance; yet, the PCR assays indicate there was no microcystin potential. Microcystis was relatively abundant in the same lake from August through October, peaking on 27 August (>35%) and 8 October (>25%), which coincides with the general and Microcystis-specific PCR assays indicating microcystin production potential from August through October.
The genome of Microcystis aeruginosa WS75, a toxin-producing cyanobacterium from Washademoak Lake
Our assembly and binning strategy recovered three good quality MAGs from Washademoak Lake, but it failed to identify assemblies of comparable quality in Harvey Lake samples. The three MAGs assembled from Washademoak Lake DNA samples (from 27 August and 8 October 2018) revealed a good degree of completeness (from 90% to 95%) and low levels of sequence contamination (from 0.5% to 4.5%). The phylogenetic analysis of a concatenated alignment of 49 core genes (Clusters of Orthologous Groups families preselected by KBase) shows that two of three Washademoak Lake MAGs belong to cyanobacteria of the genus Microcystis, whereas the third recovered genome is a β-proteobacteria of the Order Burkholderiales (Fig. 3).
Fig. 3.

The additional scaffolding steps allowed us to assemble a nearly complete cyanobacterial genome from the 27 August sample (hereafter referred as Microcystis aeruginosa WS75) (Table S3) highly similar (ANI > 97.4%) to Microcystis aeruginosa strains isolated from lakes Champlain and Caron and the reservoir Choinière in Quebec, Canada (Pérez-Carrascal et al. 2019) (Fig. S2), suggesting a close phylogenetic relationship between these lineages from New Brunswick and Quebec (Lee et al. 2016; Jain et al. 2018). The sequence identity of M. aeruginosa WS75 to genomes of other Microcystis species (e.g., M. flos-aquae TF09 and M. wesenbergii TW10) is circa 95% ANI (Fig. S2).
Regardless of differences in size and total number of genes identified, the G+C content and number of CRISPR arrays detected in the M. aeruginosa WS75 assembly (circa 4.9 Mbp) are comparable to those from M. aeruginosa NIES-2549 and M. aeruginosa NIES-843 (Kaneko et al. 2007; Yamaguchi et al. 2015) (Table 2). The repertoire of RNA-coding genes (rRNAs and tRNAs) of M. aeruginosa WS75 is identical to the NIES-2549 strain. In contrast, only 65%–71% of the 4914 predicted genes in M. aeruginosa WS75 are shared with both NIES-843 (3504 shared) and NIES-2549 (3209 shared). The reciprocal ortholog detection also revealed that M. aeruginosa WS75 contains a set of 765 exclusive genes not shared with the other two investigated M. aeruginosa genomes.
Table 2.
Features | M. aeruginosa WS75 (this study) | NIES-2549 | NIES-843 |
---|---|---|---|
Genome size (pb) | 4,891,847 | 4,301,200 | 5,842,795 |
G+C content (%) | 42.4 | 42.92 | 42.3 |
Total genes | 4,914 | 4,046 | 5,680 |
RNA genes | 51 | 51 | 52 |
CRISPR arrays | 3 | 4 | 3 |
The genome-mining survey detected 29 BGCs in M. aeruginosa WS75 accounting for more than 5% of the total assembled genomic sequences of this cyanobacterium (Table S4). According to DeepBGC, a “product activity” label was assigned to 20 of the clusters, whereas only 13 were assigned to a “product class” (Table S4). Although the antiSMASH survey indicates that most of these BGCs have no evident homologous counterparts in the MIBiG repository, 11 of them are similar to known clusters available in this database. These include complete, or near complete, BGCs involved in the biosynthesis of piricyclamides (5.7 Kbp, 91% of the BGC in M. aeruginosa PCC 7005; Fig. S3), the trypsin inhibitor aeruginosin 98- A,B,C (21.5 Kbp, 78% of the BGC in M. aeruginosa NIES-98; Fig. S4), micropeptin K139 (100% of the BGC in M. aeruginosa K-139)/cyanopeptolin (100% of the BGC in Microcystis sp. NIVA-CYA 172/5) (38 Kbp; Fig. S5) and, important to this work, the production of microcystin (60 Kpb; Fig. 4a). The BGC predicted with cytotoxic activity (hereafter WS75 MCY BGC) was very similar in order and orientation to the microcystin BGC of M. aeruginosa PCC 7806 (82% of the BGC) (Tillett et al. 2000) (Fig. 4a). We did not identify evidence of an anatoxin-a BGC in M. aeruginosa WS75.
Fig. 4.

MCY biosynthetic gene clusters from incomplete genome assemblies
Our PCR analysis detected the mcyE gene in most DNA samples from both lakes, but besides the WS75 MCY BGC, no other contigs containing the complete MCY cluster were recovered from other samples. However, when contigs assembled with metaSPAdes were mapped against WS75 MCY cluster, fragments (contigs) of the MCY cluster were found in samples from 8 October (WS78), 13 August (WS74), 11 September (WS76), and 25 September (WS77), which matches with the PCR results (Fig. 4b). The contig mapping coverage against the WS75 MCY cluster varies from the almost complete reconstruction of the MCY BGC in samples WS78 and WS74 to the highly fragmented assemblies from WS76 and WS77 (Fig. 4b). Importantly, there is no evidence of the MCY BGC in the Washademoak Lake samples collected on 30 July and 23 October, which is consistent with the negative mcyE PCR results for those same dates (Table 1). No contigs from Harvey Lake mapped to WS75 MCY BGC. We did not find evidence of additional gene clusters associated with production of other cyanotoxins in the metagenomic contigs from both lakes.
Discussion
PCR detection of toxin-producing cyanobacteria
The PCR screening indicates that during the 2018 sampling period, the potential for microcystin production, as inferred from the presence of the mcyE gene, was present throughout the summer and early fall of the year in both Washademoak and Harvey lakes. The use of different pairs of genus-specific mcyE primers allowed us to preliminarily identify Microcystis as the main contributor to the latent production of microcystin in these lakes. Despite the high relative abundance of Dolichospermum sequences in both lakes, which has been associated with microcystin production elsewhere (Huisman et al. 2018), no Dolichospermum-specific mcyE sequences were amplified by PCR. This result suggests that toxic genotypes of this taxa were either not present or below the limit of our PCR protocol during the period of study. The PCR survey also indicates that Planktothrix was a rare contributor to toxin production in certain locations. The primers used in our anaC PCR survey were designed to amplify sequences from diverse cyanobacterial genera (e.g., Anabaena, Aphanizomenon, Oscillatoria, Dolichospermum, and Microcoleus); therefore, we were unable to distinguish the particular cyanobacterial taxa associated with anatoxin-a potential. Recent reports have identified anatoxin-a, likely produced by Microcoleus, as a threat for human and animal health in Wolastoq/Saint John River in New Brunswick (McCarron et al. 2019), and our results indicate that this potent neurotoxin is also a concern for lakes in the same region.
The PCR approach permitted the detection of microcystin toxin potential in Harvey Lake, which has had blooms in the past but did not during this study period. This highlights the sensitivity of this approach and its ability to identify lakes at risk for toxic blooms, even under nonblooming conditions. As the frequency and severity of cyanobacterial blooms is anticipated to increase with climate change and cultural eutrophication (Huisman et al. 2018), the sensitivity of PCR provides an early-warning indicator for studying how lakes such as Harvey Lake, which have no or sporadic toxic blooms now, may transition to having more severe or frequent toxic blooms in the future.
The microbial community composition of Washademoak and Harvey lakes
The microbial community in both lakes was dominated by Cyanobacteria, Proteobacteria, Actinobacteria, Bacteroidetes, and Verrucomicrobia, which is consistent with previous metagenomic surveys in other lacustrine environments (Eiler and Bertilsson 2004; Pope and Patel 2008; Steffen et al. 2012). Unlike Harvey Lake, typical bloom-forming cyanobacteria (Microcystis, Dolichospermum, and Nostoc) dominated in Washademoak Lake during 2018, and indeed, a surface cyanobacterial bloom was present during the July sampling period. Dominance by bloom-forming cyanobacteria is typically associated with specific environmental conditions (Zohary and Breen 1989; de J. Magalhães et al. 2019). For example, Microcystis presence is commonly correlated with high retention times in the water column, low water transparency, and more stable and prolonged periods of thermal stratification (Soares et al. 2009; Rangel et al. 2016; Guedes et al. 2018), while being more sensitive to mixing and turbulence (Reynolds 2006). Total cyanobacterial biomass is also positively correlated with water temperature and nutrient concentration (i.e., phosphorus) (Beaulieu et al. 2013; Smith 2019). Certainly, the surface water temperatures in Washademoak Lake reached a higher maximum than in Harvey Lake in 2018 (31 °C vs 28 °C) (Fig. S1), and Washademoak Lake is mesotrophic whereas Harvey Lake is oligotrophic (New Brunswick Department of Environment and Local Government 2019).
The genome of Microcystis aeruginosa WS75 from Washademoak Lake and its potential to produce bioactive metabolites
We assembled a near-complete genome of a toxigenic Microcystis aeruginosa strain inhabiting Washademoak Lake. The difference in genome size and presence of unique genes in M. aeruginosa WS75 relative to other strains of the same species (i.e., NIES-843 and NIES-2549) can be explained, in part, not only by disparities in the number of genes involved in DNA replication, recombination, and repair (Yamaguchi et al. 2018), but also by the high number of taxon-specific genes likely involved in the biosynthesis of toxins and other secondary metabolites, which is an important characteristic of this cyanobacterial lineage (Humbert et al. 2013; Huang and Zimba 2019). The high sequence identity at the genomic level between M. aeruginosa WS75 and M. aeruginosa strains characterized in fresh water bodies in Quebec strongly suggests these isolates are part of the same locally adapted lineage of the globally distributed M. aeruginosa (Pérez-Carrascal et al. 2019).
The recovery of MAGs from environmental samples has opened the possibility of screening for new genes and gene clusters, unlocking the previously underestimated metabolic potential of bacterial communities from lakes and other aquatic systems (McMahon 2015; Cuadrat et al. 2018). Metagenomics has been particularly useful for studying potentially toxic bloom-forming cyanobacteria, avoiding the challenges and limitations of culture-based studies (Alvarenga et al. 2017; Semedo-Aguiar et al. 2018; Pérez-Carrascal et al. 2019). Additionally, genome-scale investigations of bloom-forming and toxin-producing cyanobacteria have been key to appreciating their genetic diversity and capabilities (Pérez-Carrascal et al. 2019), genome plasticity (Meyer et al. 2017), and to improve our capacity to manage freshwater ecosystems during blooming events (Yamaguchi et al. 2020).
Our genome mining analysis revealed a high potential for M. aeruginosa WS75 to produce diverse secondary metabolites. That 5% of the M. aeruginosa WS75 genome involved in the production of secondary metabolites is consistent with previous observations that suggest cyanobacteria dedicate between 5% and 6% of their coding capacity to produce secondary metabolites, with some genomes encoding up to 23 BGCs (Shih et al. 2013; Calteau et al. 2014). Identifying the MYC BGC in the M. aeruginosa WS75 genome is not surprising given that members of this genus are recognized as producers of microcystins (Pineda-Mendoza et al. 2016), but contributes to understanding the genomic footprint of this toxic genus in the Atlantic region of Canada. The MYC BGC of M. aeruginosa WS75 lacks the uma5 and uma6 genes, but the uma coding regions (uma1 to 6) have been reported in genomes of both toxic and nontoxic strains and are likely not involved in the biosynthesis of microcystins (Tillett et al. 2000). Consistent with the PCR survey, we found evidence of fragmented MYC BGCs in all PCR-positive samples from Washademoak Lake.
In addition to the MYC BGC, we also identified diverse gene sets associated with the production of other bioactive secondary metabolites that have not received the same attention as microcystins (Pearson et al. 2019). Cyanobacteria, and species of Microcystis in particular, are known to produce potentially bioactive peptides that are underexplored with respect to their relevance in human health and ecological effects (Janssen 2019). The M. aeruginosa WS75 biosynthetic repertoire includes the ability to produce aeruginosin, which is a potent inhibitor of serine proteases such as thrombin (involved in the human blood coagulation enzymatic cascade) and trypsin (part of the digestive enzymatic battery) (Ersmark et al. 2008; Pearson et al. 2019), with some variants being highly toxic to invertebrates (Scherer et al. 2016). We also identified BCGs involved in the production of cyanopeptolins and micropeptins (CTPs), which can act as inhibitors of the coagulation Factor Xla, human kallikrein, plasmin, thrombin, trypsin, and chymotrypsin. Due to their protease inhibition activity, CTPs cause high mortality rates in freshwater water flea (Daphnia sp.) and fairy shrimp (Thamnocephalus platyurus) (Huang and Zimba 2019).
Secondary metabolites also play important roles in microbial community dynamics and are thought to be produced in response to abiotic and biotic stress, thus providing advantages to the holder over other organisms coexisting in the same environment (Kultschar and Llewellyn 2018). For example, microcystin produced during a Microcystis bloom has an allelopathic effect, inhibiting the growth of Synechococcus elongatus (Hu et al. 2004). In general, bioactive metabolites can modify the abiotic and biotic conditions of aquatic environments to favor dominance of bloom-forming cyanobacteria (Kurmayer et al. 2016).
Our metagenomic analysis failed to recover a MAG from Harvey Lake or genome fragments coding for the anatoxin-a BGC in either lake, despite the anaC gene being detected by PCR analysis. In contrast to PCR detection, shotgun sequencing of environmental DNA is typically biased towards the dominant organisms in each sample, with low probabilities of detecting rare members of the community (Bharagava et al. 2019). Accordingly, abundance of anatoxin-a-producing genotypes in both lakes is expected to be low, and microcystin producing cyanobacteria would not be dominant in Harvey Lake.
From detection to comparative metagenomics of toxin production cyanobacteria in Washademoak and Harvey lakes
PCR-based surveys targeting specific genes involved in toxin biosynthesis are widely used, rapid, and a cost-effective method for qualitative (conventional PCR) and (or) quantitative (qPCR) identification of toxic cyanobacterial genotypes (Dittmann et al. 2017; Rantala-Ylinen et al. 2017). The sensitivity of PCR enables the detection of individual cells even before a cyanobacterial bloom occurs, providing time to initiate appropriate managements actions such as public warnings and to employ more costly chemical analyses for identification and quantification of cyanotoxins (Kurmayer et al. 2017).
The sensitivity of PCR allowed us to identify, but with limited taxonomic precision, the genetic potential for microcystin and anatoxin-a production in two New Brunswick lakes that have experienced recurrent cyanobacterial blooms. This rapid and effective approach requires limited expertise to undertake, making it an accessible tool for other understudied regions where toxin potential is not well-characterized. Findings can then be used to advocate for more comprehensive monitoring programs and employing quantitative approaches, such as qPCR for gene abundance and chemical analyses for toxin concentration. In addition, the PCR results allowed us to direct our metagenomic survey to recover the almost complete genome of the microcystin-producing cyanobacteria inhabiting Washademoak Lake. Our analyses revealed that M. aeruginosa WS75 from Washademoak Lake belongs to a lineage with toxigenic potential widely distributed in other lakes across eastern Canada.
Detailed characterization of cyanobacteria inhabiting Atlantic Canada freshwater systems is important for a region that is relatively understudied and becoming increasingly impacted by cyanobacterial blooms. Although our PCR-based survey was targeted to genes involved in microcystin and anatoxin biosynthesis and the metagenomic approach did not recover BGCs for the production of other cyanotoxins, investigations into the cyanobacterial genetic potential to produce other, but less common, toxic secondary metabolites (e.g., cylindrospermopsins and saxitoxins) should be explored in freshwater systems of Atlantic Canada.
We hope that surveys combining PCR and metagenomic approaches, which also are becoming a relatively low-cost survey option, are regularly employed to explore the toxin and other bioactive secondary metabolite potential of cyanobacteria communities in other understudied regions.
References
Al-Tebrineh J, Merrick C, Ryan D, Humpage A, Bowling L, and Neilan BA. 2012. Community composition, toxigenicity, and environmental conditions during a cyanobacterial bloom occurring along 1,100 kilometers of the Murray River. Applied and Environmental Microbiology, 78(1): 263–272.
Alvarenga DO, Fiore MF, and Varani AM. 2017. A metagenomic approach to cyanobacterial genomics. Frontiers in Microbiology, 8: 809.
Arkin AP, Cottingham RW, Henry CS, Harris NL, Stevens RL, Maslov S, et al. 2018. KBase: The United States department of energy systems biology knowledgebase. Nature Biotechnology, 36: 566–569.
Baker JA, Neilan BA, and McKay DB. 2002. Monitoring changing toxigenicity of a cyanobacterial bloom by molecular methods. Applied and Environmental Microbiology 68: 6070–6076.
Bankevich A, Nurk S, Antipov D, Gurevich AA, Dvorkin M, Kulikov AS, et al. 2012. SPAdes: A new genome assembly algorithm and its applications to single-cell sequencing. Journal of Computational Biology, 19(5): 455–477.
Beaulieu M, Pick F, and Gregory-Eaves I. 2013. Nutrients and water temperature are significant predictors of cyanobacterial biomass in a 1147 lakes data set. Limnology and Oceanography, 58: 1736–1746.
Bharagava RN, Purchase D, Saxena G, and Mulla SI. 2019. Chapter 26 – applications of metagenomics in microbial bioremediation of pollutants: From genomics to environmental cleanup. In: Microbial diversity in the genomic era. Edited by S Das, HR Dash. Academic Press. pp. 459–477.
Blin K, Shaw S, Steinke K, Villebro R, Ziemert N, Lee SY, et al. 2019. antiSMASH 5.0: updates to the secondary metabolite genome mining pipeline. Nucleic Acids Research, 47(W1): W81–W87.
Calteau A, Fewer DP, Latifi A, Coursin T, Laurent T, Jokela J, et al. 2014. Phylum-wide comparative genomics unravel the diversity of secondary metabolism in cyanobacteria. BMC Genomics, 15(1): 977.
Cepeda V, Liu B, Almeida M, Hill C, Koren S, Treangen T, et al. 2017. MetaCompass: reference-guided assembly of metagenomes. bioRxiv 212506.
Chen L-X, Anantharaman K, Shaiber A, Eren AM, and Banfield JF. 2020. Accurate and complete genomes from metagenomes. Genome Research, 30(3): 315–333.
Chorus I, and Welker M. 2021. Toxic cyanobacteria in water: A guide to their public health consequences, monitoring and management 2nd ed. CRC Press.
Codd GA, Lindsay J, Young FM, Morrison LF, and Metcalf JS. 2005. Harmful cyanobacteria. Springer Netherlands, Dordrecht. 23 p.
Contreras-Moreira B, and Vinuesa P. 2013. GET_HOMOLOGUES, a versatile software package for scalable and robust microbial pangenome analysis. Applied and Environmental Microbiology, 79(24): 7696–7701.
Cuadrat RRC, Ionescu D, Dávila AMR, and Grossart H-P. 2018. Recovering genomics clusters of secondary metabolites from lakes using genome-resolved metagenomics. Frontiers in Microbiology, 9: 251.
Dittmann E, Gugger M, Sivonen K, and Fewer DP. 2015. Natural product biosynthetic diversity and comparative genomics of the cyanobacteria. Trends in Microbiology, 23(10): 642–652.
Dittmann E, Rantala-Ylinen A, Sivonen K, Gągała I, Mankiewicz-Boczek J, Cirés S, et al. 2017. Conventional PCR. In Molecular tools for the detection and quantification of toxigenic cyanobacteria. Edited by R Kurmayer, K Sivonen, A Wilmotte, and N Salmaso. John Wiley & Sons, Ltd. pp. 163–203.
Doblin MA, Coyne KJ, Rinta-Kanto JM, Wilhelm SW, and Dobbs FC. 2007. Dynamics and short-term survival of toxic cyanobacteria species in ballast water from NOBOB vessels transiting the Great Lakes-implications for HAB invasions. Harmful Algae, 6(4): 519–530.
Eiler A, and Bertilsson S. 2004. Composition of freshwater bacterial communities associated with cyanobacterial blooms in four Swedish lakes. Environmental Microbiology, 6(12): 1228–1243.
Ersmark K, Del Valle JR, and Hanessian S. 2008. Chemistry and biology of the aeruginosin family of serine protease inhibitors. Angewandte Chemie International Edition, 47: 1202–1223.
Frioux C, Singh D, Korcsmaros T, and Hildebrand F. 2020. From bag-of-genes to bag-of-genomes: metabolic modelling of communities in the era of metagenome-assembled genomes. Computational and Structural Biotechnology Journal, 18: 1722–1734.
Guedes IA, Rachid CTCC, Rangel LM, Silva LHS, Bisch PM, Azevedo SMFO, et al. 2018. Close link between harmful cyanobacterial dominance and associated bacterioplankton in a tropical eutrophic reservoir. Frontiers in Microbiology, 9: 424.
Guizelini D, Raittz RT, Cruz LM, Souza EM, Steffens MBR, and Pedrosa FO. 2016. GFinisher: a new strategy to refine and finish bacterial genome assemblies. Scientific Reports, 6: 1–8.
Hannigan GD, Prihoda D, Palicka A, Soukup J, Klempir O, Rampula L, et al. 2019. A deep learning genome-mining strategy for biosynthetic gene cluster prediction. Nucleic Acids Research, 47(18): e110.
Hu Z, Liu Y, and Li D. 2004. Physiological and biochemical analyses of microcystin-RR toxicity to the cyanobacterium Synechococcus elongatus. Environmental Toxicology, 19(6): 571–577.
Huang I-S, and Zimba P V. 2019. Cyanobacterial bioactive metabolites – A review of their chemistry and biology. Harmful Algae, 83: 42–94.
Huisman J, Codd GA, Paerl HW, Ibelings BW, Verspagen JMH, and Visser PM. 2018. Cyanobacterial blooms. Nature Reviews Microbiology, 16: 471–483.
Humbert J-F, Barbe V, Latifi A, Gugger M, Calteau A, Coursin T, et al. 2013. A tribute to disorder in the genome of the bloom-forming freshwater cyanobacterium Microcystis aeruginosa. PLoS ONE, 8(8): e70747.
Humbert JF, Quiblier C, and Gugger M. 2010. Molecular approaches for monitoring potentially toxic marine and freshwater phytoplankton species. Analytical and Bioanalytical Chemistry, 397: 1723–1732.
Jain C, Rodriguez-R LM, Phillippy AM, Konstantinidis KT, and Aluru S. 2018. High throughput ANI analysis of 90K prokaryotic genomes reveals clear species boundaries. Nature Communications, 9: 5114.
Janssen EM-L. 2019. Cyanobacterial peptides beyond microcystins–A review on co-occurrence, toxicity, and challenges for risk assessment. Water Research, 151: 488–499.
Kaneko T, Nakajima N, Okamoto S, Suzuki I, Tanabe Y, Tamaoki M, et al. 2007. Complete genomic structure of the bloom-forming toxic cyanobacterium Microcystis aeruginosa NIES-843. DNA Research, 14(6): 247–256.
Kang DD, Froula J, Egan R, and Wang Z. 2015. MetaBAT, an efficient tool for accurately reconstructing single genomes from complex microbial communities. PeerJ, 3: e1165.
Kautsar SA, Blin K, Shaw S, Navarro-Muñoz JC, Terlouw BR, van der Hooft JJJ, et al. 2019. MIBiG 2.0: a repository for biosynthetic gene clusters of known function. Nucleic Acids Research, 48(D1): D454–D458.
Kent WJ. 2002. BLAT--the BLAST-like alignment tool. Genome Research, 12(4):, 656–664.
Kultschar B, and Llewellyn C. 2018. Secondary metabolites in cyanobacteria. In Secondary metabolites-sources and applications, IntechOpen, London, UK. pp. 23–36.
Kurmayer R, Deng L, and Entfellner E. 2016. Role of toxic and bioactive secondary metabolites in colonization and bloom formation by filamentous cyanobacteria Planktothrix. Harmful Algae, 54: 69–86.
Kurmayer R, Sivonen K, and Salmaso N. 2017. Introduction. In Molecular tools for the detection and quantification of toxigenic cyanobacteria. Edited by R Kurmayer, K Sivonen, A Wilmotte and N Salmaso.
Lane DJ. 1991. 16S/23S rRNA sequencing. Nucleic acid techniques in bacterial systematics. John Wiley and Sons, New York. pp. 115–175.
Lee I, Ouk Kim Y, Park S-C, and Chun J. 2016. OrthoANI: An improved algorithm and software for calculating average nucleotide identity. International Journal of Systematic and Evolutionary Microbiology, 66(2): 1100–1103.
Li D, Liu C-M, Luo R, Sadakane K, and Lam T-W. 2015. MEGAHIT: An ultra-fast single-node solution for large and complex metagenomics assembly via succinct de Bruijn graph. Bioinformatics, 31(10): 1674–1676.
Li H. 2013. Aligning sequence reads, clone sequences and assembly contigs with BWA-MEM. ArXiv 1303. [online]: Available from arxiv.org/abs/1303.3997.
Li L, Stoeckert CJJ, and Roos DS. 2003. OrthoMCL: identification of ortholog groups for eukaryotic genomes. Genome Research, 13(9): 2178–2189.
Magalhães de AA Jr, da Luz LD, and de Aguiar TR. Jr 2019. Environmental factors driving the dominance of the harmful bloom-forming cyanobacteria Microcystis and Aphanocapsa in a tropical water supply reservoir. Water Environment Research, 91: 1466–1478.
McCarron P, Deacoff C, Krkosek W, and Beach D. 2019. Atlantic Canadian Cyanobacterial Workshop 2018: workshop final report. In Atlantic Canadian Cyanobacterial Workshop 2018, Halifax, Nova Scotia, Canada.
McMahon K. 2015. Metagenomics 2.0. Environmental Microbiology Reports, 7(1): 38–39.
Meyer F, Paarmann D, D’Souza M, Olson R, Glass EM, Kubal M, et al. 2008. The metagenomics RAST server – A public resource for the automatic phylogenetic and functional analysis of metagenomes. BMC Bioinformatics, 9: 386.
Meyer KA, Davis TW, Watson SB, Denef VJ, Berry MA, and Dick GJ. 2017. Genome sequences of lower Great Lakes Microcystis sp. reveal strain-specific genes that are present and expressed in western Lake Erie blooms. PLoS ONE, 12(10): e0183859.
Micallef ML, D’Agostino PM, Sharma D, Viswanathan R, and Moffitt MC. 2015. Genome mining for natural product biosynthetic gene clusters in the Subsection V cyanobacteria. BMC Genomics, 16: 669.
New Brunswick Department of Environment and Local Government. 2019. The state of water quality in New Brunswick’s lakes and rivers: water quality monitoring results 2003–2016. [online]: Available from: www2.gnb.ca/content/dam/gnb/Departments/env/pdf/Water-Eau/TheStateOfWaterQualityInNBLakesRivers.pdf.
Orihel DM, Bird DF, Brylinsky M, Chen H, Donald DB, Huang DY, et al. 2012. High microcystin concentrations occur only at low nitrogen-to-phosphorus ratios in nutrient-rich Canadian lakes. Canadian Journal of Fisheries and Aquatic Sciences, 69(9): 1457–1462.
Parks DH, Imelfort M, Skennerton CT, Hugenholtz P, and Tyson GW. 2015. CheckM: assessing the quality of microbial genomes recovered from isolates, single cells, and metagenomes. Genome Research, 25(7): 1043–1055.
Pearson LA, Crosbie ND, and Neilan BA. 2019. Distribution and conservation of known secondary metabolite biosynthesis gene clusters in the genomes of geographically diverse Microcystis aeruginosa strains. Marine and Freshwater Research, 71(5): 701–716.
Pérez-Carrascal OM, Terrat Y, Giani A, Fortin N, Greer CW, Tromas N, et al. 2019. Coherence of Microcystis species revealed through population genomics. The ISME Journal, 13: 2887–2900.
Pick FR. 2016. Blooming algae: A Canadian perspective on the rise of toxic cyanobacteria. Canadian Journal of Fisheries and Aquatic Sciences, 73(7): 1149–1158.
Pineda-Mendoza RM, Zúñiga G, and Martínez-Jerónimo F. 2016. Microcystin production in Microcystis aeruginosa: Effect of type of strain, environmental factors, nutrient concentrations, and N:P ratio on mcyA gene expression. Aquatic Ecology, 50(1): 103–119.
Pope PB, and Patel BKC. 2008. Metagenomic analysis of a freshwater toxic cyanobacteria bloom. FEMS Microbiology Ecology, 64(1): 9–27.
Price MN, Dehal PS, and Arkin AP. 2010. FastTree 2—Approximately maximum-likelihood trees for large alignments. PLoS ONE, 5(3): e9490.
Rangel LM, Soares MCS, Paiva R, and Silva LHS. 2016. Morphology-based functional groups as effective indicators of phytoplankton dynamics in a tropical cyanobacteria-dominated transitional river–reservoir system. Ecological Indicators, 64: 217–227.
Rantala A, Fewer DP, Hisbergues M, Rouhiainen L, Vaitomaa J, Börner T, et al. 2004. Phylogenetic evidence for the early evolution of microcystin synthesis. Proceedings of the National Academy of Sciences, 101: 568–573.
Rantala A, Rajaniemi-Wacklin P, Lyra C, Lepistö L, Rintala J, Mankiewicz-Boczek J, et al. 2006. Detection of microcystin-producing cyanobacteria in Finnish Lakes with genus-specific microcystin synthetase gene E (mcyE) PCR and associations with environmental factors. Applied and Environmental Microbiology, 72(9): 6101–6110.
Rantala-Ylinen A, Känä S, Wang H, Rouhiainen L, Wahlsten M, Rizzi E, et al. 2011. Anatoxin-a synthetase gene cluster of the cyanobacterium Anabaena sp. strain 37 and molecular methods to detect potential producers. Applied and Environmental Microbiology, 77(20): 7271–7278.
Rantala-Ylinen A, Savela H, Sivonen K, and Kurmayer R. 2017. Quantitative PCR. In Molecular tools for the detection and quantification of toxigenic cyanobacteria. Edited by R Kurmayer, K Sivonen, A Wilmotte, and N Salmaso.
Reynolds CS. 2006. The ecology of phytoplankton (Ecology, biodiversity and conservation). Cambridge University Press, Cambridge.
Scherer M, Bezold D, and Gademann K. 2016. Investigating the toxicity of the aeruginosin chlorosulfopeptides by chemical synthesis. Angewandte Chemie International Edition, 55(32): 9427–9431.
Semedo-Aguiar AP, Pereira-Leal JB, and Leite RB. 2018. Microbial diversity and toxin risk in tropical freshwater reservoirs of Cape Verde. Toxins, 10(5): 186.
Shih PM, Wu D, Latifi A, Axen SD, Fewer DP, Talla E, et al. 2013. Improving the coverage of the cyanobacterial phylum using diversity-driven genome sequencing. Proceedings of the National Academy of Sciences, 110(3): 1053–1058.
Sieber CMK, Probst AJ, Sharrar A, Thomas BC, Hess M, Tringe SG, et al. 2018. Recovery of genomes from metagenomes via a dereplication, aggregation and scoring strategy. Nature Microbiology, 3: 836–843.
Smith R. 2019. Monitoring water bodies with harmful algal blooms: a Canadian landscape scan. Our Living Waters, Canada.
Soares M, Rocha MI, Marinho M, Azevedo S, Branco CWC, and Huszar V. 2009. Changes in species composition during annual cyanobacterial dominance in a tropical reservoir: Physical factors, nutrients and grazing effects. Aquatic Microbial Ecology, 57(2): 137–149.
Steffen MM, Li Z, Effler TC, Hauser LJ, Boyer GL, and Wilhelm SW. 2012. Comparative metagenomics of toxic freshwater cyanobacteria bloom communities on two continents. PLoS ONE, 7(8): e44002.
Tamames J, and Puente-Sánchez F. 2019. SqueezeMeta, a highly portable, fully automatic metagenomic analysis pipeline. Frontiers in Microbiology, 9: 3349.
Tatusova T, DiCuccio M, Badretdin A, Chetvernin V, Nawrocki EP, Zaslavsky L, et al. 2016. NCBI prokaryotic genome annotation pipeline. Nucleic Acids Research, 44(14): 6614–6624.
Tillett D, Dittmann E, Erhard M, von Döhren H, Börner T, and Neilan BA. 2000. Structural organization of microcystin biosynthesis in Microcystis aeruginosa PCC7806: an integrated peptide-polyketide synthetase system. Chemistry & Biology, 7(10): 753–764.
Vaitomaa J, Rantala A, Halinen K, Rouhiainen L, Tallberg P, Mokelke L, et al. 2003. Quantitative real-time PCR for determination of microcystin synthetase e copy numbers for Microcystis and Anabaena in lakes. Applied and Environmental Microbiology, 69(12): 7289–7297.
Vezie C, Brient L, Sivonen K, Bertru G, Lefeuvre JC, and Salkinoja-Salonen M. 1998. Variation of microcystin content of cyanobacterial blooms and isolated strains in Lake Grand-Lieu (France). Microbial Ecology, 35(2): 126–135.
Wacklin P, Hoffmann L, and Komarek J. 2009. Nomenclatural validation of the genetically revised cyanobacterial genus Dolichospermum (RALFS ex BORNET et FLAHAULT) comb. nova. Fottea, 9: 59–64.
Weller DI. 2011. Detection, identification and toxigenicity of cyanobacteria in New Zealand lakes using PCR-based methods. New Zealand Journal of Marine and Freshwater Research, 45(4): 651–664.
Wences AH, and Schatz MC. 2015. Metassembler: merging and optimizing de novo genome assemblies. Genome Biology, 16: 207.
Wilke A, Harrison T, Wilkening J, Field D, Glass EM, Kyrpides N, et al. 2012. The M5nr: a novel non-redundant database containing protein sequences and annotations from multiple sources and associated tools. BMC Bioinformatics, 13: 141.
Wu Y-W, Simmons BA, and Singer SW. 2015. MaxBin 2.0: An automated binning algorithm to recover genomes from multiple metagenomic datasets. Bioinformatics, 32: 605–607.
Yamaguchi H, Suzuki S, Tanabe Y, Osana Y, Shimura Y, Ishida K-I, et al. 2015. Complete genome sequence of Microcystis aeruginosa NIES-2549, a bloom-forming cyanobacterium from Lake Kasumigaura, Japan. Genome Announcements, 3(3): e00551–15.
Yamaguchi H, Suzuki S, Osana Y, and Kawachi M. 2018. Complete genome sequence of Microcystis aeruginosa NIES-2481 and common genomic features of group G M. aeruginosa. Journal of Genomics, 6: 30–33.
Yamaguchi H, Suzuki S, Osana Y, and Kawachi M. 2020. Genomic characteristics of the toxic bloom-forming cyanobacterium Microcystis aeruginosa NIES-102. Journal of Genomics, 8: 1–6.
Zhang C, and Zhang J. 2015. Current techniques for detecting and monitoring algal toxins and causative harmful algal blooms. Journal of Environmental Analytical Chemistry, 2: 123.
Zohary T, and Breen CM. 1989. Environmental factors favouring the formation of Microcystis aeruginosa hyperscums in a hypertrophic lake. Hydrobiologia, 178: 179–192.
Supplementary material
Supplementary Material 1 (DOCX / 513 KB)
- Download
- 513.45 KB
Information & Authors
Information
Published In
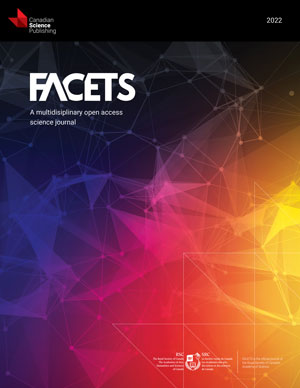
FACETS
Volume 7 • Number 1 • January 2022
Pages: 194 - 214
Editor: Kristi M. Miller
History
Received: 27 July 2021
Accepted: 2 November 2021
Version of record online: 17 February 2022
Copyright
© 2022 Valadez-Cano et al. This work is licensed under a Creative Commons Attribution 4.0 International License (CC BY 4.0), which permits unrestricted use, distribution, and reproduction in any medium, provided the original author(s) and source are credited.
Data Availability Statement
All relevant data are within the paper and in the Supplementary Material.
Key Words
Sections
Subjects
Plain Language Summary
Toxic bloom-forming cyanobacteria inhabit lakes in New Brunswick, Canada
Authors
Author Contributions
CV-C, AR-P, and JL conceived and designed the study.
KH, RC, and JL performed the experiments/collected the data.
CV-C, AR-P, and JL analyzed and interpreted the data.
AR-P and JL contributed resources.
CV-C, AR-P, and JL drafted or revised the manuscript.
Competing Interests
The authors have declared that no competing interests exist.
Metrics & Citations
Metrics
Other Metrics
Citations
Cite As
Cecilio Valadez-Cano, Kristen Hawkes, Rossella Calvaruso, Adrian Reyes-Prieto, and Janice Lawrence. 2022. Amplicon-based and metagenomic approaches provide insights into toxigenic potential in understudied Atlantic Canadian lakes. FACETS.
7: 194-214.
https://doi.org/10.1139/facets-2021-0109
Export Citations
If you have the appropriate software installed, you can download article citation data to the citation manager of your choice. Simply select your manager software from the list below and click Download.
Cited by
1. Newly isolated strains of potentially microcystin-producing cyanobacteria in potable water: case study of Mawoni village, South Africa
2. Characterization of Taxonomic and Functional Dynamics Associated with Harmful Algal Bloom Formation in Recreational Water Ecosystems
3. Sporadic diurnal fluctuations of cyanobacterial populations in oligotrophic temperate systems can prevent accurate characterization of change and risk in aquatic systems
4. Binning Metagenomic Contigs Using Unsupervised Clustering and Reference Databases
5. Using FlowCam and molecular techniques to assess the diversity of Cyanobacteria species in water used for food production
6. Sporadic Diurnal Fluctuations of Cyanobacterial Populations in Oligotrophic Temperate Systems Can Prevent Accurate Characterization of Change and Risk in Aquatic Systems