Time to refine mercury mass balance models for fish
Abstract
Mercury mass balance models (MMBMs) for fish are powerful tools for understanding factors affecting growth and food consumption by free-ranging fish in rivers, lakes, and oceans. Moreover, MMBMs can be used to predict the consequences of global mercury reductions, overfishing, and climate change on mercury (Hg) concentration in commercially and recreationally valuable species of fish. Such predictions are useful in decision-making by resource managers and public health policy makers, because mercury is a neurotoxin and the primary route of exposure of mercury to humans is via consumption of fish. Recent evidence has emerged to indicate that the current-day version of MMBMs overestimates the rate at which fish eliminate mercury from their bodies. Consequently, MMBMs overestimate food consumption by fish and underestimate Hg concentration in fish. In this perspective, we explore underlying reasons for this overestimation of Hg-elimination rate, as well as consequences and implications of this overestimation. We highlight emerging studies that distinguish species and sex as contributing factors, in addition to body weight and water temperature, that can play an important role in how quickly Hg is eliminated from fish. Future research directions for refining MMBMs are discussed.
1. Introduction
Although mercury is a naturally occurring element on the planet Earth, human activities, especially the burning of coal, have led to widespread mercury contamination of terrestrial and aquatic ecosystems (Boening 2000; Driscoll et al. 2013). Once deposited to aquatic ecosystems, inorganic mercury can be transformed to methylmercury (MeHg), which readily biomagnifies in aquatic food webs to levels in fish muscle tissue that pose a threat to human health (Clarkson et al. 2003; Sandheinrich et al. 2011; Wiener et al. 2012; Rice et al. 2014). The primary route of exposure of mercury to humans is through consumption of fish (Boening 2000; Driscoll et al. 2013). The predominant form of mercury in fish, including top predatory fish that humans target for consumption, is MeHg (Kamps et al. 1972; Bloom 1992). To develop fish consumption advisories for humans eating fish, total mercury concentration (Hg concentration), which is the sum of the MeHg concentration and the inorganic mercury concentration, is most often measured and reported for fish.
Given the consistent biomagnification of mercury with increasing trophic position within food webs around the world (LaVoie et al. 2013), mercury has been selected as a tracer of food consumption by free-ranging fish in lakes, rivers, and oceans (Trudel et al. 2000; Trudel and Rasmussen 2001). By determining Hg concentration both in the fish and in their prey, the rate at which free-ranging fish consume food in their natural environment can be estimated, based on several assumptions concerning the entry and exit of mercury to and from the fish. Here, we refer to this approach of using Hg determinations to estimate food consumption by free-ranging fish as an application of mercury mass balance models (MMBMs). Estimation of food consumption by free-ranging fish has proven invaluable in better understanding fish growth and consumption in the field (Trudel et al. 2001; Chipps and Wahl 2008; Madenjian 2011; Deslauriers et al. 2017). Moreover, estimates of food consumption by fish can be used to quantify the strength of the predator–prey link that, in turn, can be applied toward improved management of valuable fisheries. For example, quantification of the predatory effect of Chinook salmon (Oncorhynchus tshawytscha) on alewives (Alosa pseudoharengus) has been an important asset in guiding the management of the Chinook salmon fishery in Lake Michigan for more than 30 years (Hansen et al. 1993; Tsehaye et al. 2014). For this specific application to Lake Michigan fisheries, a Wisconsin bioenergetics model (BEM) was used to estimate annual consumption of alewives by the Chinook salmon population, although a MMBM could have also been used in this application.
Most often, MMBMs are used to estimate food consumption by free-ranging fish (Trudel et al. 2000; Trudel and Rasmussen 2001), but MMBMs may also be used to estimate or predict Hg concentration in free-ranging fish given a specified feeding rate schedule for the fish (Rennie et al. 2005; Trudel and Rasmussen 2006; Schartup et al. 2019). In applying MMBMs to data for free-ranging fish, the issue of Hg concentration versus MeHg concentration in the fish usually needs to be addressed. In some cases, only Hg concentration data are available, and an assumption regarding the percentage of the Hg in the fish that is MeHg is typically made. Frequently, all the Hg in the fish is assumed to be MeHg, and this assumption is justifiable based on the abovementioned studies and others (Van Walleghem et al. 2007; Raymond and Rossmann 2009; Madenjian et al. 2015; Schartup et al. 2019). All of the Hg entering the fish’s body is usually assumed to enter via food consumption, and this assumption is justifiable given the results of earlier studies (e.g., Hall et al. 1997), as well as newer study results (Hrenchuk et al. 2012; Lescord et al. 2018). Typically, the issue of whole-fish mercury concentration versus fillet or muscle mercury concentration also needs to be addressed in applying MMBMs, but conversion factors from the literature are available to deal with this issue (Becker and Bigham 1995; Peterson et al. 2005).
Although the predominant pathway of entry of Hg into the fish’s body is indeed through dietary intake and all of the Hg entering the fish’s body is typically assumed to be through food consumption, Hg may also enter the fish’s body through direct uptake from water (Trudel et al. 2000; Trudel and Rasmussen 2001; Hrenchuk et al. 2012; Lescord et al. 2018). Fish gills play a role in both direct uptake of Hg from water and in elimination of Hg (Wang and Wang 2010, 2012; Bjerregaard et al. 2011; Peng et al. 2016). Fish can also eliminate Hg from their bodies via release of bile from the liver into the digestive tract and via excretion of urine from the kidneys. Some of the factors influencing direct uptake of mercury via the gills from the water include Hg concentration and dissolved organic carbon concentration in the surrounding water, as well as salinity of the water (Wang and Wang 2010). Hg accumulation rate for the fish tends to increase with increasing concentration of Hg in the fish’s food (Trudel et al. 2000; Trudel and Rasmussen 2001; Wang and Wang 2012). The rate at which the fish eliminates Hg from its body appears to be influenced by water temperature and the fish’s weight (Trudel and Rasmussen 1997). Moreover, dietary selenium has been shown to increase Hg-elimination rate in fish (Bjerregaard et al. 2011).
Mercury accumulation in fish is equal to the balance of intakes minus any losses. As such, a critical assumption of the MMBM approach is that the rate at which fish eliminate Hg from their bodies is accurately estimated (Trudel et al. 2000; Trudel and Rasmussen 2001). For MMBM applications, Trudel and Rasmussen (1997) developed a regression model for estimating Hg-elimination rate as a function of water temperature and fish weight. This regression model was based on the best estimates of Hg-elimination rate available from the scientific literature at the time of model development. It included five published studies of Hg-elimination rate for six different fish species (including marine, brackish, and freshwater species). All but one study occurred in the laboratory. Two regression equations to estimate Hg-elimination rate were developed—one from data on northern pike (Esox lucius) and European plaice (Pleuronectes platessa) that were chronically exposed to Hg and the other from data on five species of fish that were acutely exposed to Hg. Trudel and Rasmussen (1997) concluded that Hg-elimination rate from short-term (<90 d) experiments was overestimated, because the series of observations on Hg body burden in the fish was not sufficiently long to accurately distinguish between the fast and slow components of elimination following the dosing of fish with Hg. Immediately after dosing, Hg body burden in the fish declines at a relatively fast rate, but then this period of relatively fast elimination is followed by a period of relatively slow elimination (Trudel and Rasmussen 1997). Regardless of whether the fish were chronically exposed to Hg or acutely exposed to Hg, the slow component reflects the Hg-elimination rate appropriate for use in MMBMs. In practice, the original chronic exposure equation continues to be used for MMBM applications to free-ranging fish in the field (Ferriss and Essington 2014; Schartup et al. 2019).
The goals of this perspective paper are to alert the reader to the problem of inaccurate estimation of Hg-elimination rate associated with the present-day version of MMBMs and to explore the consequences and implications of this problem. Specific objectives of our study are: (i) review recent evidence indicating that the chronic exposure regression equation overestimates Hg-elimination rates for fish, (ii) explore the underlying reasons for this overestimation of Hg-elimination rate, (iii) discuss the consequences of such overestimation on both estimates of food consumption by fish and estimates of Hg concentration in fish generated by MMBMs, (iv) review evidence indicating that factors other than weight and temperature also influence Hg-elimination rate, (v) explore the implications of overestimation of Hg-elimination rate on emerging applications for MMBMs, and (vi) explore the implications of overestimation of Hg-elimination rate on previously documented discrepancies in estimates of food consumption between MMBM and BEM applications. Regarding the sixth objective, MMBMs and BEMs both provide estimates of food consumption by fish, which should agree for a given application. However, MMBM estimates of food consumption have substantially differed from BEM estimates of food consumption (Rennie et al. 2005; Trudel and Rasmussen 2006; Ferriss and Essington 2014). Lastly, future research directions for refining MMBMs are discussed.
2. New approaches to the estimation of Hg-elimination rate
Over the past two decades, advances in analytical techniques have allowed for new insights into the rate at which fish eliminate Hg from their bodies. Two field studies on the rate of Hg elimination in free-ranging fishes took place as part of a whole-ecosystem Hg loading experiment where isotopically enriched Hg was added to the surface waters of a remote boreal lake (Harris et al. 2007). Yellow perch (Perca flavescens) and northern pike naturally accumulated isotopic Hg over several years before being moved from the experimental lake to a nearby untreated lake. Fish were then periodically captured and changes in the body burden of isotopic Hg were monitored over time (Table 1; Van Walleghem et al. 2007, 2013). The estimates of Hg-elimination rate for yellow perch and northern pike were 3.1 and 2.7 times lower, respectively, than estimates generated from the chronic exposure equation.
Table 1.
Species | Estimate of Hg-elimination rate (d−1) | Reference |
---|---|---|
Northern pike | 0.000907 | Lockhart et al. (1972) |
0.000581 | Van Walleghem et al. (2013) | |
European plaice | 0.002570 | Pentreath (1976) |
Yellow perch | 0.001417 | Van Walleghem et al. (2007) |
Lake whitefish | 0.000730 | Madenjian and O’Connor (2008) |
Lake trout | 0.000244 | Madenjian et al. (2012) |
Further, in a series of carefully controlled natural predator–prey feeding studies conducted in the laboratory (>130 d in duration), Hg-elimination rates for lake whitefish (Coregonus clupeaformis) and lake trout (Salvelinus namaycush) were estimated to be 2.4 times lower and 5.5 times lower, respectively, than those estimated from the chronic exposure equation (Table 1; Madenjian and O’Connor 2008; Madenjian et al. 2012). Hg concentration in the predators at the start and end of the experiments, as well as Hg concentration in the prey, were determined. Food consumption and growth of the predators were monitored throughout the experiment. Gross trophic transfer efficiency of Hg from the prey to the predator was assumed to be 0.80, which was a reasonable assumption based on studies cited by Trudel et al. (2000) and Trudel and Rasmussen (2001).
Common to both the whole-ecosystem experiments (Van Walleghem et al. 2007, 2013) and the laboratory feeding experiments (Madenjian and O’Connor 2008; Madenjian et al. 2012) was a natural route of Hg exposure to fish via their diet. In contrast, in most of the studies upon which the original Hg-elimination rate model was based (Trudel and Rasmussen 1997), fish were either exposed to a concentrated solution of MeHg or received an injection of MeHg into muscle or the stomach. These routes of Hg exposure do not mimic the natural route to fish through their diet, and they may have introduced artifacts that obfuscated accurate characterization of the rate at which Hg is eliminated by fish in a natural setting. Similarly, the toxicokinetics of Hg in fish fed food artificially spiked with Hg differed from those of fish fed naturally contaminated food (Bradley et al. 2017). Further, elimination rates of Hg and MeHg vary considerably across the various tissues of fish (Wang and Wang 2010, 2012; Peng et al. 2016). In addition, overestimation of Hg-elimination rate may have also been partially due to an insufficient amount of time to reliably separate the fast and slow components of elimination in some of the studies used to develop the original Hg-elimination rate model. Researchers once considered 90 d as enough time to demarcate these two components, but even more time may be required. Moreover, Hg-elimination rate was likely overestimated in one of the studies upon which the chronic exposure equation was based, due to the short duration of the elimination phase of the experiment. To estimate Hg-elimination rate, Pentreath (1976) tracked the body burden of radiolabeled MeHg in juvenile European plaice over a 70-d period immediately following the exposure of these fish to a solution of radiolabeled MeHg (Table 1). Not only was the estimate of Hg-elimination rate based on an insufficiently long tracking period, but no effort was also made to distinguish between the fast and slow components of elimination. Thus, these shortcomings of the Pentreath (1976) study contributed to the overestimation of Hg-elimination rate by the chronic exposure equation. We also point out that although the degree of overestimation of Hg-elimination rate was considerably less for the acute exposure equation than that for the chronic exposure equation, both equations overestimated Hg-elimination rate (Van Walleghem et al. 2007, 2013; Madenjian and O’Connor 2008; Madenjian et al. 2012). The acute exposure equation overestimated Hg-elimination rate by 15%–163%.
3. Influence of Hg-elimination rate on estimates of food consumption and Hg concentration
Food consumption estimates derived from MMBM applications are sensitive to Hg-elimination rate (Madenjian and O’Connor 2008). To demonstrate the effects of Hg-elimination rate on food consumption, we estimated daily ingestion rate by walleye (Sander vitreus) in Bitter Lake, South Dakota (USA) using a MMBM (Trudel et al. 2000). Data for walleye (ages 2–8 years) are summarized in Selch et al. (2019) and included fish weight (g), Hg concentration (mg/kg or parts per million (ppm) wet weight), seasonal diet composition, prey Hg concentration (ppm wet weight), and water temperature (°C). Walleye in Bitter Lake fed on amphipods, fathead minnows (Pimephales promelas), and chironomids. We calculated Hg-elimination rate (E, d−1) as a function of fish weight (W, g) and water temperature (T, °C) using the original chronic exposure equation where
(1)
Using the MMBM presented in Trudel et al. (2000), we estimated food consumption (g) for an average walleye growing from age 2 (489 g wet weight) to age 8 (1050 g wet weight), using three estimates of Hg-elimination rate: one that corresponded to E, and two lower values 0.5E and 0.1E that corresponded to rates that were 2 and 10 times slower than E, respectively. Note that E is typically used as the Hg-elimination rate in MMBM applications. Here we chose to explore Hg-elimination rates with values ≤E in this MMBM example, as data from four separate studies (Van Walleghem et al. 2007, 2013; Madenjian and O’Connor 2008; Madenjian et al. 2012) showed an overestimation of Hg-elimination rate for each of four fish species by the original chronic exposure equation. Food consumption estimated using E as the Hg-elimination rate value was appreciably higher than that obtained using slower Hg-elimination rates (Fig. 1). Cumulative food consumption at age 8 based on E was 1.5 and 2.6 times higher than that based on 0.5E and 0.1E, respectively. The discrepancy between cumulative food consumption based on E and that based on 0.5E or 0.1E would be expected to further widen as walleye aged beyond age 8.
Fig. 1.

The MMBM can be rearranged to estimate walleye Hg concentration (Ct) aswhere C0 is the initial Hg concentration in walleye (ppm), Ct is the Hg concentration in walleye at time t, and G equals specific growth rate (d−1; Trudel and Rasmussen 2006). Hg concentration of prey (ppm) and assimilation efficiency of Hg by walleye are represented by Cδ and α (=0.80), respectively. To explore how walleye Hg accumulation is influenced by elimination rate, we estimated specific feeding rate (I, d−1) of 2–8-year-old walleye using a bioenergetics model tailored to this fish species (Kitchell et al. 1977; Selch et al. 2019; Deslauriers et al. 2017). A comparison of Ct estimates at Hg-elimination rates of E, 0.5E, and 0.1E revealed that Hg concentration in young walleye with relatively high specific growth rates (ages 3–4) was reasonably well predicted by E, i.e., within 10% of observed values (Fig. 2). However, among larger, older fish (>age 4), observed Hg concentration was greater than that predicted by E. Indeed, by age 8, observed Hg concentration in walleye (1.05 ppm) was twice that predicted by eq. (2) (0.524 ppm). At lower elimination rates, Hg accumulation in walleye substantially increased with increasing age. At the lowest elimination rate (0.1E), predicted Hg concentration was higher than observed values for walleye. Predicted Hg concentrations at 0.5E, however, were in better agreement with observed values, implying that eq. (1) overestimates Hg-elimination rate for older (>4 years old), slower growing walleye (Fig. 2).
(2)
Fig. 2.

4. Factors other than temperature and weight affect Hg-elimination rate
There is growing evidence for the contention that Hg-elimination rate can vary considerably among species and between the sexes, and that much of this variability is not explained by temperature or weight (Madenjian et al. 2016). For example, in the laboratory tank studies described earlier, estimated Hg-elimination rate for lake whitefish was nearly three times higher than that for lake trout, even though the lake whitefish were of similar size to the lake trout and both of these cold-water species experienced similar water temperatures (Madenjian and O’Connor 2008; Madenjian et al. 2012, 2016). Moreover, the degree of overestimation of Hg-elimination rate using the chronic exposure equation ranged from a factor of 2.4 to a factor of 5.5 (Van Walleghem et al. 2007, 2013; Madenjian and O’Connor 2008; Madenjian et al. 2012), further supporting the contention that factors other than temperature and fish weight also affected Hg-elimination rate.
Only in the last six years has sex been recognized as an important factor regulating Hg-elimination rates in teleost fishes (Madenjian et al. 2014, 2016). For example, adult male northern pike eliminated Hg from their bodies at a rate more than twice as fast as that of adult female northern pike from the same population (Madenjian et al. 2014). Due to lack of available data, sex was not considered as an independent variable in developing the original regression models for Hg-elimination rate in fish. Given the compelling research on laboratory mice (Mus musculus), Madenjian et al. (2014, 2016) hypothesized that certain androgens, such as testosterone and 11-ketotesosterone, in male teleost fishes are capable of enhancing the rate at which males eliminate Hg from their bodies. In laboratory mice, adult males eliminated Hg from their bodies at a rate that was roughly three times higher than that of adult females (Hirayama and Yasutake 1986). Follow-up laboratory experimentation showed that testosterone was the agent responsible for enhanced Hg-elimination rate in adult male mice compared with adult female mice (Yasutake et al. 1989; Tanaka et al. 1991, 1992). In addition, Kostyniak (1980) reported that the rate that adult male mice excreted Hg in their urine differed 5-fold between strains of mice. Weight and body temperature of males did not appreciably vary between strains; therefore, this difference in Hg-elimination rates was not attributable to weight or body temperature differences between strains. Rather, variation in testosterone levels between strains could explain the observed difference in Hg-elimination rates (Kemp and Drinkwater 1989; Tanaka et al. 1991). Apparently, Hg-elimination rate for males varies considerably from one species or strain of vertebrate to another, perhaps reflecting high interspecific variability in levels of testosterone (and 11-ketotestosterone in teleost fishes) of males. Peak testosterone levels of males varied more than 40-fold across 107 species of birds (Garamszegi et al. 2005). A similar degree of interspecific variability in the testosterone and 11-ketotestosterone levels of male teleost fishes is a reasonable expectation.
Further evidence for species and sex differences in Hg-elimination rate comes from comparative studies that examined both polychlorinated biphenyl (PCB) and Hg concentrations in fish. Like Hg, nearly all PCB accumulation in fish is from dietary intake (Weininger 1978; Borgmann and Whittle 1991, 1992). Unlike Hg, long-term elimination of PCBs by fish has been characterized as negligible (Madenjian et al. 1998). Madenjian et al. (2016) found that males consistently exceeded females in PCB concentration in eight species of fish (♂:♀ = 1.17–1.43), whereas the Hg ratio (♂:♀ = 0.82–1.16) was always less than the PCB ratio, and males exceeded females in Hg concentration in some species of fish, whereas females exceeded males in Hg concentration in other fish species. The most plausible explanation for these findings was that the difference in Hg-elimination rates between the sexes varied substantially among species, thereby causing the difference between the PCB ratio and the Hg ratio to vary substantially from one species to another (Madenjian et al. 2016). In teleost fishes, males eliminated Hg from their bodies faster than females, but the relative difference in Hg-elimination rates between the sexes varied across species.
5. Emerging applications of MMBMs
An application of growing importance is to use MMBMs to generate predictions of anthropogenic effects on Hg accumulation in fish, particularly fish frequently consumed by humans. Recognition of the deleterious effects of Hg exposure to humans has prompted development of the Minimata Convention on Mercury, an international treaty aimed at reducing Hg emissions at a global scale (UNEP 2013). Accurate modeling of Hg accumulation in fish is critical to understanding how fish will respond to anthropogenic effects like lower Hg emissions and climate change. Consequently, the reassessment of Hg-elimination rates is timely given these recent research initiatives into the effects of reduced Hg emissions, climate change, changes in food web structure, and overfishing on Hg accumulation in fish (Lepak et al. 2019; Schartup et al. 2019). For example, Schartup et al. (2019) used a MMBM, based on the original chronic exposure Hg-elimination rate model, to investigate climate change and overfishing effects on Hg accumulation in Atlantic bluefin tuna (Thunnus thynnus). Because Hg-elimination rate was likely overestimated in this application, Hg concentration in Atlantic bluefin tuna tissue was probably underestimated. The degree of underestimation could be 2-fold or greater (Fig. 2).
6. Discrepancy between MMBM and BEM estimates of food consumption
When food consumption estimates have been compared between the MMBM and BEM approaches, the MMBM estimate exceeds the BEM estimate in nearly all cases (Rennie et al. 2005; Trudel and Rasmussen 2006; Ferriss and Essington 2014). For example, the MMBM estimate of food consumption rate exceeded the BEM estimate of food consumption rate by as little as roughly 40% to well over 100% in applications to four species of tunas (Ferriss and Essington 2014). This interspecific variation in the discrepancy between the MMBM and BEM estimates is roughly in accord with the variability in the degree of overestimation of Hg-elimination rate across species, namely yellow perch, northern pike, lake whitefish, and lake trout, elucidated above. Overestimation of Hg-elimination rate by factors of 2 and 5.5 can lead to overestimation of food consumption by 50% and 100%, respectively (Fig. 1). Thus, an important contributing factor to published discrepancies in food consumption estimates between application of a MMBM and application of a BEM appears to be overestimation of Hg-elimination rate resulting in overestimation of food consumption by the MMBM.
The discrepancy between the MMBM and BEM estimates of food consumption had been attributed to underestimation of swimming activity by BEMs (Trudel and Rasmussen 2006), but this hypothesis is no longer supported by recent findings. Based on the new estimates of Hg-elimination rates described in Section 2, and the results of the simulation modeling presented in Fig. 1, the bulk of this discrepancy in the estimates of food consumption appears to be explained by simply the overestimation of Hg-elimination rate. Consider the case that Hg-elimination rate is overestimated by a factor of 5.5, as was found for lake trout (Madenjian et al. 2012). This case would correspond with approximately the halfway point between the 0.5E and 0.1E scenarios displayed in Fig. 1. Thus, by age 8, cumulative food consumption would be overestimated by roughly 2-fold when using the original chronic exposure equation (eq. (1)). Coincidentally, the MMBM estimate of cumulative food consumption by age 8 lake trout in Lake Ontario was about two times higher than that from the BEM application (Trudel and Rasmussen 2006). Thus, the discrepancy in the food consumption estimates between the MMBM and BEM applications appears to be explained by the overestimation of Hg-elimination rate in this example. Our contention is further strengthened by the results of the study by Madenjian et al. (2000), who concluded that the lake trout BEM developed by Stewart et al. (1983), the same BEM used in the Trudel and Rasmussen (2006) study, yielded unbiased estimates of food consumption by adult lake trout in Lake Michigan. Madenjian et al. (2000) used PCBs as a tracer of food consumption to arrive at their conclusion. Given the known range in the degree of overestimation of Hg-elimination rate (2.4–5.5 times higher) from new studies, in conjunction with the results from our simulation modeling, much of the discrepancy between the MMBM and BEM estimates of food consumption for the four species of tunas studied by Ferriss and Essington (2014) would likely be accounted for by overestimation of Hg-elimination rate in the MMBM applications.
7. Future research directions
We see a need to continue to amass a data set of new estimates of Hg-elimination rate, based on the field and laboratory approaches that involve the natural uptake of MeHg, across a variety of fish species, water temperatures, and fish sizes (weights). The aforementioned studies included only a small number of common, north-temperate cold- and cool-water freshwater fish. Clearly there is a need to expand these types of studies to other fish species, especially commercially important species that occupy marine and brackish environments. Opportunities to conduct field studies like those by Van Walleghem et al. (2007, 2013) should be pursued, using a range of species that occupy diverse trophic guilds and temperature regimes as the focus of these new studies. Likewise, more laboratory experiments like those by Madenjian et al. (2012) are needed to build a data set of Hg-elimination rate estimates based on dietary exposure with natural foods in laboratory tanks. The incorporation of mercury stable isotopes into these natural foods may lead to further refinement of Hg-elimination rate estimates in future laboratory studies (Hrenchuk et al. 2012). To elucidate the relationship between fish weight and Hg-elimination rate, new experiments should be conducted on various sizes of fish of the same species in the laboratory. Similarly, to investigate the relationship between water temperature and Hg-elimination rate, Hg-elimination rates would need to be determined for groups of fish of the same species, but each group would be subjected to a different water temperature during the long-term feeding experiment.
Corroboration of Hg-elimination rate estimates between field studies and those from controlled laboratory experiments is needed. Such corroboration would promote greater confidence in the MMBM predictions of food consumption by fish and Hg concentration in fish. Moreover, we see great opportunity to combine many rapidly evolving technologies to better link field and laboratory approaches. For example, various telemetry technologies allow for the near-continuous tracking of individual fish movements, rates of activity, habitat use, and temperature occupancy (Hussey et al. 2015), which can be combined with nonlethal sampling methods to quantify diet composition and Hg concentrations (Baker et al. 2004). Estimates of activity from field-based telemetry data can, in turn, provide an improved understanding of feeding (i.e., metabolic) requirements of free-ranging fish (Semmens et al. 2013; Brodie et al. 2016), which are known to vary across ecosystems, even for the same species (Cruz-Font et al. 2019). Importantly, long-term field studies can capture the seasonal influence of temperature and activity, as well as individual variability, all of which can influence Hg-elimination rate of free-ranging fish (Van Walleghem et al. 2013).
Studies that explore how factors other than fish size and water temperature (e.g., sex, species, growth history, etc.) influence Hg-elimination rate in fish would make a significant contribution toward building this data set of new estimates of Hg-elimination rate. Clearly, sex is an important factor regulating Hg-elimination rates in fish. Further, some of the variation in Hg-elimination rate unexplained by fish weight and water temperature may be attributable to concentrations of testosterone and 11-ketotestosterone varying across species. Determining the levels of these two androgens in male fish across a wide array of fish species would represent an important step in investigating the relationship between androgen levels and Hg-elimination rate in male fish. Then, regression analysis could be used to establish the nature of the association between androgen levels and Hg-elimination rate. In addition, we expect that future studies will likely reveal other factors that play a role in influencing Hg-elimination rate of fish.
Once a data set of new estimates of Hg-elimination rate in fish has been amassed, then various types of regression analyses should be applied to the data set to develop a new regression model for Hg-elimination rate. The objective here would be to arrive at a regression model that predicts Hg-elimination rate with reasonable accuracy over wide ranges of the independent variables included in the model. This new regression model could then be incorporated into MMBMs (Trudel et al. 2000; Trudel and Rasmussen 2001), thereby leading to improved model performance such that unbiased estimates of food consumption are generated. In turn, the improved MMBMs can be used to check on the accuracy of the activity component of BEMs (Rennie et al. 2005; Trudel and Rasmussen 2006). We acknowledge that the processes regulating Hg-elimination rates in fish may be so complex that several regression models may need to be developed. Perhaps models may be required by latitudes (temperate vs. tropical) and system types (marine vs. freshwater). To illustrate the potential complexity of environmental influences on Hg-elimination rates, consider the case of freshwater systems contaminated with selenium. Dietary selenium has been shown to reduce retention of MeHg in freshwater fish (Bjerregaard et al. 2011). Specifically, dietary selenium augmented Hg-elimination rate of goldfish (Carassius auratus) in a dose-dependent manner.
We also see a need to recalculate food consumption estimates from previous MMBM applications using the abovementioned new regression model for Hg-elimination rate that yields accurate estimates of Hg-elimination rate. Further, differences between food consumption estimates derived from MMBM applications with those from BEM applications should be reexamined to determine the degree of discrepancy between the two sets of estimates that can be accounted for by inaccurate estimation of Hg-elimination rate. Given the results presented in this perspective, the new estimates of food consumption from the improved MMBMs would be in considerably closer agreement with the estimates of food consumption from BEMs than the estimates generated from the original formulation of the MMBMs with the biased regression equation used to estimate Hg-elimination rate.
8. Conclusion
Exciting opportunities exist to improve the accuracy of MMBMs via re-examination of Hg-elimination rates in fish. Revision of the Hg-elimination rate model would require generating a new set of Hg-elimination rate estimates based on techniques involving long-term natural dietary exposure of Hg to the fish. Once a new set of Hg-elimination rate estimates becomes available, a new model to predict Hg-elimination rate can be developed and then incorporated into MMBMs. With this new model to predict Hg-elimination rate, accuracy of the MMBMs will be enhanced. Specifically, accuracy of MMBM predictions of Hg concentration in fish under various scenarios of changes in Hg emissions, climate change, and overfishing will improve. We anticipate that much of the discrepancy in food consumption estimates between MMBM applications and BEM applications that has been previously described in the literature will vanish when accurate estimates of Hg-elimination rate are used in the MMBM applications. Corroboration between field estimates of Hg-elimination rate and laboratory estimates of Hg-elimination rate would improve the reliability of MMBM estimates of food consumption by fish and Hg concentration in fish. Finally, researchers should take advantage of rapidly evolving technologies to better link field and laboratory approaches toward quantifying energy budgets of fish.
Acknowledgements
Michael Brown (South Dakota State University) reviewed an earlier version of the manuscript and provided helpful suggestions for its improvement.
References
Baker RF, Blanchfield PJ, Paterson MJ, Flett RJ, and Wesson L. 2004. Evaluation of nonlethal methods for the analysis of mercury in fish tissue. Transactions of the American Fisheries Society, 133: 568–576.
Becker DS, and Bigham GN. 1995. Distribution of mercury in the aquatic food web of Onondaga Lake, New York. Water, Air, and Soil Pollution, 80: 563–571.
Bjerregaard P, Fjordside S, Hansen MG, and Petrova MB. 2011. Dietary selenium reduces retention of methyl mercury in freshwater fish. Environmental Science & Technology, 45: 9793–9798.
Bloom NS. 1992. On the chemical form of mercury in edible fish and marine invertebrate tissue. Canadian Journal of Fisheries and Aquatic Sciences, 49: 1010–1017.
Boening DW. 2000. Ecological effects, transport, and fate of mercury: a general review. Chemosphere, 40: 1335–1351.
Borgmann U, and Whittle DM. 1991. Contaminant concentration trends in Lake Ontario lake trout (Salvelinus namaycush): 1977 to 1988. Journal of Great Lakes Research, 17: 368–381.
Borgmann U, and Whittle DM. 1992. Bioenergetics and PCB, DDE, and mercury dynamics in Lake Ontario lake trout (Salvelinus namaycush): a model based on surveillance data. Canadian Journal of Fisheries and Aquatic Sciences, 49: 1086–1096.
Bradley MA, Barst BD, and Basu N. 2017. A review of mercury bioavailability in humans and fish. International Journal of Environmental Research and Public Health, 14: 169.
Brodie S, Taylor MD, Smith JA, Suthers IM, Gray CA, and Payne NL. 2016. Improving consumption rate estimates by incorporating wild activity into a bioenergetics model. Ecology and Evolution, 6: 2262–2274.
Chipps SR, and Wahl DH. 2008. Bioenergetics modeling in the 21st century: reviewing new insights and revisiting old constraints. Transactions of the American Fisheries Society, 137: 298–313.
Clarkson TW, Magos L, and Myers GJ. 2003. The toxicology of mercury—current exposures and clinical manifestations. New England Journal of Medicine, 349: 1731–1737.
Cruz-Font L, Shuter BJ, Blanchfield PJ, Minns CK, and Rennie MD. 2019. Life at the top: lake ecotype influences the foraging pattern, metabolic costs and life history of an apex fish predator. Journal of Animal Ecology, 88: 702–716.
Deslauriers D, Chipps SR, Breck JE, Rice JA, and Madenjian CP. 2017. Fish Bioenergetics 4.0: an R-based modeling application. Fisheries, 42: 586–596.
Driscoll CT, Mason RP, Chan HM, Jacob DJ, and Pirrone N. 2013. Mercury as a global pollutant: sources, pathways, and effects. Environmental Science & Technology, 47: 4967–4983.
Ferriss BE, and Essington TE. 2014. Can fish consumption rate estimates be improved by linking bioenergetics and mercury mass balance models? Application to tunas. Ecological Modelling, 272: 232–241.
Garamszegi LZ, Eens M, Hurtrez-Boussès S, and Møller AP. 2005. Testosterone, testes size, and mating success in birds: a comparative study. Hormones and Behavior, 47: 389–409.
Hall BD, Bodaly RA, Fudge RJP, Rudd JWM, and Rosenberg DM. 1997. Food as the dominant pathway of methylmercury uptake by fish. Water, Air, and Soil Pollution, 100: 13–24.
Hansen MJ, Boisclair D, Brandt SB, Hewett SW, Kitchell JF, Lucas MC, et al. 1993. Applications of bioenergetics models to fish ecology and management: where do we go from here? Transactions of the American Fisheries Society, 122: 1019–1030.
Harris RC, Rudd JWM, Amyot M, Babiarz CL, Beaty KG, Blanchfield PJ, et al. 2007. Whole-ecosystem study shows rapid fish-mercury response to changes in mercury deposition. Proceedings of the National Academy of Sciences of the United States of America, 104: 16586–16591.
Hirayama K, and Yasutake A. 1986. Sex and age differences in mercury distribution and excretion in methylmercury-administered mice. Journal of Toxicology and Environmental Health, 18: 49–60.
Hrenchuk LE, Blanchfield PJ, Paterson MJ, and Hintelmann HH. 2012. Dietary and waterborne mercury accumulation by yellow perch: a field experiment. Environmental Science & Technology, 46: 509–516.
Hussey NE, Kessel ST, Aarestrup K, Cooke SJ, Cowley PD, Fisk AT, et al. 2015. Aquatic animal telemetry: a panoramic window into the underwater world. Science, 348: 1255642.
Kamps LR, Carr R, and Miller H. 1972. Total mercury-monomethylmercury content of several species of fish. Bulletin of Environmental Contamination and Toxicology, 8: 273–279.
Kemp CJ, and Drinkwater NR. 1989. Genetic variation in liver tumor susceptibility, plasma testosterone levels, and androgen receptor binding in six inbred strains of mice. Cancer Research, 49: 5044–5047.
Kitchell, JF, Stewart DJ, and Weininger D. 1977. Applications of a bioenergetics model to yellow perch (Perca flavescens) and walleye (Stizostedion vitreum vitreum). Journal of the Fisheries Research Board of Canada, 34:1922–1935.
Kostyniak PJ. 1980. Differences in elimination rates of methylmercury between two genetic variant strains of mice. Toxicology Letters (Amsterdam), 6: 405–410.
LaVoie RA, Jardine TD, Chumchal MM, Kidd KA, and Campbell LM. 2013. Biomagnification of mercury in aquatic food webs: a worldwide meta-analysis. Environmental Science & Technology, 47: 13385–13394.
Lepak RF, Hoffman JC, Janssen SE, Krabbenhoft DP, Ogorek JM, DeWild JF, et al. 2019. Mercury source changes and food web shifts alter contamination signatures of predatory fish from Lake Michigan. Proceedings of the National Academy of Sciences of the United States of America, 116: 23600–23608.
Lescord GL, Johnston TA, Branfireun BA, and Gunn JM. 2018. Percentage of methylmercury in the muscle tissue of freshwater fish varies with body size and age and among species. Environmental Toxicology and Chemistry, 37: 2682–2691.
Lockhart WL, Uthe JF, Kenney AR, and Mehrle PM. 1972. Methylmercury in northern pike (Esox lucius): distribution, elimination, and some biochemical characteristics of contaminated fish. Journal of the Fisheries Research Board of Canada, 29: 1519–1523.
Madenjian CP. 2011. Bioenergetics in ecosystems. In Encyclopedia of fish physiology: from genome to environment. Vol. 3. Edited by AP Farrell. Academic Press, San Diego, California. pp. 1675–1680.
Madenjian CP, and O’Connor DV. 2008. Trophic transfer efficiency of mercury to lake whitefish Coregonus clupeaformis from its prey. Bulletin of Environmental Contamination and Toxicology, 81: 566–570.
Madenjian CP, Hesselberg RJ, DeSorcie TJ, Schmidt LJ, Stedman RM, Quintal RT, et al. 1998. Estimate of net trophic transfer efficiency of PCBs to Lake Michigan lake trout from their prey. Environmental Science & Technology, 32: 886–891.
Madenjian CP, O’Connor DV, and Nortrup DA. 2000. A new approach toward evaluation of fish bioenergetics models. Canadian Journal of Fisheries and Aquatic Sciences, 57: 1025–1032.
Madenjian CP, David SR, and Krabbenhoft DP. 2012. Trophic transfer efficiency of methylmercury and inorganic mercury to lake trout Salvelinus namaycush from its prey. Archives of Environmental Contamination and Toxicology, 63: 262–269.
Madenjian CP, Blanchfield PJ, Hrenchuk LE, and Van Walleghem JLA. 2014. Mercury elimination rates for adult northern pike Esox lucius: evidence for a sex effect. Bulletin of Environmental Contamination and Toxicology, 93: 144–148.
Madenjian CP, Stapanian MA, Cott PA, Krabbenhoft DP, Edwards WH, Ogilvie LM, et al. 2015. Females exceed males in mercury concentrations of burbot Lota lota. Archives of Environmental Contamination and Toxicology, 68: 678–688.
Madenjian CP, Rediske RR, Krabbenhoft DP, Stapanian MA, Chernyak SM, and O’Keefe JP. 2016. Sex differences in contaminant concentrations of fish: a synthesis. Biology of Sex Differences, 7: 42.
Peng X, Liu F, and Wang W-X. 2016. Organ-specific accumulation, transportation, and elimination of methylmercury and inorganic mercury in a low Hg accumulating fish. Environmental Toxicology and Chemistry, 35: 2074–2083.
Pentreath RJ. 1976. The accumulation of organic mercury from sea water by the plaice, Pleuronectes platessa L. Journal of Experimental Marine Biology and Ecology, 24: 121–132.
Peterson SA, Van Sickle J, Hughes RM, Schacher JA, and Echols SF. 2005. A biopsy procedure for determining filet and predicting whole-fish mercury concentration. Archives of Environmental Contamination and Toxicology, 48: 99–107.
Raymond B, and Rossmann R. 2009. Total and methyl mercury accumulation in 1994-1995 Lake Michigan lake trout and forage fish. Journal of Great Lakes Research, 35: 438–446.
Rennie MD, Collins NC, Shuter BJ, Rajotte JW, and Couture P. 2005. A comparison of methods for estimating activity costs of wild fish populations: more active fish observed to grow slower. Canadian Journal of Fisheries and Aquatic Sciences, 62: 767–780.
Rice KM, Walker EM Jr, Wu M, Gillette C, and Blough ER. 2014. Environmental mercury and its toxic effects. Journal of Preventive Medicine and Public Health, 47: 74–83.
Sandheinrich MB, Bhavsar SP, Bodaly RA, Drevnick PE, and Paul EA. 2011. Ecological risk of methylmercury to piscivorous fish of the Great Lakes region. Ecotoxicology, 20: 1577–1587.
Schartup AT, Thackray CP, Qureshi A, Dassuncao C, Gillespie K, Hanke A, et al. 2019. Climate change and overfishing increase neurotoxicant in marine predators. Nature, 572: 648–650.
Selch TM, Chipps SR, Blackwell BG, and Hanten RP. 2019. Influence of season, sex, age and diet composition on mercury concentration in walleye Sander vitreus. Archives of Environmental Contamination and Toxicology, 77: 336–343.
Semmens JM, Payne NL, Huveneers C, Sims DW, and Bruce BD. 2013. Feeding requirements of white sharks may be higher than originally thought. Scientific Reports, 3: 1471.
Stewart DJ, Weininger D, Rottiers DV, and Edsall TA. 1983. An energetics model for lake trout, Salvelinus namaycush: application to the Lake Michigan population. Canadian Journal of Fisheries and Aquatic Sciences, 40: 681–698.
Tanaka T, Naganuma A, Kobayashi K, and Imura N. 1991. An explanation for strain and sex differences in renal uptake of methylmercury in mice. Toxicology, 69: 317–329.
Tanaka T, Naganuma A, Miura N, and Imura N. 1992. Role of testosterone in γ-glutamyltranspeptidase-dependent renal methylmercury uptake in mice. Toxicology and Applied Pharmacology, 112: 58–63.
Trudel M, and Rasmussen JB. 1997. Modeling the elimination of mercury by fish. Environmental Science & Technology, 31: 1716–1722.
Trudel M, and Rasmussen JB. 2001. Predicting mercury concentrations in fish using mass balance models. Ecological Applications, 11: 517–529.
Trudel M, and Rasmussen JB. 2006. Bioenergetics and mercury dynamics in fish: a modelling perspective. Canadian Journal of Fisheries and Aquatic Sciences, 63: 1890–1902.
Trudel M, Tremblay A, Schetagne R, and Rasmussen JB. 2000. Estimating food consumption rates of fish using a mercury mass balance model. Canadian Journal of Fisheries and Aquatic Sciences, 57: 414–428.
Trudel M, Tremblay A, Schetagne R, and Rasmussen JB. 2001. Why are dwarf fish so small? An energetic analysis of polymorphism in lake whitefish (Coregonus clupeaformis). Canadian Journal of Fisheries and Aquatic Sciences, 58: 394–405.
Tsehaye I, Jones ML, Bence JR, Brenden TO, Madenjian CP, and Warner DM. 2014. A multispecies statistical age-structured model to assess predator-prey balance: application to an intensively managed Lake Michigan pelagic fish community. Canadian Journal of Fisheries and Aquatic Sciences, 71: 627–644.
United Nations Environment Programme (UNEP). 2013. Minamata Convention on Mercury. UNEP, Nairobi, Kenya [online]: Available from mercuryconvention.org/Portals/11/documents/Booklets/COP3-version/Minamata-Convention-booklet-Sep2019-EN.pdf.
Van Walleghem JLA, Blanchfield PJ, and Hintelmann H. 2007. Elimination of mercury by yellow perch in the wild. Environmental Science & Technology, 41: 5895–5901.
Van Walleghem JLA, Blanchfield PJ, Hrenchuk LE, and Hintelmann H. 2013. Mercury elimination by a top predator, Esox lucius. Environmental Science & Technology, 47: 4147–4154.
Wang R, and Wang W-X. 2010. Importance of speciation in understanding mercury bioaccumulation in tilapia controlled by salinity and dissolved organic matter. Environmental Science & Technology, 44: 7964–7969.
Wang R, and Wang W-X. 2012. Contrasting mercury accumulation patterns in tilapia (Oreochromis niloticus) and implications on somatic growth dilution. Aquatic Toxicology, 114–115: 23–30.
Weininger D. 1978. Accumulation of PCBs by lake trout in Lake Michigan. Ph.D. thesis, University of Wisconsin, Madison, Wisconsin. 232 p.
Wiener JG, Evers DC, Gay DA, Morrison HA, and Williams KA. 2012. Mercury contamination in the Laurentian Great Lakes region: introduction and overview. Environmental Pollution, 161: 243–251.
Yasutake A, Hirayama K, and Inoue M. 1989. Mechanism of urinary excretion of methylmercury in mice. Archives of Toxicology, 63: 479–483.
Information & Authors
Information
Published In
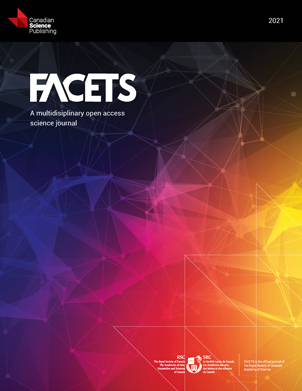
FACETS
Volume 6 • Number 1 • January 2021
Pages: 272 - 286
Editor: Mark Mallory
History
Received: 9 May 2020
Accepted: 23 December 2020
Version of record online: 4 March 2021
Copyright
© 2021 Madenjian et al. This work is licensed under a Creative Commons Attribution 4.0 International License (CC BY 4.0), which permits unrestricted use, distribution, and reproduction in any medium, provided the original author(s) and source are credited.
Data Availability Statement
All relevant data are within the paper.
Key Words
Sections
Subjects
Authors
Author Contributions
All conceived and designed the study.
All performed the experiments/collected the data.
All analyzed and interpreted the data.
All contributed resources.
All drafted or revised the manuscript.
Competing Interests
The authors have declared that no competing interests exist.
Metrics & Citations
Metrics
Other Metrics
Citations
Cite As
Charles P. Madenjian, Steven R. Chipps, and Paul J. Blanchfield. 2021. Time to refine mercury mass balance models for fish. FACETS.
6(): 272-286. https://doi.org/10.1139/facets-2020-0034
Export Citations
If you have the appropriate software installed, you can download article citation data to the citation manager of your choice. Simply select your manager software from the list below and click Download.
Cited by
1. Interplay of climate change on mercury in fish within mangrove ecosystems: A brief systematic literature review
2. Differential accumulation of metals in the lacustrine and fluvial Alpine bullheads (Cottus poecilopus) and recovery of fish from metal contamination after a flash flood
3. Consumption and activity decline in Northern Pike (Esox lucius) during and after silver nanoparticle addition to a lake
4. Tracking Fish Lifetime Exposure to Mercury Using Eye Lenses
5. High rates of mercury biomagnification in fish from Amazonian floodplain-lake food webs
6. Investigating the dynamics of methylmercury bioaccumulation in the Beaufort Sea shelf food web: a modeling perspective
7. On-Site Determination of Methylmercury by Coupling Solid-Phase Extraction and Voltammetry
8. Experimental evidence for recovery of mercury-contaminated fish populations
9. Tissue Distribution of Mercury and Its Relationship with Selenium in Atlantic Bluefin Tuna (Thunnus thynnus L.)
10. Exposure to methylmercury and inorganic mercury in the food does not lead to trophic magnification in the sea star Asterias rubens