Perspective-based search: a new paradigm for bursting the information bubble
Abstract
Nowadays, individuals heavily rely on search engines for seeking information. The presence of information bubbles (filter bubbles and echo chambers) can threaten the effectiveness of these systems in providing unbiased information and damage healthy civic discourse and open-minded deliberation. In this paper, we propose a new paradigm for search that aims at mitigating the information bubble in the search. The paradigm, which we call perspective-based search (PBS), is based on the intuition that in a fair search the user should not be limited to the results corresponding to a specific perspective of the search topic. Briefly, in PBS, different perspectives of the search topic are identified and presented to the user and the user can select a perspective for the search results. In this paper, we focus on the paradigm itself, why it is an appropriate solution, and how it differs from other solutions. We raise new questions and call for research on the paradigm and on providing solutions for implementing its required components. We do not aim at providing any specific implementation for it, although we provide some hints on implementing it. We also provide a survey of the related concepts and methods and discuss their differences with PBS.
1. Introduction
Emersion of the Internet was associated with great hopes for more knowledgeable societies with healthier civic discourse and a borderless world with a free flow of information. However, two phenomena prevented this realization. The first phenomenon is filter bubbles, as coined by Pariser (2011), wherein user-tailored information systems, such as personalized recommender systems and personalized search engines, only show agreeable information to users. Pariser explained it in his book: “The basic code at the heart of the new Internet is pretty simple. The new generation of Internet filters looks at the things you seem to like—the actual things you’ve done, or the things people like you like—and tries to extrapolate. They are prediction engines, constantly creating and refining a theory of who you are and what you’ll do and want next. Together, these engines create a unique universe of information for each of us—what I’ve come to call a filter bubble—which fundamentally alters the way we encounter ideas and information” (Pariser 2011, p. 10). This phenomenon can lead to group polarization and disconnection from other points of view. In other words, territorial borders are substituted by filter borders that prevent the borderless utopia to be realized. The second phenomenon is what Sunstein (2001a, 2007) called echo chambers (or information cocoons). Due to the cognitive biases such as selective exposure (Sears and Freedman 1967; Frey 1986) and homophily (McPherson et al. 2001), which are inherent to the human nature, people have a natural tendency to prefer confirmatory information and avoid dissonant information. This tendency can put them in echo chambers wherein people are only exposed to agreeable information. Since echo chambers can be considered as self-imposed filter bubbles (Yom-Tov et al. 2014), we hereafter use the term information bubble to refer to both the filter bubble and the echo chamber. The information bubble can lead to several disadvantageous results, among them are:
–
“When people find themselves in groups of like-minded types, they are especially likely to move to extremes” (Sunstein 2009, p. 2). This can lead to more polarized views of topics.
–
It can increase intolerance to opposing views (Garrett and Resnick 2011).
–
Individuals may lose a common reference point, since they all see different information (Resnick 2011).
–
The society may fragment into polarized enclaves (Resnick 2011).
–
Users’ autonomies are threatened. The information system decides what users should and should not see. The users may not be aware of what the system thinks about them and may not even know the presence of such filters in the system (Pariser 2011; Eslami et al. 2015).
These consequences can damage healthy civic discourse and open-minded deliberation. In this paper, we propose a new paradigm for information retrieval (IR) to mitigate these problems. It is important to note that in this paper we focus on the paradigm itself and raise new research questions around it on the realms of the Semantic Web, information retrieval, text mining, etc. We call on researchers to investigate the paradigm and provide solutions for implementing its required components. Here, we do not aim at providing any specific implementation, although we will discuss some points on the implementation in Section 3. We also provide a survey of the related concepts and methods in Section 5.
Current IR paradigms are either nonpersonalized or personalized. In the nonpersonalized approach, a combination of search results from different perspectives are shown to the user, which could be confusing, and since users usually view only a few results, the user will probably see only a small portion of different perspectives on that topic without being aware of the other opinions and thoughts on it. In the personalized approach, this phenomenon can occur more severely. In other words, if the system categorizes the user to one of the perspectives according to the user information, such as geographical location, language, social network connections, and profile, it biases the results towards that perspective, and thus it is highly probable that the user remains unaware of the other perspectives.
1.1. Our proposal
The new paradigm, which we call perspective-based search (PBS), is based on the intuition that in a fair search the user should not be limited to the results corresponding to a specific perspective of the search topic and should be able to identify different perspectives on it and see the topic from those perspectives. As an example, suppose that a user is searching “2014 Crimean crisis”. There are different viewpoints towards the Crimean crisis. One is the US government’s viewpoint which is pro-Ukrainian. On the other hand, there is the Russian government’s viewpoint which is pro-Russian. Such apparent conflicts in perspectives are prevalent in many of the searches, and thus a natural way of dealing with these kinds of conflicts is required in search engines. PBS emphasizes alerting the user about the existence of such different viewpoints and gives the user control in choosing from which perspective they want to see the search results. It is noteworthy that in this paper a perspective is not limited to a political perspective and, loosely speaking, we consider a perspective as any point of view that affects the desired ranking of search results. The paradigm can also provide another benefit by enabling users to more easily formulate their information needs in some circumstances and obtain a higher recall. This benefit is explained more in Sections 2.1.1 and 2.1.2. It is important to note that in this paper we do not focus on technical details, instead we conceptualize PBS and provide arguments for supporting it from an analytical point of view, although some hints on its implementation are also provided. We also raise new research questions and call for research on them.
1.2. Research questions
In this paper, we address the following research questions:
1.
How can we mitigate the information bubble in search? Different approaches to mitigating the information bubble are possible. We introduce our suggested approach in Section 2.
2.
How can the proposed approach be implemented? Although we do not aim at providing any specific implementation for the proposed approach, we provide some hints on implementing it in Section 3.
3.
What are the advantages of the proposed approach? We analyze the strengths of the approach in Section 4. In Section 4, we also explain how it can be employed to promulgate worldviews and lifestyles.
4.
How is the proposed approach contrasted to the existing ones? In Section 5, we discuss the related work and compare the proposed approach with them.
1.3. Contributions
The main contributions of this paper can be summarized as follows.
1.
We propose the PBS paradigm for search that aims at mitigating the information bubble in search. We shed light on the problem of mitigating the information bubble and propose the paradigm as a solution for it, which addresses different aspects of the problem. There are already plenty of works aiming at the mitigation (see Section 5). However, PBS integrates many of them in a unified form. Thus, the main contribution of this paper is establishing a common ground for researchers to work on the problem of mitigating the information bubble and providing a unified form for delivering the results to users. From an analytical perspective, we provide arguments on why PBS is superior to the previous solutions, although experiments must be conducted in the future to support them.
2.
We provide several possible perspective types that can be integrated into PBS. Specifically, belief perspectives and social networks members perspectives are novel in the sense that, to the best of our knowledge, they have not been proposed previously, are not used for mitigating the information bubble in searches, or the way they are used does not provide the benefits achievable in PBS.
3.
We provide some hints on implementing PBS that relate it to different fields such as the Semantic Web, information retrieval, text mining, and journalism. These hints can be a good starting point for future research on PBS.
4.
We provide a survey of the related methods and concepts that is not only invaluable per se, but also sheds light on the differences between PBS and the previously proposed works.
2. Perspective-based search
In this section, we introduce and conceptualize PBS. Moreover, we present some interesting types of perspectives in Section 2.1, which also help in clarifying the concept of PBS.
A basic scenario of PBS is illustrated in Fig. 1. The components in Fig. 1. are mentioned for illustrative purposes and different instantiations may have different components (see Section 3.1 for more details). A basic retrieval operation in PBS proceeds as follows:
1.
The user issues a query.
2.
The search component retrieves the search results as in a traditional search system.
3.
The perspective extraction component extracts the relevant perspectives. The component also scores and ranks perspectives based on their importance (and possibly user’s preferences).
4.
The user interface (UI) component, presents the search results to the user. Also, all or parts of the filtered perspectives are shown to users to make them aware of the existing perspectives on the topic (see Fig. 2 for a hypothetical example). The user can then select a desired perspective(s).
5.
The search component retrieves the results with respect to the selected perspective(s).
6.
The procedure continues from step 3 until the information need of the user is satisfied.
Fig. 1.

Fig. 2.

It is worth mentioning that extensions to the basic PBS are possible. One such extension is discussed in Section 3.1.4.
It is important to note that PBS, unlike some other approaches (e.g., see Sections 5.3.2 and 5.3.3), does not sacrifice the user’s autonomy to mitigate the information bubble. PBS emphasizes the role of users in the mitigation. It alerts users about the existence of different perspectives and allows them to consciously choose a desired perspective. We discuss this in more detail in Section 4.1, where we elaborate the strengths of PBS from an analytical point of view.
In PBS, a perspective in its most general sense can be formally defined as:
Given a query (q), a perspective (p ∈ perpectives(q)) is defined as a point of view from which the desired search results for q are different from the desired search results without considering p.
Given a collection of documents (D), a basic PBS engine accepts a query (q) from a user (u) and returns a list of candidate perspectives Pq,u(possibly together with other information such as the default search results) that are related to q and are possibly personalized according to the preferences of u. If the user selects one or a number of perspectives (P ⊆ Pq,u), the PBS engine returns a list of documents Rq,P ⊂ D, which are sorted according to their relevance to q from the perspective of P (maybe together with a new set of candidate perspectives).
In this sense, personalized search can be viewed as a special case of PBS, in which the perspective is “My Viewpoint”, i.e., P = {“My Viewpoint”}. In the following subsection, we explain some other possible perspectives types in PBS.
2.1. Perspectives
The general structure of PBS is generic and is not limited to any set of perspectives. In addition, in each application, PBS can be used by defining the appropriate perspectives according to the particular needs of that application. However, we present some interesting and general types of perspectives, which also helps in making the concept of PBS clearer. These perspectives address the following questions:
–
Different groups of people have different opinions and beliefs about different topics. How can we inform individuals of the opinions and beliefs of different groups?
–
Different people have different preferences and worldviews. How can we allow an individual to view the world from the perspective of another person?
–
Different media have different orientations and frame their contents differently. How can we allow an individual to view the Web content from the viewpoint of a media?
–
Demographic factors can affect the opinions and interests of people. How can we allow the searchers to view the search results from the perspectives of different demographic groups?
–
About some issues, some people are for the issue and some are against it. How can we inform the searchers about such opposing viewpoints?
It is noteworthy that although facets (see Sections 5.2.2 and 5.2.3) can also be considered as perspectives in PBS, we do not mention them here because they are more related to disambiguating the query than to bursting the information bubble and also because of brevity.
2.1.1. Belief perspectives
In a classic IR system, users access the search results either from a general perspective (nonpersonalized methods) or from their own perspective (personalized methods). Therefore, they are unable to view the searched topic from the viewpoint of different beliefs. In many circumstances, they may even be unable to identify different viewpoints existing on the search topic. This can cause the formation of information bubbles and lead to false perceptions of reality.
For example, suppose that a user believes in a particular religion and that religion is dominant in the user’s country. If the person wants to research other religions, a personalized search system may bias the results towards the user’s beliefs (e.g., by ranking the webpages viewed more by the user’s compatriots higher), thus leaning towards a point of view criticizing other religions. This can be unfair and result in severe misjudgments and more polarized viewpoints, which can trap the individuals into their limited understanding of the truth, i.e., into their own information bubbles. Also, another problem may happen when the user is in a minority by biasing the user’s search results (according to information such as the user’s social connections and geographical location) towards what the user is reluctant to (unless an exhaustive profile on the search topic is available indicating the user’s beliefs). The bias may even be inherent to the source data (White and Hassan 2014; Kulshrestha et al. 2017), and the search results may be biased even without any personalization and thus violate the openness norm (Bozdag and van den Hoven 2015) (e.g., when the number of followers of a religion is significantly lower compared to other religions and they have produced less online content). These problems can happen in almost any topic in which there are conflicting opinions, like many political, social, and even scientific topics.
Moreover, in these situations, formulating the queries that convey the real user needs with the traditional personalized paradigm may be challenging. For example, if users search for critiques on their religious beliefs, personalization techniques may bring up the results confirming their own beliefs like the documents written by the members of the same religion, quoting the critiques in a distorted manner and answering them unfairly, i.e., documents written in a similar manner to what Garrett (2013) mentioned about partisan information seekers: “There is little evidence that partisans are driven to form balanced opinions; instead, they seek information in order to better defend their position or ridicule others”. However, if the user were able to view the different existing perspectives on the topic in a categorized format, the user would be able to read the ones of interest and conduct research in a more fair fashion and with less partiality about the topic. As another example, one might want to know the attitudes of a country’s political orientation about a political issue. By including the country name in the query, the search engine normally brings up the pages mentioning the country name, whereas a page that describes a topic from a country’s political viewpoint does not necessarily mention the country name.
As a solution, in PBS different possible perspectives on the search topic are presented to the user, and the user chooses the desired perspective if wanted. This puts control in the users’ hands and allows the user to view the topic from different perspectives. For example, a Russian may search about “2014 Crimean crisis” and choose USA as the desired perspective or vice versa. Or a Christian may search a religious topic and mention Islam as the perspective.
2.1.2. Social networks members perspectives
Another possible type of perspective in PBS is to search from the viewpoint of a particular user (or group of users) in a social network, e.g., when a user is interested in another user’s preferences and wants to search a topic from the user’s point of view or wants to know what the user and those who are similar to the user think about a particular topic. Viewing the world from the perspectives of different people may also help the user in thinking outside the box and come up with more creative ideas (Teevan and Yu 2017). For instance, assume that in a social network for multimedia content a user’s taste of movies is similar to another user. By using PBS, a user can easily search the query “movie” with determining the similar user as the perspective to obtain the movies of interest. We call these perspectives social networks members’ perspectives. This type of perspectives could be considered similar to the traditional personalized search but with selecting a particular user (or group of users) as the perspective by the searcher, instead of using the searcher as the perspective.
It’s important to note that this type of perspectives does not interfere with users’ privacy since it considers only the public profile of the user. Moreover, some users may even try to enrich their profiles to help others better understand their beliefs. For example, a religious figure may add information and (or) links to websites that represent preferred beliefs and lifestyle to help the believers of that religion view things from the same perspective. Thinking this way, PBS may become an interesting media for publishing thoughts and lifestyle information. We discuss this in more detail in Section 4.2.
2.1.3. Data source perspectives
Data sources could also be used as perspectives. For example, if an individual wants to know the attitudes of the Republican Party towards a particular political or social topic, the individual could choose a news agency with Republican leanings, e.g., Fox News (Morris and Francia 2010), as the perspective and obtain the results from that point of view. Similarly, one could search by choosing Xinhua as the perspective to retrieve results from the viewpoint of the Chinese government. The important point is that unlike traditional IR operators like “site:” or “insite:”, the search results should not be limited to the documents of the chosen data source and the data source is only used as a guidance to what really the user intends to see. This allows the user to simply enter what the user is searching for without sacrificing the retrieval recall. An interesting application of this type of perspective is to provide a search facility in a website (e.g., a news agency) in which the users can search the Web from the perspective of the website.
2.1.4. Demographic perspectives
Demographic factors could also be used as perspectives. Users with different personalities, expertise, age ranges, language proficiencies, etc. could have different information needs. Allowing users to choose the demographic perspective themselves allows them to indicate their search goals more conveniently. For example, mentioning “kid” as perspective along with query “poem” could allow the mother of a child to find childish poems easily. As another example, a user searching about food may choose a country as the perspective to become familiar with the food culture of the country.
Some, but not all, of these factors are taken into consideration in some search systems (e.g., search engines for children (Gossen 2015)), but presenting them in a uniform format, as in PBS, can be beneficial. More details on the benefits of this unification are explained in Section 4.1.5.
2.1.5. Sentiment perspectives
Although there are some tools for retrieval based on the sentiments (see Section 5.4.1) of the results (e.g., Zheng and Fang 2010; Demartini 2011; see Section 5.3.1), PBS offers a straightforward way of integrating sentiment-based search into general search engines. This can be done by considering sentiment-related perspectives (e.g., negative and positive) in PBS.
3. Implementation hints
In this paper, we do not aim to provide any specific implementation. However, in this section, we provide some hints on implementing a PBS system. In this section, we assume that the process and the components mentioned in Fig. 1 are used in PBS. First, in Section 3.1, we explain the components in more detail. Then, in Section 3.2, we provide some guidelines on how to acquire the required information for PBS. Finally, in Section 3.3 we mention two suggestions on the design of a PBS system.
3.1. Components
Here, we explain the components shown in Fig. 1 in more detail. It is worth noting that more components could be employed to take greater advantage of PBS. An example of such a component is described in Section 3.1.4.
3.1.1. Search component
The search component is the main retrieval component of a PBS system. This component retrieves the results for the query and with respect to the selected perspective(s). At first, when the user issues a query and still no perspective is selected, the search component considers a default perspective in the retrieval. The default perspective can be either “My Perspective” (which results in personalized results) or “General Perspective” (which results in nonpersonalized results). Then, when the user selects one or more perspectives, the component retrieves the results based on the perspective(s).
3.1.2. Perspective extraction component
The perspective extraction component is responsible for identifying and categorizing relevant perspectives in PBS. For example, it identifies what types of viewpoints are available for the searched topic, or it explores a social network’s users and suggests specific pundits as perspectives, or it detects that there are conflicting opinions on the topic and considers positive and negative perspectives as the relevant perspectives.
Also, since many relevant perspectives may exist for a query, and considering the importance of the UI, this component ranks the extracted components to allow the UI component to better present them to the user. Some of the current research problems on retrieval (ranking documents) could also be raised about this ranking (ranking perspectives). For example, a system can personalize the ranking based on the user’s preferences.
3.1.3. UI component
One of the most important points that demands special attention in PBS is the user interaction. Some UI approaches are used in similar IR tasks. For example, in search result clustering (SRC), the clusters are mainly represented as lists or hierarchies (see Fig. 3). However, we think that the traditional representation of concepts in the form of lists or hierarchies has not been very successful, and we present our suggestion.
Fig. 3.
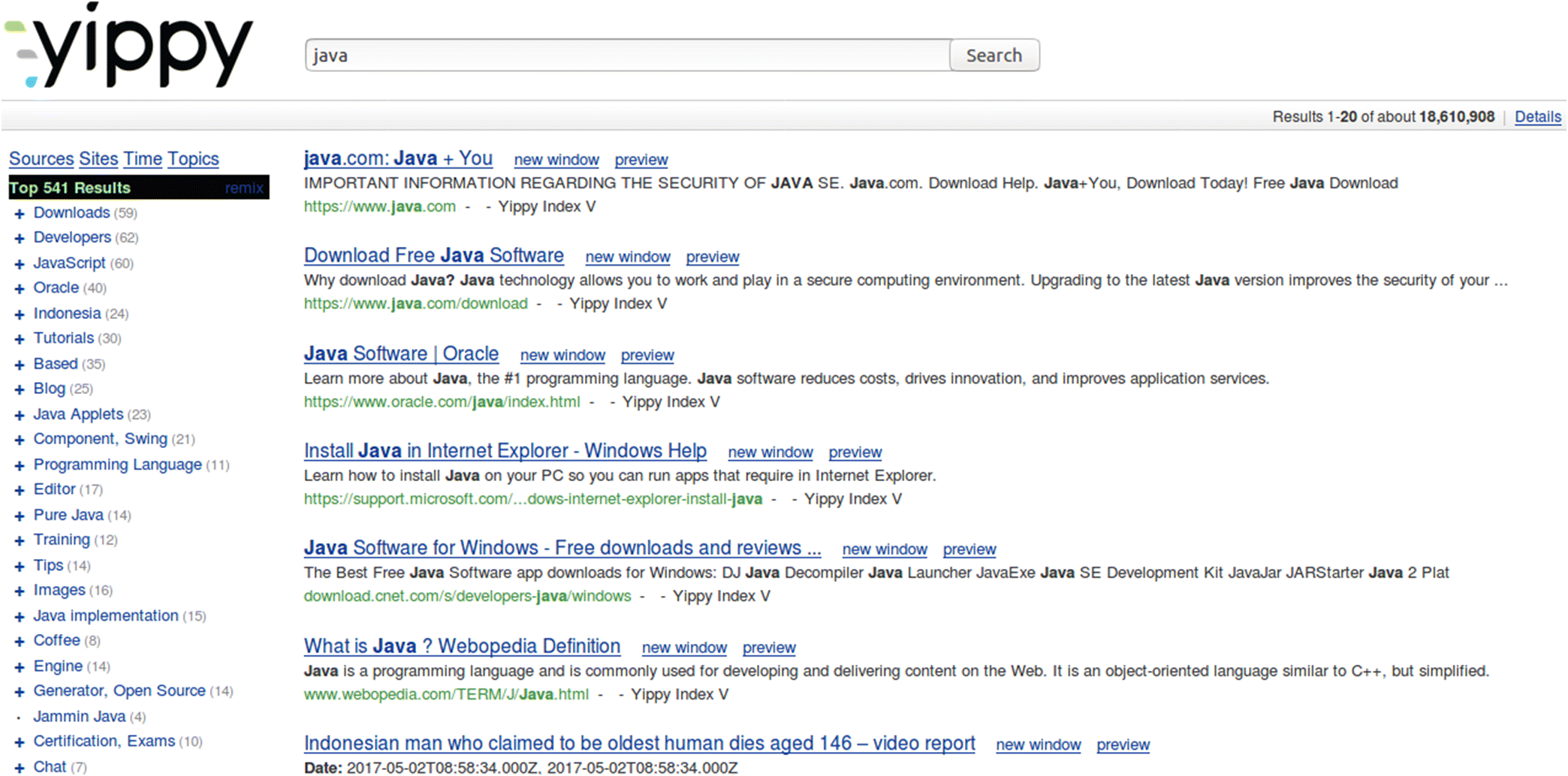
One of the reasons why most of the Web clustering engines (Carpineto et al. 2009) were not welcomed with open arms by the users may be that their over-informativeness and complexity make the users confused and disoriented (e.g., see the sidebar in Fig. 3). Therefore, UI is important in PBS. One of the solutions to this issue is to rank all the possible perspectives and display only the most interesting ones to the user instead of displaying a mess of perspectives and creating confusion. Figure 2 shows an example UI, in which a perspectives area is added to a search results page. In this UI design, the default list of results is shown as the search result and the perspectives of interest are also shown to the user; the search perspective can then be changed if desired. In the example, “Republican Party” and “Democratic Party” are suggested as perspectives to an American user searching about the abortion laws, and the user can change the search perspective with just a single click. Moreover, an option to view more perspectives on the topic is provided to the user beneath the suggestions to present additional perspectives to the user.
3.1.4. Perspective search component
In addition to the core components, some supplementary components may be integrated into a PBS system. For example, a search topic may relate to many perspectives, and viewing all of them may be time-consuming for the user. A PBS system may employ a perspective search component to allow the user to search among the related perspectives. The component can help the user to find the desired perspectives by showing perspectives that are relevant to the perspective search query. For instance, the user may enter US as the perspective search query, whereas the system uses United States of America; the perspective search component retrieves the perspective as the result of the user’s perspective search. Figure 4 shows an example of perspective search in which auto-completion is also offered.
Fig. 4.

3.2. Knowledge acquisition
A PBS system requires at least two types of information: (i) what each perspective is and (ii) how the perspectives relate to documents. Having access to the required information, one may use existing methods to implement PBS (e.g., by using the existing personalization methods to bias the results towards the selected perspective), although more research is needed to develop, test, and validate existing and new methods. Several approaches to obtain these types of information are possible. In the following, we describe some possibilities from different fields of research.
3.2.1. Crowdsourcing
One approach is to obtain the information by user collaboration and from user-generated content (based on Web 2.0 principles). This approach has been used in many websites and is shown to be practical, effective, and successful. We can name Wikipedia,1 Pinboard,2 Stack Exchange,3 and reCAPTCHA4 as some successful examples of this approach, in which their content is generated by the users and the focus is not on artificial intelligence for generating the content. A similar approach can be employed for PBS. In other words, users help to identify perspectives and relate them to documents. For example, users define perspectives by providing the perspective labels (e.g., the Republican Party) and the corresponding Wikipedia pages as the descriptions. Meanwhile, users tag the webpages with the defined perspectives, similar to folksonomy websites like Pinboard. It should be noted that this approach is prone to mistagging and employing methods for detecting mistags may become necessary over time. Other crowd-sourcing methods are also possible. For example, one might employ games to collect the required information (gamification) (von Ahn 2006). Another approach to obtain the information from users is discussed in Section 4.2.
3.2.2. Journalism and the Semantic Web
Some websites such as Debatepedia,5 Debate.org,6 Debatebase,7 and ProCon.org8 provide information on controversial issues, conflicting opinions, different sides of debates, etc. Another approach is to use data on these websites to identify perspectives and the corresponding webpages. Also, the Argument Web (Bex et al. 2013) and Argument Interchange Format (Chesñevar et al. 2006) can facilitate obtaining the required data and semantics in a machine-readable format. Although this approach may be a low-cost solution to obtain the required information, the available data may be limited and available only for some topics. This can make its applicability limited.
3.2.3. Information retrieval
An extension to the approach suggested in Bennett et al. (2015, p. 1) also seems promising. They propose using “the aggregate search behaviors of others in the beneficiary’s demographic groups” in searching on behalf of others (see Section 5.3.6). Based on the existence of selective exposure in humans, search engines can estimate the membership of a user to a group, say Muslims, by observing their interactions with the system. After identifying many members of the group, the search engine can then observe their behaviors in other topics, e.g., searching for a restaurant, and use their aggregate search behaviors in retrieving the results from the perspective of that group, possibly resulting in Halal restaurants. Moreover, by observing different behaviors in different groups on a search topic, the search engine can infer that the groups have possibly different opinions on the topic, and thus suggest the groups as perspectives in the searches on that topic. For example, by observing that in the United States those who are inclined toward the Republican party and those who are inclined toward the Democratic party behave differently on searches about “progressive tax”, it can deduce that the Republican party and the Democratic party are two candidate perspectives for the search topic in the United States. This approach can be considered as an implicit form of using user-generated content, in which the required information is implicitly extracted from the collaborations of users with the system.
3.2.4. Machine learning
Another approach is to use machine-learning tools to automatically extract the required information. This approach demands special attention from academia and raises new research questions on how to extract the required information automatically. Some results in previous works suggest that the automatic extraction is indeed possible. For example, Lin et al. (2006, p. 6) stated that, “The results suggest that the choice of words made by the authors, either consciously or subconsciously, reflects much of their political perspectives. Statistical models can capture word usage well and can identify the perspective of documents with high accuracy.” Thus, unsupervised approaches similar to the ones used for topic modeling (Blei and Lafferty 2009) may be useful for identifying perspectives. However, at least at the early stages, some kind of supervision may be required for learning such methods. The abovementioned approaches may be used for the supervision. For example, data on websites such as Debatepedia may be used to learn the methods. Moreover, top-down methods for creating a Semantic Web (Stevenson and Ciravegna 2003; Iskold 2007c) (e.g., see Li and Huan 2008) may be helpful. Some of the methods used in the papers mentioned in Section 5.4 may also be applicable. In particular, extracting the required information for some perspective types may be easier than the others. For example, the information for the sentiment perspectives may be relatively easily extracted using methods similar to the one used in Zheng and Fang (2010). Moreover, a bootstrapping approach, similar to the one used in Park et al. (2011a), may be employed—that is to say, the system tries to automatically extract the required information with an acceptable precision, and then the users can amend the information.
3.2.5. Social networks
Because of the existence of social phenomena like homophily, analyzing the link structure of the Web and social networks can help in revealing the existence of polarizations and finding stances on topics. For example, Dori-Hacohen (2017) showed that Wikipedia articles exhibit homophily, i.e., linked pages are more likely to have the same controversy label than nonlinked pages. It leverages the phenomenon to detect controversy in Wikipedia. Thus, one way to obtain the required perspective information is by analyzing the link structures of the Web and social networks, which requires further research.
3.2.6. Semantic Web
As time passes and PBS becomes more widespread, development of ontologies/semantic networks/knowledge graphs (OSKs) for relations of concepts/topics and perspectives could facilitate the search as well as production of more useful and exhaustive OSKs (before that, available OSKs like DBpedia9 can be used for this purpose). Using Semantic Web inference techniques is also promising. For example, the system may use the information (e.g., click-through data) about the perspectives of the topics similar to (according to the OSK) the searched topic to enrich its information about the perspectives of the searched topic. This can help reduce the sparseness problem. As an example of the use of OSKs in PBS, suppose that the user selects “Russia” as the perspective when searching about a political issue; the system can detect that “Vladimir Putin” is the president of Russia according to an OSK, and thus also retrieve pages tagged with the “Vladimir Putin” perspective but are not explicitly tagged with the “Russia” perspective. Also, website owners currently use mark-ups and tagging (e.g., with Schema.org tags) to better introduce their contents to search engines and to raise their ranks in searches. The very same idea can be employed in PBS. In other words, content producers may tag different parts of their websites with perspective tags, representing the relevancy of those perspectives, which can be a rich and reliable source of information about perspectives. This approach is similar to the bottom-up approach of creating a Semantic Web by embedding semantic annotations into the data (Iskold 2007b). The success of the approach in the Semantic Web (e.g., Guha (2013) stated that two years after the launch of Schema.org over four million Internet domains use its markup) suggests that it may be a promising solution for implementing PBS.
3.3. Design suggestions
There are two considerations about PBS design that are worth sharing:
–
When the user selects a perspective (or perspectives) in a PBS system, the system has the option to, or not to, personalize the search results according to the user’s preferences. However, our suggestion is not to personalize the results when a perspective is selected so as not to lose common reference points among individuals (Resnick 2011).
–
There are some personal or situational factors that can affect an individual’s tendency to explore more aspects of a searched topic. For example, Munson and Resnick (2010, p. 1) showed that there are differences among individuals: “…some people are diversity-seeking while others are challenge-averse.” Diversity-seeking users may be interested in many existing perspectives on the search topics, whereas presenting many perspectives to challenge-averse users may be dissatisfactory. Similarly, Liao and Fu (2013) showed that topic involvement (as a personal factor) and perceived threat (as a situational factor) influence users’ selective exposure to information. These factors can be considered in PBS to deliver a better user experience. For instance, users with a high topic involvement may be more interested in scrutinizing different perspectives of the topic, and thus presenting a larger number of and more conflicting perspectives may gratify them more.
4. Discussion
We discuss the strengths of PBS in Section 4.1, and in Section 4.2 we explain that PBS can be employed to promulgate worldviews and lifestyles.
4.1. Strengths of PBS
PBS is a solution to the problem of mitigating the information bubble. Plenty of solutions have been proposed to address the problem (see Section 5.3). However, PBS provides several benefits of the existing approaches, while averting several of their drawbacks. In the following subsections, we discuss the strengths of PBS in more detail.
4.1.1. Promoting awareness of the information bubble
One of the main advantages of PBS is that it promotes users’ awareness of the information bubble problem. This is done by presenting the users with different viewpoints on their search topics and informing them that search results can be different from each viewpoint. This is in contrast to some other approaches like enabling serendipity (Section 5.3.2), diversification (Section 5.3.3), and using intermediary topics (Section 5.3.5). This characteristic is important for the search engine to have the value of transparency discussed in Bozdag and Timmermans (2011). It allows the users to understand the limitations of their bubbles and try to get out of their bubbles if the topic really matters. In addition, this can promote critical thinking and avoid believing falsehoods.
PBS also informs the users about different viewpoints on the search topic and allows them to view the results from those viewpoints. By informing individuals about different stances on the topic, PBS can prevent individuals from moving to extremes and forming polarized views of the topic.
4.1.2. Context modeling
One of the challenges in search personalization is to correctly handle the user’s context. For example, suppose that a user has always been a fan of a brand like Apple, and the user’s profile is tied to it, but for some reason the user is now searching for positive or neutral information on a rival like Microsoft. In this case, a personalized search engine may bias the results toward the unintended ones. As another example, the search results for a believer of a religion, say Christianity, may be biased when searching about another religion, say Islam, in a personalized search system. These biases may trap the users into their own information bubbles.
Retrieving the appropriate results for the user in different contexts and avoiding unwanted biases was a challenge in the traditional personalized search engines. PBS solves the problem in a straightforward way by allowing the user to control the search. This control lets the user exploit the power of personalization without being stuck in the information bubble. PBS thus conforms to the value of identity discussed in Bozdag and Timmermans (2011).
4.1.3. User autonomy
A characteristic of PBS that is of paramount importance is that it respects users’ autonomy. Unlike several other approaches (e.g., see Sections 5.3.2, 5.3.3, and 5.3.5), the approach taken by PBS puts the users in the driver’s seat and allows them to choose when and how they want to view the topic from other perspectives. Even, PBS allows the users to knowingly insist on their own viewpoints and choose to view the topic from their own perspectives (e.g., selecting their political party as the perspective), although after they are apprised that there are other perspectives. Thus, PBS conforms to the value of autonomy discussed in Bozdag and Timmermans (2011). Respecting users’ autonomy also avoids several possible negative civic and ethical implications (see Bozdag and van den Hoven 2015; Dori-Hacohen et al. 2015) and increases the trustworthiness of search engines.
4.1.4. Minimum overhead
PBS can be provided to the user without much modification to the well-established UI of search engines. The user can perform normal search operations and PBS does not intervene in the day-to-day search tasks. This is in contrast to the some approaches such as clustered presentation (see Section 5.3.4) in which a totally different UI is presented to the user. This characteristic is crucial for popularizing a system aiming at mitigating the bubble, because a different UI will probably require providing PBS in a different webpage, which can dramatically narrow possible users.
Moreover, in many circumstances being informed about different perspectives on the search topic is not important to the user and manipulating the original, possibly personalized, search results to cover different perspectives (e.g., in the diversification approach; see Section 5.3.3) may even reduce user satisfaction. The weaker performance is also shown to exist in other coercive mitigation approaches (e.g., see Graells-Garrido et al. 2016a). Because of reduced satisfaction, such an approach may not be an appropriate option for search engine provider companies and may cause conflicts of interest. Our suggested UI for PBS shows the user the default search results without any manipulation, but it also informs the user about the existence of different perspectives (Fig. 2) and allows the user to intentionally select a perspective. This can avoid reducing the user satisfaction, while helping to mitigate the information bubble. Not having this property can be a big barrier to the adoption of a solution for bubble mitigation by major search engines.
4.1.5. A uniform approach
Although each of the perspective types proposed in Section 2.1 could benefit users in several aspects, the power of PBS is not limited to the advantages of its individual perspectives. Viewing all those points of view in a unified form, named perspectives, puts a lot of flexibility into the paradigm. First, the user could become familiar with all of the features in a uniform format, which can reduce the overhead of feature adoption considerably. This decreases the cost and time of learning how to interact with the system and a better experience is delivered to the users. This can be contrasted with the previous approach in which the user had to visit a website for kids’ search, another one for sentiment-based search, etc.
Second, by uniforming many capabilities, a common ground for research is established in which synergy can take place. Moreover, new research problems arise and new facilities can be delivered to users. An example (mentioned in Section 3.1.2) is personalization in ranking perspectives: ranking the perspectives with respect to both the query and the user’s preferences. Another example is perspective search, which was discussed in Section 3.1.4.
Furthermore, by representing many points of view in a unified form, combining different points of view (perspectives) would also be possible, which lets the user refine the point of view and gradually add more perspectives.
4.2. PBS as a medium
PBS can also be seen as a medium. Suppose that a group of people with a particular worldview (or interest), say Muslims, want to promulgate their worldview. One approach could be to build a rich representation of their worldview in PBS to nudge people into seeing their opinions. For example, they can provide an exhaustive list of webpages related to the “Muslim” perspective so that the search engine can detect perspective Muslim in relevant searches and suggest it to the users, which can raise awareness on Islam. Moreover, this can help like-minded people to find relevant results easier. For instance, by listing Halal restaurants in the Muslim perspective’s list, Muslims can help each other find Halal restaurants. This can also be seen as a form of advertising that can benefit the like-minded community.
The list-wise approach can be compared with the tagging approach mentioned in Section 3, in which users could tag webpages with different perspectives. The list-wise approach is more centralized and the list can be considered as an abstract representation of the perspective, an abstract vegetarian in our example. The better the followers of a worldview develop its representation, the better and easier the searchers find information from that worldview. The representation may be developed in an open and democratic fashion. For example, a website similar to Wikipedia may be created, in which users can freely amend the representations of perspectives and add new webpages to them. The representations may even become enriched by adding semantic elements to them, e.g., mentioning that a vegetarian has a negative sentiment towards meat. The enrichment may be done by developing perspective-focused semantic networks or linking perspectives to the nodes of existing semantic networks.
On the other hand, search engines and other information systems may also provide their users with an option to permit the system to use their information (e.g., their search logs) to enable other users to view the world (e.g., search the Web) from their perspectives. The users may be interested in allowing this to happen, e.g., because they want to promulgate their worldviews.
5. Related work
In the following subsections, we first discuss the information bubble in more detail. Then, in Section 5.2, we explain the IR approaches that are related to PBS. In Section 5.3, we survey different approaches to overcome the information bubble. Finally, we discuss several concepts that may be deemed similar to our concept of perspective in Section 5.4.
5.1. Information bubble
In this subsection, we explain the filter bubble and the echo chamber phenomena.
5.1.1. The filter bubble
The filter bubble was coined by Eli Pariser in his book The Filter Bubble: What the Internet Is Hiding from You (Pariser 2011). Pariser discussed three challenges with respect to the personalized filters: (i) “… you’re alone in it. A cable channel that caters to a narrow interest (say, golf) has other viewers with whom you share a frame of reference. But you’re the only person in your bubble. In an age when shared information is the bedrock of shared experience, the filter bubble is a centrifugal force, pulling us apart.” (p. 10) (ii) The filter bubble is invisible. When you visit a conservative or liberal news source, you may know that you are visiting content curated to present a particular viewpoint. But when you use a personalized information system, you probably do not know what the system thinks about you and how it models you and whether it is right or wrong. The system decides what you should see and you may not even be aware of the existence of such personalized filters. (iii) You don’t choose to enter the bubble. When you read a particular newspaper, you have actively decided which newspaper to read and probably have some ideas about its leanings. You are the one who decides what kind of filter to use in obtaining information about the world. But, with personalized filters, you do not make such a decision. Filters come to you and they are hard to avoid, because they drive up profits for the companies who use them.
The filter bubble problem can arise in different systems. For example, in a search engine it may arise through personalization algorithms. In a recommender system, it may arise through recommender algorithms. And in a social network news feed, it may arise through algorithmic curation (Berman and Katona 2018) of content.
The concept of filter bubble has attracted much attention, resulting in many studies. Davies (2018, p. 14) redefined filter bubbles as socio-technical recursion: “…users coming back for more because they like the suggestions offered to them which have been inferred from their previous searches.” Some research validated the existence of filter bubbles (e.g., see Nguyen et al. 2014), whereas the other findings (see Bechmann and Nielbo 2018; Dutton and Fernandez 2019) suggested that the filter bubble problem may not be that severe. Tran and Herder (2015) introduced a method for detecting the filter bubble in ongoing news stories. Bozdag and van den Hoven (2015) and Bozdag (2015) provided interesting explanations on the relations between the filter bubble and different democracy models and also the relations between tools introduced to mitigate the filter bubble and the democracy models. Moeller and Helberger (2018) reported on the effects of algorithmic news recommendation systems or filter bubbles. Resnick et al. (2013) reviewed some approaches for bursting the information bubble and promoting diverse exposure.
5.1.2. The echo chamber
The echo chamber theory (Sunstein 2001a, 2001b, 2007) has its roots in the social science concept homophily (McPherson et al. 2001) and in the psychology-related concept selective exposure (Sears and Freedman 1967; Frey 1986). Cavoukian and Chibba (2018, p. 37) explained: “In news media an echo chamber is a metaphorical description of a situation in which information, ideas, or beliefs are amplified or reinforced by transmission and repetition inside an ‘enclosed’ system, where different or competing views are censored, disallowed, or otherwise underrepresented.” Echo chambers are shown to exist in social media (An et al. 2013a). One may argue that the echo chamber phenomenon is more severe in cyberspace, because in the real world people are compelled to have social interactions with many people (not necessarily with those they want to) and this makes them exposed to different topics and viewpoints. Garrett (2013) authored an interesting paper on selective exposure that reflects the state of the research area that offered some criticism on the echo chamber concept.
5.2. Related IR tasks
We discuss three related IR tasks: personalized search, SRC, and faceted search.
5.2.1. Personalized search
As opposed to the traditional system-centered IR methods, personalized methods rely on a user-centered approach and try to tailor the results to the user’s preferences. Indeed, personalized search usually tends to bias the results towards the interests of the user according to the available information. Personalized search can be considered as a tool for implementing PBS. There are many studies on personalization in IR that are beyond the scope of this paper; readers are referred to Ghorab et al. (2013), Bouadjenek et al. (2016), Pasi (2010), and Steichen et al. (2012) for more information.
Personalization could severely lead to the problem of information bubble. Unlike traditional personalized search systems in which the only perspective is “My Perspective”, in PBS different existing perspectives about the search topic are presented to the user and the user can select the desired perspective. Thus, PBS can deliver the benefits of personalization to the user without sacrificing breadth of vision or compromising autonomy.
5.2.2. Search result clustering
An IR approach related to PBS is SRC (Carpineto et al. 2009). Because a query may be related to multiple topics or subtopics, clustering search results and presenting the clusters to the user can help the user search more conveniently. For example, for the query “Java”, the results are categorized into different categories such as “Programming Language”, “Drink”, “Geographic Location”, etc. Figure 3 shows an example of the output of such system, Yippy10 (formerly Clusty (Iskold 2007a)). Some other related tools are KeySRC (Bernardini et al. 2009), Lingo (Osinski and Weiss 2005), Lingo3g11 (Osinski and Weiss 2007), and SnakeT (Ferragina and Gullì 2004).
SRC is different from PBS in four aspects. The first and the most important difference is that in SRC the results are categorized based on their facets (e.g., programming language, drink, island, etc. for query Java), whereas in PBS different types of perspectives could be considered but only one is a perspective based on the result facets. Thus, SRC could be considered as a special case of PBS.
Moreover, in SRC clusters are expected to mostly represent concrete concepts that are explicitly mentioned in the documents (e.g., using Java as a programming language in a document), whereas perspectives in PBS may refer to abstract (possibly subjective) concepts not necessarily mentioned directly in the documents (e.g., being from a liberal viewpoint).
In addition, SRC is mainly focused on helping the user find and select the desired search results, whereas the focus in PBS is on mitigating the information bubble.
On the other hand, in the popular approach to SRC, the categories are represented as lists or hierarchies, which are confusing to the end user and waste time finding the appropriate results. This kind of UI is very likely to make users reluctant to use the provided categories. The common approach to UI in such systems may be one important reason why these systems are not so popular. Carpineto et al. (2012, p. 15) stated that “… while it [clustering] is less effective for partial subtopic coverage because of the additional cognitive effort required on the part of the user to browse through the cluster labels before examining any document”. The problem is resolved by the UI suggested for PBS, in which the results are presented in a simple format with minimum intervention the user’s common searches (see Section 3.1.3).
5.2.3. Faceted search
Another IR approach related to PBS is faceted search (Tunkelang 2009; Wei et al. 2013). Zelevinsky (2010) defined facets as: “navigable and summarizable properties, tagged onto the records in the system.” Faceted search is commonly used in e-commerce websites (e.g., Amazon12) and is also used in other search systems on metadata-rich collections (e.g., library.oum.edu.my/oumlib/iportal_search/faceted); items are gvien tags such as type, category, author, subject, format, and price that can be used to navigate the collection for the desired items. The main difference between faceted search and SRC is that in faceted search items are, presumably manually, tagged with facets, whereas in SRC the clusters are generated at the query by clustering the search results. Hearst (2006) provides a more detailed comparison between faceted search and SRC.
There are three major differences between faceted search and PBS. First, faceted search is mainly defined in the domain of documents with manually tagged facets, whereas PBS is broader and can be used in a Web search. Second, similar to SRC, faceted search is mainly related to facet perspectives, whereas PBS covers different perspective types, e.g., belief perspectives. Lastly, faceted search is focused on facilitating exploration of the collection by the users, whereas the most important goal in PBS is mitigating the information bubble.
5.3. Mitigating the information bubble
We present different approaches proposed to mitigate the information bubble in IR related tasks such as search and recommendation.
5.3.1. User awareness and visualization
One approach to mitigate the information bubble is to promote the user’s awareness about different dimensions of the task, e.g., search or recommendation by UI facilities and visualization techniques. For example, Carson (2015) proposed recommending that Google allows users to manually toggle between personalized and nonpersonalized results (e.g., through a switch icon). This causes users to pay more attention to the information bubble phenomenon and understand its existence as well as empowering them to control it. Much earlier in 1990s, Negroponte (1995, p. 154) coined “The Daily Me” to refer to personalized news summaries and “The Daily Us” to refer to nonpersonalized news summaries, and then suggested: “These are not two distinct states of being, black and white. We tend to move between them, and, depending on time available, time of day, and our mood, we will want lesser or greater degrees of personalization. Imagine a computer display of news stories with a knob that, like a volume control, allows you to crank personalization up or down. You could have many of these controls, including a slider that moves both literally and politically from left to right to modify stories about public affairs.” Stracke (2014) suggested allowing the users to modify the amount of personalization in search by enabling or disabling categories of search signals (e.g., search history, browsing history, and geography). Nagulendra and Vassileva (2014) suggested visualizing the filter bubble and allowing the user to control what resides inside it and what does not. The user controls which categories to be inside the bubble and which users to be inside it for each category. Similarly, El-Arini et al. (2012) proposed transparent user models, i.e., user-interpretable badges such as “liberal” or “Apple fanboy”, for personalization, which can make the filters more transparent to users and allow them to correct them. Demartini and Siersdorfer (2010) and Demartini (2011) proposed showing the sentiment polarity (see Section 5.4.1) of each search result next to it so the user can view results with different sentiments. Demartini (2011) also allowed the user to filter the results based on their sentiments. Aktolga (2014) proposed a similar approach based on sentiments but also suggested showing the times, i.e., time references mentioned within documents. Gao et al. (2018) designed a system that provides visual hints and recommendations of opinions on different sides with different indicators. They concluded that the proposed system “is promising in raising users’ awareness of their own stances and preferences, as well as mitigating selective exposure to information” (Gao et al. 2018, p. 10). Zheng and Fang (2010) presented a retrieval system that delivers search results in “for” and “against” categories and tries to find the topics inside each category and show the corresponding topics (two topics discussing the same query perspective but expressing different opinions) next to each other. Liao and Fu (2014a) considered displaying a source position indicator, showing both valence (pro/con) and magnitude (moderate/extreme) of positions on controversial topics and examined its effects on users’ selection and reception of diverse opinions. They also considered and examined the effects of a source expertise indicator, i.e., a design feature that explicitly distinguishes information sources with higher competence (Liao and Fu (2014b). Liao et al. (2015) also considered providing aspect indicators (e.g., displaying whether a comment is about side effects or effectiveness of a medicine) to mitigate selective exposure. They argued that providing the aspect indicators to users can reduce their resistance to diverse opinions and encourage them to explore information from different perspectives, since it can potentially narrow down their biased beliefs to a subset of aspects, and thus reduce cognitive dissonance. Schwartz (2012) explained Bing’s elections portal that can filter news sources with right or left political leanings. Similarly, The Wall Street Journal provides “Blue Feed, Red Feed”,13 in which it categorizes the Facebook posts on some controversial topics into two liberal and conservative categories and displays them side by side. Oh et al. (2009) evaluated the effect of displaying the political orientation (see Section 5.4.5) of blog posts in the weblog search task. Munson et al. (2013) and Munson (2012) developed a browser widget that shows the relative amount of the user’s liberal and conservative visited pages to nudge the user to have a more balanced reading behavior. Munson and Resnick (2010) also evaluated the effect of simple presentation techniques such as highlighting agreeable items or showing them first on readers’ satisfaction.
Some general differences between works in this approach and PBS are:
1.
They are mainly limited to one or a few perspectives, e.g., left/right leaning. PBS considers several perspective types.
2.
The perspectives they consider are mainly query-independent, e.g., positive/negative sentiment or right/left leaning, whereas PBS allows a different set of perspectives for each query.
3.
Many studies (Negroponte 1995; Munson and Resnick 2010; El-Arini et al. 2012; Munson 2012; Munson et al. 2013; Liao and Fu 2014a, 2014b; Nagulendra and Vassileva 2014; Liao et al. 2015) focused on problems other than search, e.g., filtering/recommendation, browsing comments, or browsing news sites.
4.
Many studies focused on user awareness (e.g., Munson 2012; Munson et al. 2013) or filtering the results (e.g., Schwartz 2012) but not both, whereas PBS increases users’ awareness on different perspectives on the search topic and also allows them to filter the results accordingly.
5.3.2. Serendipity
Maccatrozzo (2012, p. 2) proposed that another approach for mitigating the information bubble is to enable serendipity; they defined serendipity as “the ability to make fortunate discoveries by accident”. Maccatrozzo et al. (2017, p. 1) also used a similar approach and defined serendipity as “making a pleasant and relevant discovery that was unexpected”. Recommending serendipitous items to the user can mitigate the information bubble in two ways: users become aware of items outside of their own bubble and these items may catch users’ attention and encourage them to explore new paths and visit unprecedented items. Exploring these new items may also affect future recommendations.
Serendipity can be contrasted with PBS in several ways.
1.
With serendipity the user does not control the mitigation process. The user may be unaware of the decisions the system makes and has no control of when and how to do the mitigation.
2.
In serendipity the user does not control the mitigation process resulting in several ethical issues (see Bozdag and van den Hoven 2015; Dori-Hacohen et al. 2015).
3.
Unlike the search task of PBS, the sernedipity methodsare used for recommender systems.
4.
Using serendipity may, in some cases (e.g., for challenge-averse users (Munson and Resnick 2010)), reduce user satisfaction. Not allowing the users to control when they want to explore serendipitous items may reduce their satisfaction of the recommended items (similar to Graells-Garrido et al. 2016a).
5.
The effect of using serendipity to mitigate the information bubble is very limited compared with PBS. In this approach, the users are not becoming aware of the bubble problem and also cannot control it. Moreover, the serendipitous items may not represent different viewpoints but only different topics that may be interesting to the user.
5.3.3. Diversification
Another popular approach to mitigate the information bubble is to diversify the results in search engines or recommender systems. Diversification has been employed for other goals (e.g., tackling query ambiguity; see Santos et al. 2015) and several methods are proposed for it (e.g., Dang 2014).
Demartini (2011), Giachanou et al. (2014), Aktolga and Allan (2013), Aktolga (2014), Kacimi and Gamper (2011, 2012), and Meguebli et al. (2014a) considered diversifying the search results according to their sentiments. Aktolga (2014) considered diversification by time and opinionatedness. Kacimi and Gamper (2011, 2012) and Meguebli et al. (2014a) used the semantic similarity of documents (the Jaccard similarity of topic elements and (or) key concepts of documents) to obtain semantic diversity, which can be considered a form of aspect-based diversity (see Section 5.4.3). Kiritoshi and Ma (2014, 2015, 2016) addressed sentiment-based diversification of news, and they also considered a form of aspect-based diversification by using two measures “difference in factor coverage” and “difference in details”. Park et al. (2013) introduced agenda diversity and questioned its methods. Graells-Garrido (2014), Graells-Garrido and Lalmas (2014), and Graells-Garrido et al. (2016b) considered diversifying user timelines (e.g., in Twitter) according to the geographical dimension (see Section 5.4.6) of micro-posts. Wang et al. (2015) attempted to employ online analytical processing (Han et al. 2011) techniques to generate brief personalized summaries delivering news updates in multiple dimensions (such as entity, topic, and time). Although this paper does not focus on diversification or bursting the information bubble, its approach of presenting only updates of the news without repeating what the user has already read may indeed cause it to present diverse information to users. Munson et al. (2009a, 2009b) addressed diversifying news aggregators according to political orientations (see Section 5.4.5). Yom-Tov et al. (2014) focused on increasing exposure to different political opinions and discussed the role of language models (Zhai 2008) on people’s exposure to opposing viewpoints. When the language model of a document is similar to that of an individual, the document is more likely to be read despite describing an opposite viewpoint. On the other hand, An et al. (2013b) placed doubt on the wisdom of exposing people to diverse opinions.
Diversification can be contrasted with PBS in several ways.
1.
The methods in diversification mainly consider only one or a very limited set of perspectives for diversification (e.g., only sentiment). PBS presents a unified framework that can support many different perspective types.
2.
Unlike PBS, in diversification the user does not control the mitigation process. The user may be unaware of the decisions the system makes and have no control on when and how to do the mitigation.
3.
In diversificationwhen the user does not control the mitigation process it brings up several ethical issues (see Bozdag and van den Hoven 2015; Dori-Hacohen et al. 2015).
4.
Using diversification may reduce user satisfaction. Most of the times the users may not be curious about different perspectives. In addition, some users may be challenge averse (Munson and Resnick 2010). Not allowing the users to control when they want to explore more perspectives and just modifying the default (possibly personalized) relevancy-based ranker to increase diversity can reduce their satisfaction of the search results (similar to Graells-Garrido et al. 2016a for a recommender system).
5.
The effect of using diversification is limited compared with PBS. In this approach, the users are not made aware of the bubble problem, are not explicitly informed about the existence of other perspectives on the search topic, and cannot control which perspective to view.
5.3.4. Clustered presentation
Some studies (Park et al. 2008, 2009, 2010, 2011a, 2012; Ma and Yoshikawa 2009; Chhabra and Resnick 2012, 2013) considered presenting different dimensions of news events as separate clusters to users to mitigate media bias. In clustered presentation, news is clustered according to its aspects (see Section 5.4.3). The clusters are presented to users to make them aware of the presence of different dimensions and help them explore different dimensions, thereby mitigating the biases incurred by reading only from a single news source.
Clustered presentation can be contrasted with PBS in several ways.
1.
Unlike PBS whose task is search, the methods in clustered presentation are mainly focused on finding and presenting related news items to a piece of news at hand.
2.
Since clustered presentation is different from the well-established list view, using it for day-to-day tasks can harm the user experience and make the users confused.
3.
In clustered presentation, presenting the results in different clusters without any personalization can decrease the quality of results in the users’ day-to-day searches and thus reduce the user satisfaction.
4.
Unlike PBS that allows having lots of perspectives per search, allowing a large number of clusters in the clustered presentation may confuse the users and reduce understandability of the interface.
5.
In clustered presentation, not having specific names for clusters, as opposed to the perspectives in PBS, makes understanding their meanings and perceiving their viewpoints difficult.
5.3.5. Using intermediary topics
Two reasons for the creation of the information bubble are homophily (McPherson et al. 2001) and selective exposure (Sears and Freedman 1967; Frey 1986). In a social network context, users tend to connect with like-minded people and only read agreeable information. One approach to mitigate the information bubble is to recommend users with dissimilar viewpoints. However, this approach may provoke cognitive dissonance (Festinger 1957). Therefore, some studies (Graells-Garrido 2014, 2015; Graells-Garrido et al. 2014, 2015, 2016a; Bleackley 2017 and in Liao et al. (2015) in the context of a political forum) proposed using intermediary topics, i.e., topics that users with distant views on some sensitive issues have in common, to overcome the cognitive dissonance and provide opportunity for a connection.
This approach is different from PBS in two aspects.
1.
This approach to mitigate the information bubble is fundamentally different from PBS. The former aims to facilitate the formation of connections or communications between people of different beliefs/viewpoints, whereas the latter aims at bursting the information bubble in the search task.
2.
Unlike PBS, the decisions the system makes by using intermediary topics may not be transparent to the user, which may raise several ethical issues (see Bozdag and van den Hoven 2015; Dori-Hacohen et al. 2015).
5.3.6. Assisting users in mitigating the information bubble
Sometimes, a user wants to go out of the bubble and explore new ideas. Some methods facilitate this exploration. Taramigkou et al. (2013) proposed a methodology to help users explore new items that may be serendipitous and interesting to them. They implemented their methodology in a music recommender system. The general procedure of the method is as follows. The user selects the destination genre (identified by Latent Dirichlet Allocation (Blei et al. 2003)) and the system identifies the destination user who is a best match for the destination genre. Then, the shortest path between the user and the destination user is calculated and a predefined number of artists are selected per each node in the path. The list of artists is then presented to the user.
Bennett et al. (2015) considered the problem of searching on behalf of others, e.g., trying to find cool clothes for a teenage child. Although this approach does not pay attention to the information bubble, it can be considered a way of searching according to the interests of people other than the searcher, and thus a way of viewing the world from outside of the searcher’s information bubble. This approach is similar to using our demographic perspective; however, Bennett et al. (2015) did not provide a technical method for realizing on-behalf-of searches and focused on studying these searches according to Web search logs and characterizing this type of search.
Doris-Down et al. (2013) introduced a mobile application to bring people with different political viewpoints together for a cup of coffee and a face-to-face conversation to combat echo chambers.
These methods aim to help the users willing to go out of their bubbles, whereas PBS aims to raise user awareness of the bubbles and provide the required tools to mitigate them in searches.
5.3.7. Informing about disputed claims
One reason people may get trapped in bubbles is that they are not aware of the existence of other opinions and competing viewpoints. Thus, some systems aim to make the users more conscious of disputed claims and encourage them to judge the claims more critically. For example, Ennals et al. (2010) implemented a browser extension, called Dispute Finder, to alert users when the information they read online is disputed. They also proposed a popup interface with articles for and against the claim when a highlighted claim is clicked on. Rbutr14 is another extension that aims to tell users when the webpage they are viewing has been rebutted, disputed, or contradicted elsewhere on the Internet. Dori-Hacohen and Allan (2013) considered automatic identification of controversial webpages and suggested alerting users about controversial issues, for example, through a warning at the top of the webpage stating that “This webpage represents one of several perspectives on a controversial topic”. Also, Hypothes.is15 is an annotation tool that allows the users to add comments or critiques on webpages at the page-level or sentence-level and collaborate with others, which can help in improving the epistemic quality of information.
Moreover, sometimes a user is aware of controversy in an issue, but does not precisely know each group’s views on the issue and is unable to reach a conclusion. Kriplean et al. (2012) developed ConsiderIt, which invites the participants to create pro and con lists about issues. It also enables users to adopt the pros and cons contributed by others into their own lists. These lists can help everyone better explore and understand facets of issues. This approach can foster deliberation and promote more balanced civil discourse.
Kriplean et al. (2014) employed reference librarians to integrate an on-demand fact-checking service into a crowdsourced voters’ guide. Each user could request that a librarian fact-check a pro or con point contributed by another user and the librarians “… reviewed these requests, identified verifiable claims, researched the accuracy of those claims, and posted a public evaluation and report about each claim.”
This approach can be contrasted with PBS in several ways.
1.
Disputed claims are about specific events/phenomena, whereas perspectives in PBS can be much more general. For example, viewing the results from the viewpoint of a Republican is much more general than an opinion on a single topic like Obamacare.
2.
PBS is a solution to the existence of bubbles in search results, whereas informing about disputed claims is a solution to help users browsing pages on the Web or users seeking information about a special subject in finding more balanced information.
3.
This approach is limited to disputed claims, whereas PBS can be used for many other topics, e.g., searching the Web for poems from the perspective of a child.
4.
Ennals et al. (2010, p. 2) stated that “Fact checking web sites and search engines work well when you know something is disputed, but are of little use if you did not realize that what you were reading was disputed. The primary aim of Dispute Finder is to let you know when something you are reading is disputed.” However, using fact checking websites and dispute alerting tools is not widespread. On the other hand, PBS embeds alerts for users about other perspectives and allows them to control the results in search engines, which are a main source of information for people. This can make PBS much more effective in mitigating the information bubble compared with the disputed claims based solutions.
5.
Disputed claims (different beliefs) may be only one of the many possible perspectives in PBS.
6.
PBS allows users to find information from different perspectives, whereas filtering opinions according to different viewpoints (not only mentioned stances on the particular topic at hand) may not be possible in the informing about disputed claims approach.
5.4. Perspective-related concepts
Several concepts have been introduced in the previous works that have some similarities with our concept of perspective. In the following, we explain these concepts and discuss their differences with perspective.
5.4.1. Sentiment
Sentiment16 analysis is a salient domain of research in IR, which “deals with the computational treatment of opinion, sentiment, and subjectivity in text” (Pang and Lee 2008, p. 1). Several studies discussed in Section 5.3 (Demartini and Siersdorfer 2010; Zheng and Fang 2010; Demartini 2011; Kacimi and Gamper 2011, 2012; Aktolga and Allan 2013; Aktolga 2014; Giachanou et al. 2014; Graells-Garrido 2014; Liao and Fu 2014a) considered sentiment in their research. Because sentiment analysis is well-known in IR, we do not discuss it further here. For more information, readers are referred to Pang and Lee (2008).
Sentiment can be considered as only one of the many possible perspectives in PBS.
5.4.2. Controversy and stance
A lot of topics are controversial, i.e., there is not an obvious consensus among nearly all people. For example, many of religious and political issues are controversial. Some studies address the problem of detecting controversy or identifying stances (“an overall position held by a person toward an object, idea or proposition” (Somasundaran and Wiebe 2010, p. 1)) in a controversial topic. Dori-Hacohen et al. (2015) elaborated on this and discussed several related issues, including the definition of controversy and the related ethical concerns. Awadallah et al. (2012) presented OpinioNetIt, an opinion base of facets, opinion holders, and their opinions, which are automatically extracted from news and other web documents. OpinioNetIt includes information such as a politician’s position—support or opposition—on a controversial topic. Agrawal et al. (2003) addressed partitioning authors into opposite camps within a given topic. Park et al. (2011c) considered unsupervised classification of news articles based on disputant relations. They identified opposing disputant groups dynamically and classified the articles based on their positions. Paul et al. (2010) proposed a method for summarizing contrastive viewpoints in opinionated texts. Ren and de Rijke (2015) addressed the task of summarizing contrastive themes, in which they consider a viewpoint to be “a topic with a specific sentiment label” (p. 1), similar to Paul et al. (2010), and a theme as “a set of viewpoints around a specific set of topics and an explicit sentiment opinion” (p. 1). Somasundaran and Wiebe (2009, 2010) addressed classifying stances in product debates and ideological debates, respectively. Thomas et al. (2006) used transcripts of U.S. Congressional floor debates to determine whether the speeches represenedt opposition to or support of the proposed legislation.
Although sentiment analysis is related to controversy detection and stance analysis, they are not identical. Awadallah et al. (2012, p. 1) stated that: “… political controversies are much more complex and opinions are often expressed in subtle forms, which makes determining pro/con polarities much more difficult than with product reviews for cameras, movies, etc.—the typical objects in prior work on opinion mining.”
Our concept of perspective can be contrasted with the concepts of controversy and stance in several ways.
1.
Controversy and stance are mainly related to belief perspectives. PBS can support many other perspectives.
2.
In PBS, one can search a topic from a belief perspective, even when the topic is not controversial. For example, “prayer” in a query may refer to totally different concepts from the viewpoints of Muslims, Jews, or Christians. Moreover, one’s beliefs may be pertinent to apparently irrelevant characteristics and preferences, e.g., people with different political orientations may prefer different brands (Wing 2012) or have different personal characteristics (Pew Research Center 2014). These differences can be reflected in the search results when searched from different belief perspectives.
3.
Most works in this category do not focus on mitigating the information bubble in searches.
5.4.3. Aspects
Content producers view the same events from different viewpoints and select different aspects of reality. Several methods focus on identifying these aspects. Park et al. (2008, 2009, 2010, 2012) introduced NewsCube, an online news service aiming at mitigating the effect of media bias. NewsCube automatically clusters news according to their aspects and provides multiple viewpoints on a news event of interest to the readers (see Section 5.3.4). Park et al. (2011a) proposed NewsCube2.0 and exploited user collaboration to determine the viewpoints of and the framing17 methods used in the news articles. Using user collaboration, NewsCube2.0 can deal with various types of articles and different forms of framing methods, beyond straight news articles and fact selection, which were the focus of NewsCube. Chhabra and Resnick (2012) proposed CubeThat, which takes a similar approach to NewsCube but allows the user to change the cluster assignment of a news story. Similarly, Ma and Yoshikawa (2009) employed a clustered presentation of news to deliver diverse news reports on a certain news event. Meguebli et al. (2014a) and Kiritoshi and Ma (2014, 2015, 2016) implicitly considered aspects for diversifying news recommendations by exploiting semantic dissimilarity and sentiment dissimilarity measures. Kiritoshi and Ma (2014, 2015, 2016) employed a “difference in details” measure to consider the differences in the amount of details of news articles. Kacimi and Gamper (2011, 2012) employed a similar approach using semantic dissimilarity and sentiment dissimilarity for diversification in the search task. Kacimi and Gamper (2012) also provided a summary of opinions, including different sentiments and arguments, covered by the results of the search. On the other hand, Meguebli et al. (2014b) focused on determining users’ political orientations by comparing their aspects to the aspects extracted from Wikipedia pages.
This concept can be contrasted with our concept of perspective in several ways.
1.
Aspects are mainly related to belief perspectives. PBS can support many other perspectives.
2.
Works using aspects are mainly focused on the news domain and the task of recommendation.
3.
In PBS, the focus is on different viewpoints, whereas aspects focus on different dimensions of the topic at hand. For example, two documents may be categorized in two different aspects because they focus on different dimensions of the topic, despite having the same viewpoint (e.g., the liberal viewpoint).
4.
Aspects of the documents are determined (e.g., by clustering) according to the topic at hand, e.g., the news article being browsed or the query being searched. In PBS, some types of perspectives may be determined independently of the query, e.g., a document may be independently assigned to the Muslim perspective.
5.
The dissimilarity-based approach (diversifying or clustering according to dissimilarity measures) of most of the works using aspects can result in aspects without meaningful interpretations. In addition, obtaining well-defined labels representing aspects, which are needed in PBS to inform the user about the perspectives, may not be possible.
6.
In search, using the dissimilarity-based approach requires the extraction of aspects and the categorization of documents to be performed at the query, which can incur considerable performance overhead. In PBS, documents can be tagged with perspectives at indexing.
5.4.4. Ideological perspectives
Ideologies are reflected in people’s statements. For example, two editorials written by a Palestinian author and an Israeli author about the Israeli–Palestinian conflict are expected to be divergent and induce contradictory conclusions. Lin et al. (2006) addressed the problem of identifying ideological perspectives on the bitterlemons corpus,18 which includes editorials written by Israeli and Palestinian authors on the Israeli–Palestinian conflict. Lin and Hauptmann (2006) also considered the problem of determining whether two collections are written from different perspectives. Lin et al. (2008) further proposed a statistical model for determining ideological discourse in an unsupervised manner.
Identifying ideological perspectives is fundamentally different from sentiment analysis about a particular item. One’s sentiment is different for each item, while one’s perspective is probably more static, presumably underpinned by their ideologies or beliefs about the world (Lin et al. 2006).
This concept can be contrasted with our concept of perspective in several ways.
1.
Ideological perspectives are mainly related to belief perspectives. PBS can support many other perspectives.
2.
The methods of ideological perspectives are designed for articles with similar topics. PBS has articles with different topics in the collection.
3.
The methods in this category do not focus on mitigating the information bubble in a search, whereas PBS focuses on that goal.
4.
The methods in this category are mainly focused on only two ideological perspectives and scaling them for many perspectivesmay not be possible compared with the possibilities of PBS.
5.
Using the methods in this category for automatically extracting perspectives, one cannot necessarily obtain well-defined labels representing perspectives, which are needed in PBS to inform the user about the perspectives.
6.
The perspectives are mainly query-independent and depend on the corpus, e.g., being pro-Israel/pro-Palestine irrespective of the query, whereas PBS allows a different set of perspectives for each query.
5.4.5. Political orientations
Some works focused on political orientations (Table 1). For example, Kim and Lee (2014) considered the problem of determining the political orientations (liberal, conservative, and moderate) of news articles based on orientations of Twitter users. Oh et al. (2009) used SVM (Cortes and Vapnik 1995) and Naïve Bayes to classify political viewpoints of blog posts. Zhou et al. (2011) addressed the problem of classifying political leanings of news articles based on the assumption that conservative users vote for conservative articles more often, and similarly for liberal users and articles. Also, Kulshrestha et al. (2017) performed their analysis for quantifying search bias with respect to the Democratic and Republican parties. Park et al. (2011b) used commenters’ sentiment patterns for identifying political orientations of news stories. Meguebli et al. (2014b) proposed an unsupervised approach for identifying users’ political orientations based on a knowledge base extracted from Wikipedia.
Table 1.
Paper | Political orientations |
---|---|
Munson et al. 2013; Kim and Lee 2014 | Liberal/Conservative/moderate |
Munson et al. 2009a, 2009b; Munson and Resnick 2010; Munson 2012 | Liberal/Conservative/independent |
Oh et al. 2009; Zhou et al. 2011; Meguebli et al. 2014b | Liberal/Conservative |
Schwartz 2012 | Right/left |
Meguebli et al. 2014b; Kulshrestha et al. 2017 | Democratic/Republican parties |
Park et al. 2011b | Liberal/Democrat |
Meguebli et al. 2014b | Islamist/secular |
The border between the political orientation category and the ideological perspective category may not be obvious at first glance. We put methods having a generative nature in the ideological perspectives category, as ideology is somehow generative and can generate new thoughts. On the other hand, methods we put in the political orientations category have a discriminative nature, for example Oh et al. (2009) used SVM to classify political orientations.
This concept can be contrasted with our concept of perspective in several ways.
1.
Political orientations are mainly related to belief perspectives. PBS can support many other perspectives.
2.
Political orientations are limited (Table 1). There are plenty of other categorizations, e.g., Christian/non-Christian or vegetarian/nonvegetarian).
3.
The orientations are query independent. For example, the liberal/conservative categorization may not make sense in a technology-related search. PBS allows a different set of perspectives for each query.
4.
The studies in this category do not necessarily focus on mitigating the information bubble in a general-purpose search engine, as opposed to PBS. Many of them focus on news-related systems (e.g., news aggregators).
5.4.6. Document dimensions
A number of papers considered some dimensions of documents. For example, Aktolga (2014), Aktolga and Allan (2013), and Singh et al. (2013) considered the time dimension, i.e., time references mentioned within documents; Aktolga (2014) and Aktolga and Allan (2013) also considered the sentiment dimension and Singh et al. (2013) also considered the geographical dimension. Odijk (2016) considered the framing and time dimensions and focused on four generic news frames: conflict, human interest, economic consequences, and morality. Liao et al. (2015) used aspect indicators, i.e., interface features indicating the focused aspect of information (e.g., indicating whether the comment was about effectiveness or side effects of a medication) to mitigate selective exposure. Liao and Fu (2014a) also studied how source position indicators, which indicate positions of information sources (e.g., pro/con), impact user’s information-seeking behavior. They extended their approach by also considering source expertise indicators, i.e., “design features that explicitly distinguish information sources with higher competence” Liao and Fu (2014b, p. 1).
Several of the works in this category focused on tasks other than search, e.g., Liao et al. (2015) and Liao and Fu (2014a, 2014b) reported on systems for browsing comments. In addition, the dimensions are not necessarily used as viewpoints. For example, in Singh et al. (2013), time and geographical dimensions were used as filters that the user can use to dig into specific subsets of the search results. In contrast, in PBS, the focus is on the viewpoint. For instance, for the query “tourist attractions”, a document may not mention a geographical location, but is relevant to its corresponding perspective since, e.g., it mentions a tourist attraction near it.
5.4.7. Demographic factors of users
Another concept employed in previous works is demographics. For example, Graells-Garrido (2014), Graells-Garrido and Lalmas (2014), and Graells-Garrido et al. (2016b) considered geographic factors. Gossen (2015) considered age and focused on search engines for children. Also, Bennett et al. (2015) considered demographic groups (e.g., men over 50 years) and focused on searching on behalf of others.
Demographics can be considered as only one of the many possible perspective types in PBS. In addition, the works in this category do not necessarily have mitigating the information bubble in mind. For example, Gossen (2015) focused on search engines for children, whereas PBS allows a user (possibly an adult) to search on behalf of a child.
10
12
14
15
16
Merriam-Webster’s Online Dictionary: “an attitude, thought, or judgment prompted by feeling”.
17
To frame (Entman 1993, p. 2) is to “select some aspects of a perceived reality and make them more salient in a communicating text, in such a way as to promote a particular problem definition, causal interpretation, moral evaluation, and/or treatment recommendation for the item described”.
18
From bitterlemons.org/.
6. Conclusion and future work
In this paper, we proposed the PBS paradigm that aims at mitigating the information bubble in Web searches. The new paradigm suggests shedding light on the search topic by presenting existing perspectives to the user and giving the user control afterwards. PBS has also the benefit of enabling users to formulate their information needs more easily in some circumstances and obtain a higher recall. We discussed the strengths of PBS in Section 4. We also provided a survey of the related concepts and methods in Section 5.
We did not provide any specific implementation for PBS, although we provided some hints on implementing it. We brought up several research problems on implementing PBS in Section 3 and called for research on them.
Much needs to be done to realize a PBS system. In Section 6.1, we mention some important related research questions.
6.1. Future work
There are several questions regarding the implementation of a PBS system that are left open, awaiting further research.
–
A PBS system needs information related to perspectives. How to acquire the information is an important question for PBS implementation. We touched on how to acquire such information in Section 3.2. Each of the mentioned approaches may require more research. Determing the pros and cons of each of the possible sources of information is another research question.
–
How to find the perspectives relevant to a query and rank them is another research question. Although approaches like using the perspective tags assigned by users (e.g., through a folksonomy) to the search result pages as the relevant perspectives can be usable, they may not be sufficient for an industrial search engine. Thus, finding better methods with less reliance on manual tagging demands more research.
–
How to personalize the ranking of relevant perspectives according to the preferences and characteristics of the user is also another research problem. Not personalizing the relevant perspectives that are shown to users may result in showing perspectives that are not interesting to them and reduce their engagement in mitigating the information bubble.
–
Another research question is how to perform the search from a selected perspective. Some available methods may be sufficient for searching from some types of perspectives. For example, some personalized IR methods are usable for searching from the perspective of a selected user. However, finding well-performing methods for searching from other perspective types is an important research question.
There are also some questions related to the proposed paradigm as a whole that are worth studying. We briefly present our stances on them and provide our arguments from an analytical perspective. User-based experiments are needed to evaluate the validity of them in the real world.
–
Is PBS effective at mitigating the information bubble? We believe the answer to this question is positive. In PBS, users are made aware of the bubble problem, informed about the existence of different perspectives on the topic, and provided with tools to control which perspective to view the results from. This makes it superior to other approaches that provide only one or two of these benefits (e.g., see Section 5.3.3).
–
Are users interested in using PBS facilities? We believe the answer is conditionally yes. PBS is designed to have minimum intervention in users’ day-to-day search tasks. It takes into account that not all users in all situations and on all topics are interested in viewing the topic from different perspectives. Therefore, it does not force users to use its facilities (as opposed to, e.g., some diversification methods). It presents users different perspectives on their searches and gives them a chance to go out of their bubbles and experience new perspectives (in fact, it nudges them to do so by exposing them to other perspectives). If the user wants to have such an experience in a particular situation and on a particular topic, the user will probably be interested in using PBS facilities. Moreover, as mentioned earlier, PBS allows users to go deeper in their own bubbles and use PBS to focus more on their own perspectives.
–
Is there any drawback in using PBS for the user? Almost, no. PBS is designed to deliver an appropriate user experience and to not disturb users’ usual tasks. It allows the user to benefit from personalization in the search results (as opposed to, e.g., clustered presentation), while also having the possibility of observing different perspectives on the topic with minimum visual nuisance. It uses a list view of the results (see Fig. 2) and does not require much change in the well-established UI of search engines (as opposed to, e.g., clustered presentation). It also respects the user’s autonomy (as opposed to, e.g., the serendipity approach), and thus avoids several ethical concerns (see Bozdag and van den Hoven 2015; Dori-Hacohen et al. 2015). Moreover, its uniform approach (see Section 4.1.5) allows it to deliver benefits of different perspective types without much learning overhead on users. Also, in PBS, presenting the original (possibly personalized) results to users by default (as opposed to, e.g., some diversification methods) avoids conflicts of interest with search engine provider companies (see Section 4.1.4).
Acknowledgements
We would like to thank Mohammad Ali Tavallaei for sharing his creative thoughts with us and also Javid Dadashkarimi and Elahe Rabbani for their help in surveying related work.
References
Agrawal R, Rajagopalan S, Srikant R, and Xu Y. 2003. Mining newsgroups using networks arising from social behavior. In Proceedings of the 12th International Conference on World Wide Web, WWW ’03, Budapest, Hungary, 20–24 May 2003. pp. 529–535.
Aktolga E. 2014. Integrating non-topical aspects into information retrieval. Ph.D. thesis, University of Massachusetts Amherst, Amherst, Massachusetts.
Aktolga E, and Allan J. 2013. Sentiment diversification with different biases. In Proceedings of the 36th International ACM SIGIR Conference on Research and Development in Information Retrieval, SIGIR ’13, Dublin, Ireland, 28 July–1 August 2013. pp. 593–602.
An J, Quercia D, and Crowcroft J. 2013a. Fragmented social media: a look into selective exposure to political news. In Proceedings of the 22nd International Conference on World Wide Web, WWW ‘13 Companion, Rio de Janeiro, Brazil, 13–17 May 2013. pp. 51–52.
An J, Quercia D, and Crowcroft J. 2013b. Why individuals seek diverse opinions (or why they don’t). In Proceedings of the 5th Annual ACM Web Science Conference, WebSci ’13, Paris, France, 2–4 May 2013. pp. 15–18.
Awadallah R, Ramanath M, and Weikum G. 2012. Harmony and dissonance: organizing the people’s voices on political controversies. In Proceedings of the Fifth ACM International Conference on Web Search and Data Mining, WSDM ’12, Seattle, Washington, 8–12 February 2012. pp. 523–532.
Bechmann A, and Nielbo KL. 2018. Are we exposed to the same “news” in the news feed? Digital Journalism, 6(8): 990–1002.
Bennett P, Fishkov A, and Kıcıman E. 2015. Persona-ization: searching on behalf of others. In Proceedings of the SIGIR 2015 International Workshop on Social Personalisation & Search (SPS’15), Santiago, Chile, 9–13 August 2015. pp. 26–31.
Berman R, and Katona Z. 2018. Curation algorithms and filter bubbles in social networks. Technical Report. NET Institute Working Paper No. 16-08.
Bernardini A, Carpineto C, and D’Amico M. 2009. Full-subtopic retrieval with keyphrase-based search results clustering. In 2009 IEEE/WIC/ACM International Joint Conference on Web Intelligence and Intelligent Agent Technology, WI-IAT ’09, Milan, Italy, 15–18 September 2009. Vol. 1, pp. 206–213.
Bex F, Lawrence J, Snaith M, and Reed C. 2013. Implementing the argument web. Communications of the ACM, 56(10): 66–73.
Bleackley P. 2017. CommonGround: an algorithm to bypass filter bubbles [online]: Available from sourceforge.net/projects/commonground/.
Blei DM, and Lafferty JD. 2009. Topic models. In Text mining: classification, clustering, and applications. Edited by AN Srivastava and M Sahami. Chapman and Hall/CRC, New York, New York. pp. 101–124.
Blei DM, Ng AY, and Jordan MI. 2003. Latent Dirichlet allocation. Journal of Machine Learning Research, 3: 993–1022.
Bouadjenek MR, Hacid H, and Bouzeghoub M. 2016. Social networks and information retrieval, how are they converging? A survey, a taxonomy and an analysis of social information retrieval approaches and platforms. Information Systems, 56: 1–18.
Bozdag E. 2015. Bursting the filter bubble: democracy, design, and ethics. Ph.D. thesis, Delft University of Technology, Delft, the Netherlands.
Bozdag E, and Timmermans J. 2011. Values in the filter bubble ethics of personalization algorithms in cloud computing. In 1st International Workshop on Values in Design—Building Bridges between RE, HCI and Ethics, Lisbon, Portugal, 6 September 2011. pp. 7–15.
Bozdag E, and van den Hoven J. 2015. Breaking the filter bubble: democracy and design. Ethics and Information Technology, 17(4): 249–265.
Carpineto C, D’Amico M, and Romano G. 2012. Evaluating subtopic retrieval methods: clustering versus diversification of search results. Information Processing & Management, 48(2): 358–373.
Carpineto C, Osiński S, Romano G, and Weiss D. 2009. A survey of web clustering engines. ACM Computing Surveys, 41(3): 17.
Carson AB. 2015. Public discourse in the age of personalization: psychological explanations and political implications of search engine bias and the filter bubble. Journal of Science Policy & Governance, 7(1): 1–13.
Cavoukian A, and Chibba M. 2018. Start with privacy by design in all big data applications. In Guide to big data applications. Edited by S Srinivasan. Springer International Publishing, Cham, Switzerland. Chapter 2, pp. 29–48.
Chesñevar C, McGinnis J, Modgil S, Rahwan I, Reed C, Simari G, et al. 2006. Towards an argument interchange format. The Knowledge Engineering Review, 21(4): 293–316.
Chhabra S, and Resnick P. 2012. CubeThat: news article recommender. In Proceedings of the Sixth ACM Conference on Recommender Systems, RecSys ’12, Dublin, Ireland, 9–13 September 2012. pp. 295–296.
Chhabra S, and Resnick P. 2013. Does clustered presentation lead readers to diverse selections? In CHI ‘13 Extended Abstracts on Human Factors in Computing Systems, CHI EA ’13, Paris, France, 27 April–2 May 2013. pp. 1689–1694.
Cortes C, and Vapnik V. 1995. Support-vector networks. Machine Learning, 20(3): 273–297.
Dang VB. 2014. A proportionality-based approach to search result diversification. Ph.D. thesis, University of Massachusetts Amherst, Amherst, Massachusetts.
Davies HC. 2018. Redefining filter bubbles as (escapable) socio-technical recursion. Sociological Research Online, 23(3): 637–654.
Demartini G. 2011. ARES: a retrieval engine based on sentiments. In Advances in Information Retrieval: 33rd European Conference on IR Research, ECIR 2011, Dublin, Ireland, 18–21 April 2011. pp. 772–775.
Demartini G, and Siersdorfer S. 2010. Dear search engine: what’s your opinion about…?: sentiment analysis for semantic enrichment of web search results. In Proceedings of the 3rd International Semantic Search Workshop, SEMSEARCH ’10, Raleigh, North Carolina, 26 April 2010. Article No. 4.
Dori-Hacohen S. 2015. Controversy detection and stance analysis (full 5-page version) [online]: Available from https://sites.google.com/a/dori-hacohen.com/shiri/publications.
Dori-Hacohen S. 2017. Controversy analysis and detection. Ph.D. thesis, University of Massachusetts Amherst, Amherst, Massachusetts.
Dori-Hacohen S, and Allan J. 2013. Detecting controversy on the web. In Proceedings of the 22nd ACM International Conference on Information & Knowledge Management, CIKM ’13, San Francisco, California, 27 October–1 November 2013. pp. 1845–1848.
Dori-Hacohen S, Yom-Tov E, and Allan J. 2015. Navigating controversy as a complex search task. In ECIR Supporting Complex Search Task Workshop, Vienna, Austria, 29 March 2015.
Doris-Down A, Versee H, and Gilbert E. 2013. Political blend: an application designed to bring people together based on political differences. In Proceedings of the 6th International Conference on Communities and Technologies, C&T ’13, Munich, Germany, 29 June–2 July 2013. pp. 120–130.
Dutton WH, and Fernandez L. 2019. How susceptible are Internet users? Intermedia, 46(4): 36–40.
El-Arini K, Paquet U, Herbrich R, Van Gael J, and Agüera y Arcas B. 2012. Transparent user models for personalization. In Proceedings of the 18th ACM SIGKDD International Conference on Knowledge Discovery and Data Mining, KDD ’12, Beijing, China, 12–16 August 2012. pp. 678–686.
Ennals R, Trushkowsky B, and Agosta JM. 2010. Highlighting disputed claims on the web. In Proceedings of the 19th International Conference on World Wide Web, WWW ’10, Raleigh, North Carolina, 26–30 April 2010. pp. 341–350.
Entman RM. 1993. Framing: toward clarification of a fractured paradigm. Journal of Communication, 43(4): 51–58.
Eslami M, Rickman A, Vaccaro K, Aleyasen A, Vuong A, Karahalios K, et al. 2015. “I always assumed that I wasn’t really that close to [her]”: reasoning about invisible algorithms in news feeds. In Proceedings of the 33rd Annual ACM Conference on Human Factors in Computing Systems, CHI ’15, Seoul, Republic of Korea, 18–23 April 2015. pp. 153–162.
Ferragina P, and Gullì A. 2004. The anatomy of SnakeT: a hierarchical clustering engine for web-page snippets. In Proceedings of the 8th European Conference on Principles and Practice of Knowledge Discovery in Databases, PKDD ’04, Pisa, Italy, 20–24 September 2004. pp. 506–508.
Festinger L. 1957. A theory of cognitive dissonance. Row, Peterson & Company, Evanston, Illinois.
Frey D. 1986. Recent research on selective exposure to information. Advances in Experimental Social Psychology, 19: 41–80.
Gao M, Do HJ, and Fu W-T. 2018. Burst your bubble! An intelligent system for improving awareness of diverse social opinions. In 23rd International Conference on Intelligent User Interfaces, IUI ’18, Tokyo, Japan, 7–11 March 2018. pp. 371–383.
Garrett RK. 2013. Selective exposure: new methods and new directions. Communication Methods and Measures, 7(3–4): 247–256.
Garrett RK, and Resnick P. 2011. Resisting political fragmentation on the Internet. Daedalus, 140(4): 108–120.
Ghorab MR, Zhou D, O’Connor A, and Wade V. 2013. Personalised information retrieval: survey and classification. User Modeling and User-Adapted Interaction, 23(4): 381–443.
Giachanou A, Markov I, and Crestani F. 2014. Opinions in federated search: University of lugano at TREC 2014 federated web search track. In Proceedings of the Twenty-Third Text Retrieval Conference, TREC 2014, Gaithersburg, Maryland, 19–21 November 2014.
Gossen T. 2015. Targeted search engines for children: search user interfaces and information-seeking behaviour. Ph.D. thesis, Otto von Guericke University Magdeburg, Magdeburg, Germany.
Graells-Garrido E. 2014. Enhancing web activities with information visualization. In Proceedings of the 23rd International Conference on World Wide Web, WWW ‘14 Companion, Seoul, Korea, 7–11 April 2014. pp. 39–44.
Graells-Garrido E. 2015. Biased behavior in web activities: from understanding to unbiased visual exploration. Ph.D. thesis, Universitat Pompeu Fabra, Barcelona, Spain.
Graells-Garrido E, and Lalmas M. 2014. Balancing diversity to counter-measure geographical centralization in microblogging platforms. In Proceedings of the 25th ACM Conference on Hypertext and Social Media, HT ’14, Santiago, Chile, 1–4 September 2014. pp. 231–236.
Graells-Garrido E, Lalmas M, and Quercia D. 2014. People of opposing views can share common interests. In Proceedings of the 23rd International Conference on World Wide Web, WWW ‘14 Companion, Seoul, Korea, 7–11 April 2014. pp. 281–282.
Graells-Garrido E, Lalmas M, and Baeza-Yates R. 2015. Finding intermediary topics between people of opposing views: a case study. In International Workshop on Social Personalisation & Search, SPS2015, Santiago, Chile, 9–13 August 2015. pp. 13–18.
Graells-Garrido E, Lalmas M, and Baeza-Yates R. 2016a. Data portraits and intermediary topics: encouraging exploration of politically diverse profiles. In Proceedings of the 21st International Conference on Intelligent User Interfaces, Sonoma, California, 7–10 March 2016. pp. 228–240.
Graells-Garrido E, Lalmas M, and Baeza-Yates R. 2016b. Encouraging diversity- and representation-awareness in geographically centralized content. In Proceedings of the 21st International Conference on Intelligent User Interfaces, IUI ‘16, Sonoma, California, 7–10 March 2016. pp. 7–18.
Guha RV. 2013. Light at the end of the tunnel [online]: Available from iswc2013.semanticweb.org/content/keynote-ramanathan-v-guha.html.
Han J, Kamber M, and Pei J. 2011. Data mining: concepts and techniques. 3rd edition. Morgan Kaufmann, Waltham, Massachusetts.
Hearst MA. 2006. Clustering versus faceted categories for information exploration. Communications of the ACM, 49(4): 59–61.
Iskold A. 2007a. Overview of clustering and Clusty search engine [online]: Available from readwrite.com/2007/01/05/overview_of_clu/.
Iskold A. 2007b. Semantic web: difficulties with the classic approach [online]: Available from readwrite.com/2007/09/19/semantic_web_difficulties_with_classic_approach/.
Iskold A. 2007c. Top-down: a new approach to the semantic web [online]: Available from readwrite.com/2007/09/20/the_top-down_semantic_web/.
Kacimi M, and Gamper J. 2011. Diversifying search results of controversial queries. In Proceedings of the 20th ACM International Conference on Information and Knowledge Management, CIKM ’11, Glasgow, UK, 24–28 October 2011. pp. 93–98.
Kacimi M, and Gamper J. 2012. MOUNA: mining opinions to unveil neglected arguments. In Proceedings of the 21st ACM International Conference on Information and Knowledge Management, CIKM ’12, Maui, Hawaii, 29 October–2 November 2012. pp. 2722–2724.
Kim JG, and Lee KS. 2014. Predicting political orientation of news articles based on user behavior analysis in social network. IEICE Transactions on Information and Systems, E97-D(4): 685–693.
Kiritoshi K, and Ma Q. 2014. Named entity oriented related news ranking. In Database and Expert Systems Applications: 25th International Conference, DEXA 2014, Munich, Germany, 1–4 September 2014, Proceedings, Part II. pp. 82–96.
Kiritoshi K, and Ma Q. 2015. A diversity-seeking mobile news app based on difference analysis of news articles. In Database and Expert Systems Applications: 26th International Conference, DEXA 2015, Valencia, Spain, 1–4 September 2015, Proceedings, Part II. pp. 73–81.
Kiritoshi K, and Ma Q. 2016. Named entity oriented difference analysis of news articles and its application. IEICE Transactions on Information and Systems, E99-D(4): 906–917.
Kriplean T, Morgan J, Freelon D, Borning A, and Bennett L. 2012. Supporting reflective public thought with considerit. In Proceedings of the ACM 2012 Conference on Computer Supported Cooperative Work, CSCW ’12, Seattle, Washington, 11–15 February 2012. pp. 265–274.
Kriplean T, Bonnar C, Borning A, Kinney B, and Gill B. 2014. Integrating on-demand fact-checking with public dialogue. In Proceedings of the 17th ACM Conference on Computer Supported Cooperative Work & Social Computing, CSCW ’14, Baltimore, Maryland, 15–19 February 2014. pp. 1188–1199.
Kulshrestha J, Eslami M, Messias J, Zafar MB, Ghosh S, Gummadi KP, et al. 2017. Quantifying search bias: investigating sources of bias for political searches in social media. In Proceedings of the 2017 ACM Conference on Computer Supported Cooperative Work and Social Computing, CSCW ’17, Portland, Oregon, 25 February–1 March 2017. pp. 417–432.
Li D, and Huan L. 2008. The ontology relation extraction for semantic web annotation. In 2008 Eighth IEEE International Symposium on Cluster Computing and the Grid (CCGRID), Lyon, France, 19–22 May 2008. pp. 534–541.
Liao QV, and Fu W-T. 2013. Beyond the filter bubble: interactive effects of perceived threat and topic involvement on selective exposure to information. In Proceedings of the SIGCHI Conference on Human Factors in Computing Systems, CHI ’13, Paris, France, 27 April–2 May 2013. pp. 2359–2368.
Liao QV, and Fu W-T. 2014a. Can you hear me now?: mitigating the echo chamber effect by source position indicators. In Proceedings of the 17th ACM Conference on Computer Supported Cooperative Work & Social Computing, CSCW ’14, Baltimore, Maryland, 15–19 February 2014. pp. 184–196.
Liao QV, and Fu W-T. 2014b. Expert voices in echo chambers: effects of source expertise indicators on exposure to diverse opinions. In Proceedings of the SIGCHI Conference on Human Factors in Computing Systems, CHI ’14, Toronto, Ontario, 26 April–1 May 2014. pp. 2745–2754.
Liao QV, Fu W-T, and Mamidi SS. 2015. It is all about perspective: an exploration of mitigating selective exposure with aspect indicators. In Proceedings of the 33rd Annual ACM Conference on Human Factors in Computing Systems, CHI ’15, Seoul, Republic of Korea, 18–23 April 2015. pp. 1439–1448.
Lin W-H, and Hauptmann A. 2006. Are these documents written from different perspectives?: a test of different perspectives based on statistical distribution divergence. In Proceedings of the 21st International Conference on Computational Linguistics and the 44th Annual Meeting of the Association for Computational Linguistics, ACL-44, Sydney, Australia, 17–18 July 2006. pp. 1057–1064.
Lin W-H, Wilson T, Wiebe J, and Hauptmann A. 2006. Which side are you on?: identifying perspectives at the document and sentence levels. In Proceedings of the Tenth Conference on Computational Natural Language Learning, CoNLL-X ’06, New York City, New York, 8–9 June 2006. pp. 109–116.
Lin W-H, Xing E, and Hauptmann A. 2008. A joint topic and perspective model for ideological discourse. In Proceedings of the European Conference on Machine Learning and Knowledge Discovery in Databases, ECML PKDD ’08, Antwerp, Belgium, 15–19 September 2008, Proceedings, Part II. pp. 17–32.
Ma Q, and Yoshikawa M. 2009. Topic and viewpoint extraction for diversity and bias analysis of news contents. In Advances in Data and Web Management: Joint International Conferences, APWeb/WAIM 2009, Suzhou, China, 2–4 April 2009, Proceedings. pp. 150–161.
Maccatrozzo V. 2012. Burst the filter bubble: using semantic web to enable serendipity. In Proceedings of the 11th International Semantic Web Conference, Boston, Massachusetts, 11–15 November 2012, Proceedings, Part II. pp. 391–398.
Maccatrozzo V, Terstall M, Aroyo L, and Schreiber G. 2017. SIRUP: serendipity in recommendations via user perceptions. In Proceedings of the 22nd International Conference on Intelligent User Interfaces, IUI ’17, Limassol, Cyprus, 13–16 March 2017. pp. 35–44.
McPherson M, Smith-Lovin L, and Cook JM. 2001. Birds of a feather: homophily in social networks. Annual Review of Sociology, 27(1): 415–444.
Meguebli Y, Kacimi M, Doan B-L, and Popineau F. 2014a. Stories around you—a two-stage personalized news recommendation. In International Conference on Knowledge Discovery and Information Retrieval (KDIR 2014), Rome, Italy, 21–24 October 2014. pp. 473–479.
Meguebli Y, Kacimi M, Doan B-L, and Popineau F. 2014b. Unsupervised approach for identifying users’ political orientations. In Proceedings of the 36th European Conference on IR Research on Advances in Information Retrieval, ECIR 2014, Amsterdam, the Netherlands, 13–16 April 2014, Proceedings. Vol. 8416, pp. 507–512.
Moeller J, and Helberger N. 2018. Beyond the filter bubble: concepts, myths, evidence and issues for future debates. Technical Report. University of Amsterdam, Amsterdam, the Netherlands [online]: Available from hdl.handle.net/11245.1/478edb9e-8296-4a84-9631-c7360d593610.
Morris JS, and Francia PL. 2010. Cable news, public opinion, and the 2004 party conventions. Political Research Quarterly, 63(4): 834–849.
Munson SA. 2012. Exposure to political diversity online. Ph.D. thesis, University of Michigan, Ann Arbor, Michigan.
Munson SA, and Resnick P. 2010. Presenting diverse political opinions: how and how much. In Proceedings of the SIGCHI Conference on Human Factors in Computing Systems, CHI ’10, Atlanta, Georgia, 10–15 April 2010. pp. 1457–1466.
Munson SA, Zhou DX, and Resnick P. 2009a. Designing interfaces for presentation of opinion diversity. In CHI ‘09 Extended Abstracts on Human Factors in Computing Systems, CHI EA ’09, Boston, Massachusetts, 4–9 April 2009. pp. 3667–3672.
Munson SA, Zhou DX, and Resnick P. 2009b. Sidelines: an algorithm for increasing diversity in news and opinion aggregators. In Proceedings of the Third International Conference on Weblogs and Social Media, ICWSM 2009, San Jose, California, 17–20 May 2009.
Munson SA, Lee SY, and Resnick P. 2013. Encouraging reading of diverse political viewpoints with a browser widget. In Proceedings of the Seventh International AAAI Conference on Weblogs and Social Media, Boston, Massachusetts, 8–11 July 2013. pp. 419–428.
Nagulendra S, and Vassileva J. 2014. Understanding and controlling the filter bubble through interactive visualization: a user study. In Proceedings of the 25th ACM Conference on Hypertext and Social Media, HT ’14, Santiago, Chile, 1–4 September 2014. pp. 107–115.
Negroponte N. 1995. Being digital. 1st edition. Hodder & Stoughton, London, UK.
Nguyen TT, Hui P-M, Harper FM, Terveen L, and Konstan JA. 2014. Exploring the filter bubble: the effect of using recommender systems on content diversity. In Proceedings of the 23rd International Conference on World Wide Web, WWW ’14, Seoul, Korea, 7–11 April 2014. pp. 677–686.
Odijk D. 2016. Context & semantics in news & web search. ACM SIGIR Forum, 50(1): 93–94.
Oh A, Lee H, and Kim Y. 2009. User evaluation of a system for classifying and displaying political viewpoints of weblogs. In Proceedings of the Third International Conference on Weblogs and Social Media, ICWSM 2009, San Jose, California, 17–20 May 2009. pp. 282–285.
Osinski S, and Weiss D. 2005. A concept-driven algorithm for clustering search results. IEEE Intelligent Systems, 20(3): 48–54.
Osinski S, and Weiss D. 2007. Clustering search results with carrot2 [online]: Available from project.carrot2.org/publications/carrot2-dresden-2007.pdf.
Pang B, and Lee L. 2008. Opinion mining and sentiment analysis. Foundations and Trends® in Information Retrieval, 2(1–2): 1–135.
Pariser E. 2011. The filter bubble: what the Internet is hiding from you. The Penguin Group, New York, New York.
Park S, Kang S, Lee S, Chung S, and Song J. 2008. Mitigating media bias: a computational approach. In Proceedings of the Hypertext 2008 Workshop on Collaboration and Collective Intelligence, WebScience ’08, Pittsburgh, Pennsylvania, 19–21 June 2008. pp. 47–51.
Park S, Kang S, Chung S, and Song J. 2009. NewsCube: delivering multiple aspects of news to mitigate media bias. In Proceedings of the SIGCHI Conference on Human Factors in Computing Systems, CHI ’09, Boston, Massachusetts, 4–9 April 2009. pp. 443–452.
Park S, Lee S, and Song J. 2010. Aspect-level news browsing: understanding news events from multiple viewpoints. In Proceedings of the 15th International Conference on Intelligent User Interfaces, IUI ’10, Hong Kong, China, 7–10 February 2010. pp. 41–50.
Park S, Ko M, Kim J, Choi HJ, and Song J. 2011a. NewsCube2.0: an exploratory design of a social news website for media bias mitigation. In 2nd International Workshop on Social Recommender Systems, Hangzhou, China, 19 March 2011.
Park S, Ko M, Kim J, Liu Y, and Song J. 2011b. The politics of comments: predicting political orientation of news stories with commenters’ sentiment patterns. In Proceedings of the ACM 2011 Conference on Computer Supported Cooperative Work, CSCW ’11, Hangzhou, China, 19–23 March 2011. pp. 113–122.
Park S, Lee K, and Song J. 2011c. Contrasting opposing views of news articles on contentious issues. In Proceedings of the 49th Annual Meeting of the Association for Computational Linguistics: Human Language Technologies—Volume 1, HLT ’11, Portland, Oregon, 19–24 June 2011. pp. 340–349.
Park S, Kang S, Chung S, and Song J. 2012. A computational framework for media bias mitigation. ACM Transactions on Interactive Intelligent Systems, 2(2): 8.
Park S, Ko M, Lee J, and Song J. 2013. Agenda diversity in social media discourse: a study of the 2012 Korean general election. In Proceedings of the Seventh International AAAI Conference on Weblogs and Social Media, Boston, Massachusetts, 8–11 July 2013. pp. 486–495.
Pasi G. 2010. Issues in personalizing information retrieval. IEEE Intelligent Informatics Bulletin, 11(1): 3–7.
Paul MJ, Zhai C, and Girju R. 2010. Summarizing contrastive viewpoints in opinionated text. In Proceedings of the 2010 Conference on Empirical Methods in Natural Language Processing, EMNLP ’10, Cambridge, Massachusetts, 9–11 October 2010. pp. 66–76.
Pew Research Center. 2014. Political polarization in the American public. Survey report. Pew Research Center, Washington, DC.
Ren Z, and de Rijke M. 2015. Summarizing contrastive themes via hierarchical non-parametric processes. In Proceedings of the 38th International ACM SIGIR Conference on Research and Development in Information Retrieval, SIGIR ’15, Santiago, Chile, 9–13 August 2015. pp. 93–102.
Resnick P. 2011. Personalized filters yes; bubbles no [online]: Available from presnick.wordpress.com/2011/07/17/personalized-filters-yes-bubbles-no/.
Resnick P, Garrett RK, Kriplean T, Munson SA, and Stroud NJ. 2013. Bursting your (filter) bubble: strategies for promoting diverse exposure. In Proceedings of the 2013 Conference on Computer Supported Cooperative Work Companion, CSCW ’13, San Antonio, Texas, 23–27 February 2013. pp. 95–100.
Santos RLT, Macdonald C, and Ounis I. 2015. Search result diversification. Foundations and Trends® in Information Retrieval, 9(1): 1–90.
Schwartz B. 2012. Bing launched elections portal: filter news by party, social integration, maps & more [online]: Available from searchengineland.com/bing-launched-elections-portal-filter-news-by-party-social-integration-maps-more-137681.
Sears DO, and Freedman JL. 1967. Selective exposure to information: a critical review. Public Opinion Quarterly, 31(2): 194–213.
Singh R, Hsu Y-W, and Moon N. 2013. Multiple perspective interactive search: a paradigm for exploratory search and information retrieval on the web. Multimedia Tools and Applications, 62(2): 507–543.
Somasundaran S, and Wiebe J. 2009. Recognizing stances in online debates. In Proceedings of the Joint Conference of the 47th Annual Meeting of the ACL and the 4th International Joint Conference on Natural Language Processing of the AFNLP: Volume 1, ACL ’09, Suntec, Singapore, 2–7 August 2009. pp. 226–234.
Somasundaran S, and Wiebe J. 2010. Recognizing stances in ideological on-line debates. In Proceedings of the NAACL HLT 2010 Workshop on Computational Approaches to Analysis and Generation of Emotion in Text, CAAGET ’10, Los Angeles, California, 5 June 2010. pp. 116–124.
Steichen B, Ashman H, and Wade V. 2012. A comparative survey of personalised information retrieval and adaptive hypermedia techniques. Information Processing & Management, 48(4): 698–724.
Stevenson M, and Ciravegna F. 2003. Information extraction as a semantic web technology: requirements and promises. In Proceedings of the 14th European Conference on Machine Learning (ECML-03), Workshop on Adaptive Text Extraction and Mining (ATEM03) held with ECML/PKDD, Cavtat-Dubrovnik, Croatia, 22 September 2003. pp. 74–78.
Stracke J. 2014. User control of search filter bubble. US Patent 8,886,644.
Sunstein CR. 2001a. Echo chambers: Bush v. Gore, impeachment, and beyond. Princeton University Press, Princeton, New Jersey.
Sunstein CR. 2001b. Republic.com. Princeton University Press, Princeton, New Jersey.
Sunstein CR. 2007. Republic.com 2.0. Princeton University Press, Princeton, New Jersey.
Sunstein CR. 2009. Going to extremes: how like minds unite and divide. Oxford University Press, New York, New York.
Taramigkou M, Bothos E, Christidis K, Apostolou D, and Mentzas G. 2013. Escape the bubble: guided exploration of music preferences for serendipity and novelty. In Proceedings of the 7th ACM Conference on Recommender Systems, RecSys ’13, Hong Kong, China, 12–16 October 2013. pp. 335–338.
Teevan J, and Yu L. 2017. Bringing the wisdom of the crowd to an individual by having the individual assume different roles. In Proceedings of the 2017 ACM SIGCHI Conference on Creativity and Cognition, C&C ’17, Singapore, Singapore, 27–30 June 2017. pp. 131–135.
Thomas M, Pang B, and Lee L. 2006. Get out the vote: determining support or opposition from congressional floor-debate transcripts. In Proceedings of the 2006 Conference on Empirical Methods in Natural Language Processing, EMNLP ’06, Sydney, Australia, 22–23 July 2006. pp. 327–335.
Tran GB, and Herder E. 2015. Detecting filter bubbles in ongoing news stories. In Proceedings of the 23rd Conference on User Modeling, Adaptation and Personalization, Dublin, Ireland, June 29–July 3 2015.
Tunkelang D. 2009. Faceted search. Morgan & Claypool Publishers, San Rafael, California.
von Ahn L. 2006. Games with a purpose. Computer, 39(6): 92–94.
Wang D, Li L, and Li T. 2015. NewsCubeSum: a personalized multidimensional news update summarization system. In 2015 IEEE 14th International Conference on Machine Learning and Applications (ICMLA), Miami, Florida, 9–11 December 2015. pp. 981–986.
Wei B, Liu J, Zheng Q, Zhang W, Fu X, and Feng B. 2013. A survey of faceted search. Journal of Web Engineering, 12(1–2): 41–64.
White RW, and Hassan A. 2014. Content bias in online health search. ACM Transactions on the Web, 8(4): 25.
Wing N. 2012. Buyology Inc. report shows what brands democrats, republicans prefer [online]: Available from huffingtonpost.com/2012/06/18/buyology-inc-report-democrats-republicans_n_1605795.html.
Yom-Tov E, Dumais S, and Guo Q. 2014. Promoting civil discourse through search engine diversity. Social Science Computer Review, 32(2): 145–154.
Zelevinsky V. 2010. Breaking down the assumptions of faceted search. In Proceedings of the Fourth Workshop on Human-Computer Interaction and Information Retrieval, New Brunswick, New Jersey, 22 August 2010. pp. 126–128.
Zhai CX. 2008. Statistical language models for information retrieval. Morgan & Claypool Publishers, San Rafael, California.
Zheng W, and Fang H. 2010. A retrieval system based on sentiment analysis. In Proceedings of the Fourth Workshop on Human-Computer Interaction and Information Retrieval, New Brunswick, New Jersey, 22 August 2010. pp. 52–54.
Zhou DX, Resnick P, and Mei Q. 2011. Classifying the political leaning of news articles and users from user votes. In Proceedings of the Fifth International AAAI Conference on Weblogs and Social Media, Barcelona, Catalonia, Spain, 17–21 July 2011. pp. 417–424.
Information & Authors
Information
Published In

FACETS
Volume 4 • Number 1 • June 2019
Pages: 350 - 388
Editor: AMM Sharif Ullah
History
Received: 17 January 2019
Accepted: 24 April 2019
Version of record online: 5 August 2019
Copyright
© 2019 Tabrizi and Shakery. This work is licensed under a Creative Commons Attribution 4.0 International License (CC BY 4.0), which permits unrestricted use, distribution, and reproduction in any medium, provided the original author(s) and source are credited.
Data Availability Statement
All relevant data are within the paper.
Key Words
Sections
Subjects
Authors
Author Contributions
SAT conceived and designed the study.
AS drafted or revised the manuscript.
Competing Interests
We have filed a US patent related to the proposed paradigm. Despite the similarities, we believe that the patent does not interfere with the publication of this article for the following reasons. (a) At the time of submitting this manuscript, the patent was published but was not examined. (b) This article is written from a theoretical perspective and focuses on the paradigm, its importance, related research questions, and why it is an appropriate solution, although we have provided some hints on implementing the paradigm. The patent is written from an implementational perspective and focuses on how to implement the paradigm. (c) The paper includes an extensive survey of the related methods and concepts which accounts for roughly half of the paper. The patent lacks such a survey.
Metrics & Citations
Metrics
Other Metrics
Citations
Cite As
Shayan A. Tabrizi and Azadeh Shakery. 2019. Perspective-based search: a new paradigm for bursting the information bubble. FACETS.
4(1): 350-388. https://doi.org/10.1139/facets-2019-0002
Export Citations
If you have the appropriate software installed, you can download article citation data to the citation manager of your choice. Simply select your manager software from the list below and click Download.
Cited by
1. A Systematic Review of Fairness, Accountability, Transparency, and Ethics in Information Retrieval
2. Knowledge mapping of information cocoons: A bibliometric study using visual analysis
3. Generation Mechanism of “Information Cocoons” of Network Users: An Evolutionary Game Approach
4. Understanding the “Pathway” Towards a Searcher’s Learning Objective
5. We the swarm—Methodological, theoretical, and societal (r)evolutions in collective decision-making research
6. Turning Filter Bubbles into Bubblesphere with
Multi‐Viewpoint KOS
and Diverse Similarity