A scoping review of the digital agricultural revolution and ecosystem services: implications for Canadian policy and research agendas
Abstract
The application of technologies such as artificial intelligence, robotics, blockchain, cellular agriculture, and big data analytics to food systems has been described as a digital agricultural revolution with the potential to increase food security and reduce agriculture’s environmental footprint. Yet, the scientific evidence informing how these technologies may impact or enhance ecosystem services has not been comprehensively reviewed. In this scoping review, we examine how digital agricultural technologies may enhance agriculture’s support of ecosystem services. Keyword searches in academic databases resulted in 2337 records, of which 74 records met review criteria and were coded. We identify three clusters of digital agricultural technologies including those that make farm management more precise, increase connectivity, and create novel foods. We then examine modelling and empirical evidence gaps in research linking these technologies to ecosystem services. Finally, we overview barriers to implementing digital agricultural technologies for better ecosystem services management in the Canadian context including economic and political systems; lack of policies on data management, governance, and cybersecurity; and limited training and human resources that prevents producers from fully utilizing these technologies.
Introduction
One of the greatest sustainability challenges in the 21st century is to produce enough food for a growing human population while reducing food systems’ impacts on ecosystems (Godfray and Garnett 2010; Foley 2011). The current wave of technological innovation and digitization in agriculture, known as the digital agricultural revolution (DAR), is said to have the potential to simultaneously increase food security and reduce agriculture’s environmental footprint (Balafoutis et al. 2017; Fraser and Campbell 2019; Basso and Antle 2020). The DAR includes recent technological advancements such as artificial intelligence (AI), robotics, three-dimensional (3-D) printing, nanotechnology sensors, unmanned aerial vehicles (UAVs), blockchain, controlled-environment agriculture (CEA), big data analytics, and the Internet of Things (IoT) (Weersink et al. 2018; Klerkx and Rose 2020). These DAR advancements are game-changing tools essential to facilitating innovative work and redefining techniques in established fields such as food science (e.g., cellular agriculture to create novel foods) and genetics wherein big data, robotics, nanotechnology, and automation are ubiquitous.
Enthusiasm about the application of digital agricultural technologies (DATs) is widespread (see De Clercq et al. 2018 and Walch 2019), as they are considered necessary for keeping the global food system within its environmental limits in likely future scenarios (Springmann et al. 2018). Yet, the DAR brings with it echoes of techno-optimism that require a sober pause to better analyse the deeper potential human and environmental impacts of implementing these technologies. An emerging body of scholarship critically examines the social dimensions of digital farming by highlighting inequities resulting from production-biased models of food security (Bronson 2019; Klerkx et al. 2019; Miles 2019; Rotz et al 2019a). While there is a growing focus on the social dimensions of digital farming, the claimed environmental and ecosystem services (ES) benefits of DATs have not been given the same scrutiny (Lajoie-O’Malley et al. 2020).
There is a paucity of empirical evidence on the proposed causal pathways through which many DATs may reduce agriculture’s negative impacts on the environment. Indeed, several DATs have yet to undergo scientifically grounded assessments that would allow evidence-based comparisons of their environmental impacts (van Eenennaam 2019; Lonkila and Kaljonen 2021; Glaros et al. 2021). In addition, where empirical research show DATs with potential environmental benefits (e.g., reduced agrichemical inputs and pollution through variable rate fertilization) (Finger et al. 2019), the proposed benefits may be based on flawed assumptions regarding human behaviour. For example, decision-makers using DATs may be motivated by profit maximization rather than positive environmental outcomes; thus, technology-driven agricultural intensification could exacerbate agricultural expansion rather than spare land for habitat (Jiren et al. 2018).
In this scoping review, we identify ways in which DATs may serve as an intervening variable between agriculture and ES. We then examine the scientific evidence regarding the ability of DATs to reduce agriculture’s negative impacts on ES and increase agriculture’s enhancement of ES. We review international, peer-reviewed literature, but our discussion and recommendations are focused on the Canadian context. Canada is representative of many industrialized countries in that farmers have access to relatively low-cost capital, there is an expanding and sophisticated hardware and software market for agriculture, the extension of rural broadband and other enabling infrastructures is underway, and there is a well-established network of universities and colleges whose mission is to create a talent pool in emerging technologies. Despite these advantages, Canadian agriculture has been slow to adopt these technologies (Mitchell et al. 2018; Rotz et al. 2019b; Bronson 2019; Phillips et al. 2019). Hence, we aim to contribute to a growing body of literature on ES in Canada in conjunction with contributions featured in this “Food, Fiber, Fuel, and Function” collection.
Analytical framework and research questions
We define ES using the Common International Classification of Ecosystem Services (CICES V5.1) (Haines-Young and Potschin 2018) and drawing from literature outlining agriculture–ES interactions (Dale and Polasky 2007; Swinton et al. 2007; Zhang et al. 2007; Power 2010; Baer and Birgé 2018; Campagne et al. 2018; TEEB 2018; MacPherson et al. 2020; Bennett et al. 2021). CICES is a comprehensive ES classification system that maps onto and extends ES classifications such as Reid et al. (2005) and TEEB (2018). We focus on regulation and maintenance ES1 because DATs’ ability to maximize agriculture’s provisioning services and reduce ecosystem disservices to agriculture are well-described elsewhere (see for example Schrijver et al. 2016 and Li et al. 2016). We exclude cultural ES as studies on agriculture’s cultural ES rarely implicate DATs as a variable (see for example Van Berkel and Verburg 2014; Assandri et al. 2018).
This approach provides us with the agricultural–ES analytic framework presented in Fig. 1, which displays several possible types (air quality, biodiversity, biomass, etc.,) of interactions between ecosystems and agricultural systems and groups these into quadrants. These interactions are further explained in Table 1. We conceptualize agriculture as both influenced by ES (Q1) and ecosystem disservices (Q4), while simultaneously influencing ecosystems through services (Q2) and disservices (Q3). As mentioned above, the “ecosystem disservices to agriculture” listed in Q4 do not relate to the ways in which DAT may enhance productivity of or reduce demand on regulation and maintenance services, hence they fall outside of the scope of this review. This leads us to the three key research questions:
1.
What scientific evidence supports claims that DATs can reduce agriculture’s demand for ES as inputs? (Quadrant 1)
2.
What scientific evidence supports claims that DATs allow agriculture to sustainably enhance or diversify regulation and maintenance ES? (Quadrant 2)
3.
What scientific evidence supports claims that DATs reduce downstream, negative impacts on regulation and maintenance ES? (Quadrant 3)
Fig. 1.

Table 1.
Ecosystem service | Description | Q1: ecosystem service to agriculture | Q2: agriculture services to ecosystems | Q3: agriculture disservices to dcosystems |
---|---|---|---|---|
Air quality | Local regulation of temperature, humidity, and atmospheric chemical composition | Air quality regulation | Air quality regulation | Reduction in air quality and (or) increase in variability of temperature, humidity, etc. |
Biodiversity | Biodiversity in terms of genetic, species, community, or ecosystem increases or reductions in loss | Biodiversity | Biodiversity increase | Reduction of biodiversity |
Biomass | Carbon mass increase; carbon/nitrogen ratio is correlated to biodiversity increases and ecosystem functioning | Biomass provision | Beneficial biomass increases (soil, carbon) and reductions (reduce fire hazards) | Reduction of biomass beneficial to ecosystem functioning |
Climate regulation | GHG emissions reduction, sequestration, leakage reduction; regulation of climate variability | Climate regulation | GHG sequestration and reduction of GHG emissions | Increased GHG emissions and other atmospheric toxins |
Disease | Decrease in disease transfer and presence of disease vectors | Disease reduction through biodiversity | Decrease or elimination of disease | Increased diseases and disease vectors |
Energy | Overall reduction in energy demands or increases to less carbon-intense energy | Energy provision (solar, biomass/fuel, wind, etc.) | — | Increased competition for and consumption of nonrenewable energy resources |
Herbicide and pesticide | Decrease in herbicide and pesticide pollution | Bioremediation of toxins | — | Increased herbicide and pesticide pollution |
Land | Habitat, ecosystem fragmentation, and spatial demand | Land provision | Increase habitat and landscape connectivity | Increased habitat loss and ecosystem fragmentation increase |
Nitrogen fixation | Nitrogen fixation increases; highly related to nutrient management but involves separate functions (fixation vs. avoidance of leakage) | Nitrogen fixation | Nitrogen fixation | Increased nitrogen runoff |
Nutrient management | Cycling, abatement, and reduction of eutrophication and runoff | Nutrient cycling | Abatement of nutrient runoff | Increased nutrient runoff |
Pests | Increase in pest reduction; addresses ecological functions rather than chemical applications | Pest reduction in agriculture | Pest reduction in ecosystem | Increased pests |
Pollination | Intricately linked to biodiversity, but a distinct service provided by pollinators | Pollinator increase in agriculture | Pollinator increase in ecosystem | Reduction of pollinators in ecosystem |
Soil health | Soil structure, fertility, erosion control, disturbance regulation | Soil health improvement | Soil health improvement | Reduction in soil health |
Waste | Reduction of wastes in human food systems through management or biotic and abiotic functions | Bioremediation and abiotic filtration, sequestration, and storage of waste from agriculture | Bioremediation and abiotic filtration, sequestration, and storage of waste from ecosystem | Increased food waste from agriculture and food system inefficiencies |
Water management | Provision, purification, chemical condition, hydrological cycle, sedimentation | Water provisioning and purification; flood mitigation | Hydrological cycle contributions | Water sedimentation and pollution |
Note
GHG, greenhouse gases.
1
For an extensive definition of these services see: web.archive.org/web/20210425071651/https://biodiversity.europa.eu/ecosystems/mapping-and-assessment-of-ecosystems-and-their-services-maes-1/common-international-classification-of-ecosystem-services-cices
Methods
We follow scoping review methods outlined by Arksey and O’Malley (2005), Tricco et al. (2018), and Sucharew and Macaluso (2019). As illustrated in Fig. 2, the process included (i) identifying research questions; (ii) using exclusion criteria and duplicate elimination to conduct keyword searches in electronic databases, reviewing article reference lists, and performing full-text reviews; (iii) thematic coding; and (iv) reporting results. Each step of the review process is detailed below.
Fig. 2.

Identification of research question(s)
The guiding questions listed in the previous section are based on our previous research on the environmental impact of novel food products and DATs that revealed limited reliable ecological data modelling and disparate approaches to modelling sustainability and ES (see Glaros et al. 2021).
Identification and screening of records
We conducted a keyword search using the Web of Science (WoS) and Google Scholar. The WoS electronic database was used to identify peer-reviewed literature and conference proceedings. Google Scholar was used to identify highly cited grey literature and additional peer-reviewed literature missing in the WoS database. Keyword combinations focused on commonly used terms and technologies in digital agriculture. Table 2 shows keyword search combinations. We limited our search to 2016–2021 to capture the most recent scientific research in rapidly evolving fields. In WoS, the keyword search was limited to “topic” which only searches titles, keywords, and abstracts (n = 68). In Google Scholar, a black box algorithm searches full documents and ranks search results according to journal rank, author rank, and recent citations (n = 2254).2 Additional records (for example Mosby et al. 2020) (n = 15) were suggested by experts in topical areas.
Table 2.
Keyword 1 | Keyword 2 | Keyword 3 |
---|---|---|
ecosystem service* | precision agriculture | — |
ecosystem service* | smart farming | — |
ecosystem service* | precision farming | — |
ecosystem service* | digital agriculture | — |
ecosystem service* | smart agriculture | digital |
ecosystem service* | blockchain | agriculture |
ecosystem service* | controlled environment agriculture | — |
ecosystem service* | big data analysis | agriculture |
ecosystem service* | genetic engineering | agriculture |
ecosystem service* | Agriculture 4.0 | — |
ecosystem service* | cellular agriculture | — |
ecosystem service* | artificial intelligence | agriculture |
The resulting records (n = 2337) were screened by the inclusion/exclusion criteria listed below.
1.
Scientific quality—peer-reviewed literature in academic journals, peer-reviewed conference proceedings, and highly cited grey literature reports from government agencies and international and national nongovernmental organizations.
2.
Relevancy—the record must address or review causal pathways in which DATs impact or enhance specific ES.
3.
Parsimony—Google Scholar has a high number of irrelevant results, so our evaluation of Google Scholar results focused on the first 100, highly ranked records.
The records resulting from the exclusion process were imported into the reference management software Mendeley wherein duplicates were eliminated using the “merge duplicates” function, resulting in 79 records. Following snowball methods of Klerkx et al. (2019), reference lists of the resulting records were examined for additional pertinent citations. This produced an additional 12 references for a total of 91 records for full-text assessment. The full-text assessment excluded 17 records as they addressed DATs and mentioned but did not adequately address how DATs may impact agriculture–ES interactions. This resulted in 74 records for thematic coding.
Thematic coding
Emergent coding (Altheide et al. 2008) identified themes in the literature. After full-text assessment, coding was conducted in a spreadsheet (Excel) that included technology types and clusters, ES addressed, coder notes, and record citations.
Report findings
The articles were summarized in a report that was circulated by lead authors to experts in the areas of food system research for vetting of preliminary discussion elements. Several experts were included as co-authors because of their substantial contributions.
2
The algorithm is officially described here (web.archive.org/web/20210506202616/https://scholar.google.com/scholar/about.html). In general, we found that after approximately the first 100 records in Google Scholar there were no additional records of interest and a lack of cited materials.
Results
Our results are presented in two subsections. First, we present a quantitative analysis of patterns in the literature. We then overview and discuss evidence of possible DATs interventions in agriculture–ES interactions.
Patterns in the literature
Table 3 displays the number of records (count) that mention each DAT and each ES. Percentages reflect the number of articles out of the 74 coded. Records sometimes note more than one technology or ES so the counts do not sum to 74. The technology “Other” variable grouped together cloud computing, information and communications technologies (ICT), IoT, and other digital technologies related to connectivity in agricultural settings.
Table 3.
Technology | Count | % | Service | Count | % |
---|---|---|---|---|---|
Precision agriculture | 27 | 36 | Nutrient management | 43 | 58 |
Sensors | 25 | 34 | Climate regulation | 31 | 42 |
Genetics | 23 | 31 | Land | 16 | 22 |
Big data | 19 | 26 | Biodiversity | 14 | 19 |
Smart farming | 16 | 22 | Herbicide and pesticide | 13 | 18 |
Artifical intelligence/machine learning | 13 | 18 | Water management | 13 | 18 |
Robotics and automation | 12 | 16 | Pests | 13 | 18 |
Cellular ag | 12 | 16 | Waste | 10 | 14 |
Unmanned aerial vehicles/remote sensing | 12 | 16 | Soil health | 10 | 14 |
Other | 12 | 16 | Disease | 9 | 12 |
Blockchain | 7 | 9 | Energy | 3 | 4 |
Controlled-environment agriculture | 4 | 5 | Air quality | 3 | 4 |
Nitrogen fixation | 2 | 3 | |||
Pollination | 2 | 3 | |||
Biomass | 2 | 3 |
Of the DATs, precision agriculture (PA) (36%), sensors (34%), genetic research (31%), and big data (26%) featured most prominently in the literature. Somewhat surprisingly, relatively few publications explicitly linked ES functioning to agricultural implementation of blockchain or CEA (inclusive of vertical agriculture). Of the ES addressed, nutrient management (58%) and climate regulation (42%) featured most prominently, while pollination (3%), nitrogen fixation (3%), and biomass production (3%) were rarely mentioned in literature on DATs.
The Table 4 and Table 5 crosstabs reveals patterns that must be interpreted in the context of the literature. In Table 4, a crosstab table presents patterns linking specific DATs cited in relation to each ES. The colouring within the table reflects low to high counts of records, with the darkest areas showing above 90th percentile counts. For example, the darker colouring shows that nutrient management and climate regulation (e.g., greenhouse gas (GHG) emission reductions and carbon sequestration) were the most cited ES. Yet, more importantly, the crosstab format exposes associations between ES and DATs. The highest count in Table 4 is for nutrient management and PA (26 articles). This is indicative of a mature literature on the relation of nutrient management and DATs grouped under PA. Similarly, the literature on novel foods focused on the way that cellular agriculture outcompetes modern beef production in terms of environmental sustainability and is presented as a possible pathway to climate regulation (8 articles), better nutrient management (6), and through reduced nutrient runoff resulting from reductions in the land (4) footprint. In Table 5, a crosstab of DATs reveals how technologies were clustered in publications. For example, 17 records addressed both PA and sensors, which makes sense given the use of sensors in many PA technologies.
Table 4.

Table 5.

A significant portion of the literature linking DATs to ES is aspirational about causal pathways between technology adoption and positive outcomes for ES. More specifically, of the 74 documents evaluated, 17 (23%) are commentaries or exploratory reviews of trends in the literature, while the remaining 57 (77%) conduct either primary or secondary research. Of note, the literature on connectivity (specifically distributed digital ledger technology, focused on blockchain) tended to be preliminary and consists mostly of commentaries, reviews, or exploratory models. Literature on novel foods included life-cycle assessments (LCA) but was also not empirically strong as modelling efforts often conflicted. By contrast, the records found in management were mainly empirical research articles.
Digital agricultural technologies and their links to ecosystem services
Emergent coding revealed three clusters of DATs that (i) make farm management more precise (e.g., sensors, AI, robotics), (ii) increase connectivity in the food system (e.g., supply chain management technologies, blockchain, and expansion of ICT), and (iii) create novel foods that replace current, resource-intensive agricultural practices (e.g., cellular agriculture). Some records addressed more than one theme. Of the 74 records coded, most of the records (63 (85%)) address farm management, while novel foods were addressed in 12 (16%) and connectivity in only nine (12%). The clusters of DATs are noted at the top of Table 4 and Table 5, which respectively identify DATs–ES associations and DATs associations with other DATs.
More precise farm management
This theme includes DATs that offer producers more precise ways of managing their farm operations by, for example, giving them the ability to precisely apply and automate inputs as crops need and to integrate complex algorithms into decisions from risk management to marketing and yield calculations in real-time. The literature uses several terms to refer to overlapping groupings of DATs aimed at management—for example, DAR, precision agriculture/farming, smart farming/agriculture, and Agriculture 4.0/5.0. Efforts to differentiate these broad terms often emphasize PA as a site-specific, field-focused set of tools while smart farming integrates, “agronomy, human resource management, personnel deployment, purchases, risk management, warehousing, logistics, maintenance, marketing and yield calculation into a single system” (Schönfeld et al. 2018, p 110) (see also Wolfert et al. 2017). Bertoglio et al. (2021) showed in a bibliometric study that the use of the term smart farming rose in 2012 as climate-smart farming approaches and digital agricultural tools were also on the rise. Yet, the distinction between these terms seems to increasingly blur. Indeed, discourse analysis approaches identify that the use and definition of such terms frame particular power relations within food systems and, in the case of PA, often undervalue environmental outcomes (Duncan et al. 2021). In our review of literature, every article that we coded as smart farming (n = 14) either also interchangeably used the term “precision agriculture” (PA) or heavily cited articles mentioning PA. So, we group together these records below.
PA and smart farming technologies
PA and smart farming technologies make production systems more precise by using advances in Global Navigation Satellite System technologies, big data, and automated robotic farm equipment to improve guidance, recording, and management (“reacting”) technologies (Balafoutis et al. 2017; Finger et al. 2019). Often PA also takes advantage of specialized software, data analytics, and modern information and communication technologies to inform decision-making (Finger et al. 2019). This requires sensors attached to individual animals, plant locations, other geographic locations on farms to gather data. The interweaving of these technologies creates an IoT involving feedback between sensors and equipment such as smart tractors or robotic milking machines to provide real-time automated adjustments that aim to improve agricultural objectives (Schrijver et al. 2016; Tzounis et al. 2017). In addition to use on large grain operations, in North America and Europe, PA techniques are widely applied to dairy production and may have led to improved cow health and reduced environmental footprints (Rotz et al. 2019b). While PA is typically associated with large-scale grain and animal operations, the broad definition of PA as site-specific, sensor-based monitoring and automated adjustment also applies to smaller-scale operations that can afford such technologies (Finger et al. 2019).
PA is associated with sensors, big data, and smart farming (Table 5). Finger et al. (2019) reviewed the quality of evidence in studies on PA and smart farming tools’ ability to improve nutrient management (Q1 and Q3), assist with climate regulation (Q1 and Q3), save water (Q1), reduce herbicide and pesticide (Q3). Regarding herbicides and pesticides, Clapp (2021) argued that DATs may be one reason we have seen increasing glyphosate use rather than the anticipated decrease with DAT adoption. PA and smart farming tools are associated with improved nutrient management (Q1 and Q3) on large farms (Balafoutis et al. 2017) and potentially on small farms (Kanter et al. 2019), potential contributions towards climate regulation through lower reliance on external inputs (Q1) and avoided fuel use (Q3) (Balafoutis et al. 2017; Kakamoukas et al. 2021), and reduction of the land footprint of agriculture (Q1–3) (Capmourteres et al. 2018). PA benefits to ES are most likely to be empirically measurable in large-scale farming operations (such as grain and oilseed) and biophysical monitoring of animals in red meat, poultry, pork, and aquaculture sectors. Yet, smart farming technologies deployed in smaller farms using agroecological approaches may also show measurable enhancements to ES by increasing farm level input efficiency and allowing monitoring of environmental indicators (Wittman et al. 2020). While PA and smart farming literature indicates potential environmental benefits, there are very few empirical studies showing causal inference on specific ES (see Finger et al. 2019). Many of the controlled studies focus on variable rate technology control of nutrient (mainly nitrogen) and water inputs but report only on agricultural productivity instead of environmental outcomes (see for example Khosla et al 2002). Targeted randomized controlled trials that examine specific PA and smart farming technologies while measuring environmental variables would strengthen PA and smart farming claims to benefit ES.
Big data
There is considerable overlap of big data literature examined in this review with sensors, PA, and AI and machine learning. Big data is cited as a way to improve nutrient management through more precise application of nutrients leading to reduction in input demands (Q1) and reduction of nutrient leaking (Q3). Similar dynamics are cited for reduced herbicide and pesticide use (Q3) (Balafoutis et al 2017; Weersink et al. 2018). Big data may also reduce GHG emissions (climate regulation) by using atmospheric and local emission monitoring to inform farm management (Q3) (Delgado et al. 2019). As well, big data can reduce the need for land (Q1) by identifying less profitable areas and allowing the cultivation of more biodiversity (Q2) in agricultural landscapes (Capmourteres et al. 2018). While big data drives the DAR by allowing rapid advances in fields such as genetics and genomics (Zaidi et al. 2020) and CEA (Charania and Li 2020), better models for linking big data sets in PA, precision conservation, and ES modelling are needed (Capmourteres et al. 2018; Khanna et al. 2018; Delgado et al. 2019). Additionally, research gaps include ways in which big data might drive positive management practices for reducing agricultural energy demands and increasing pollination, soil health, nitrogen fixation, local air quality, and pest management.
AI and machine learning
AI and machine learning literature often also cites sensors, big data, robotics and automation, and UAV and remote sensing. AI and machine learning was linked to improvement in nutrient management (Q1 and Q3) as big data, UAV, robotics, and AI and machine learning are used for efficient spot application of nutrients, herbicides, and pesticides (Q3). In addition, UAV, big data, and AI and machine learning were also linked to monitoring that would allow early identification of pests and disease for treatment and limitation of spread in the surrounding ecosystem (Q3) (Gossen and McDonald 2020). In combination with genetic engineering and big data, AI and machine learning accelerates breeding of climate resilient plants (climate regulation) thus enhancing agriculture and ecosystems’ abilities to respond climate variability (Q2) (Harfouche et al. 2019). While AI and machine learning include several applications (such as targeted pest reduction) that have apparent ES benefits (reduced pesticide use), there is a paucity of empirical research linking AI and machine learning to most ES (see Table 5). This sector would benefit from targeted research projects examining how AI and machine learning are used in specific agricultural cases that lead to ES benefits.
Controlled environment agriculture
CEA refers to a continuum of technologies that include greenhouses, container farms, aquaponics operations that integrate vegetable and fish production, and vertical farms. These are all variations of farming that use sensors, timers, lighting, and other technologies to create smart management systems using AI and automation. Academic research on CEA has proliferated3 and there is a rapidly growing business sector with start-ups such as Ostara4 and Freight Farms5 emphasizing high-tech, low environmental impact farming (Charania and Li 2020). Yet, the literature on CEA and ES often relies on hypothetical LCA models (see for example Forchino et al. 2017; Benis et al. 2017, and Wildeman 2020). Empirical studies that directly compare field agriculture to CEA in terms of ES benefits (see for example Newell et al. 2021 on hydroponic fodder) are rare. We found only four records that mention CEA directly addressing ES, but numerous CEA publications (and business media) that assume environmental benefits from CEA.
Regardless of the specifics of the CEA application, most studies of CEA find them to have hypothetically more efficient nutrient management (Q1 and Q3), herbicide and pesticide use (Q3), and water management (Q1 and Q3) when water cycling is used. There is a hypothetical argument regarding climate regulation (Q2 and Q3) based on potential reduction of food miles, but only if CEA are geographically distributed. Yet, Benis et al. (2017) showed in a LCA that depending on surrounding climatic factors some CEA operations may increase GHG emissions relative to field agriculture. There is also a hypothetical argument about CEA creating a lower land footprint (Q1 and Q2) that may lead to land sparing for conservation based on higher production efficiency per square meter and averted agricultural land conversion. This argument does not consider high energy demands (light, heating, and cooling) leading to increased GHG emissions and upstream impacts of energy generation on land, biodiversity, and other ES (Benis et al. 2017; Newell et al. 2021; Beacham et al. 2019). Additionally, much of the adoption of CEA may not be averting existing agricultural land use or new conversion. For example, Freight Farms emphasizes that many of its adopters have little agricultural experience and choose CEA in extreme conditions (e.g., Alaska and dense urban areas). The literature widely recognizes that these hypothetical ES benefits are highly context dependent and require renewable energy to lower the impacts of CEA high energy demand. The assumption that CEA will replace a substantial amount of any agricultural sector and benefit ES, therefore, remains speculative and requires more empirical research.
Robotics and automation
As shown in Table 5, robotics and automation are highly associated with AI and machine learning, sensors, and big data. In field agriculture, robotics use in relation to ES emphasizes autonomous field equipment that can precisely apply nutrients (nutrient management) (Q1 and Q3) and herbicides and pesticides (Q3) or may completely eliminate the use of herbicides and pesticides (Q3) through the use of lasers for weed control (Duckett et al. 2018; Gossen and McDonald 2020). In addition, robotics contribute to better water management (Q1 and Q3) through optimization of irrigation systems, lower energy demand (Q1), and GHG emissions (climate regulation) (Q3) by electrifying farm vehicles and implements, and increase soil health (Q2) as the autonomous field units cause less compaction than larger tractors and conventional equipment (Duckett et al. 2018). When robotics are deployed as part of CEA, they may contribute to land-use optimization (Q1) and disease reduction (Q2 and Q3) by reducing interface with the surrounding environment (Charania and Li 2020). While research on field applications of robotics and automation shows benefits to ES, the purported benefits of these technologies in CEA suffers from the same caveats of the overall CEA literature—lack of empirical research verifying supposed ES benefits of creating CEA rather than pursuing field agriculture.
Genetics
Agricultural genetics (and genomics) reduce variability in agricultural production by targeting genetic modifications that enhance desirable traits that, for example, increase production potential, lower pest damage, and increase storage times. This field’s most numerous overlaps with other technologies are with robotics and automation and cellular agriculture. The association with cellular agriculture is likely a result of the novel foods literature examining cellular agriculture and advances in genetic engineering in the same publications. Genetics is highly associated with the management DAT cluster, but is also the second highest contributor to the novel foods cluster. While agricultural genetics includes traditional agriculture selection and crop breeding practices, we focused our search on new genetic engineering approaches. Following NASEM (2016), we use the term “genetic engineering” to refer to transgenic techniques (recombinant DNA), somatic cell transfer, genome editing (CRISPR)/Cas9 nuclease system), RNA-based approaches (e.g., dsRNA, mRNA, RNAi), synthetic chromosomes, and target epigenetic modification. In plants, such modifications have typically targeted pesticide tolerance but are now used to increase yield, nutritional content, stress tolerance, medicine production, and pest resistance (NASEM 2016). Animal modifications often target objectives such as increased growth, increased production (e.g., dairy and wool), and disease resistance (Forabosco et al. 2013; Long 2014; Lievens et al. 2015). Advances in agricultural genetics have been accelerated by DAR technologies such as AI, robotics, and big data (Harfouche et al. 2019).
Agricultural genetics includes some of the most scientifically supported and promising benefits to ES. These benefits include resource efficiencies such as with nutrient-use (nutrient management) (Q1 and Q3) (NASEM 2016; Brookes and Barfoot 2018) and water-use efficiency (water management) (Q1 and Q3). GE may lead to reduced land footprint (Q1) due to increased nutritional quality of forage and (theoretically) avoided agricultural land expansion (Brookes and Barfoot 2017; NASEM 2016). Waste reduction (Q1 and Q3) within agriculture may be reduced through increased storage times, thus leading to reduced need for inputs and harmful impacts on the environment (NASEM 2016). GE may benefit ecosystems by increasing biodiversity (Q2) in plants engineered for climate variability, drought tolerance, salinity tolerance, and disease/pest resistance in plants (e.g., species restoration of blight-resistant Castanea dentata) (NASEM 2016) and pollinators (pollination) (Q1 and Q2) (Greenlight Biosciences 2021). Furthermore, GE enables climate regulation through reduced methane production (Q2 and Q3) from better-quality forage (NASEM 2016), enhanced soil carbon sequestration, and avoided tractor fuel use (NASEM 2016). Other ES-related benefits include enhanced photosynthesis (biomass) (Q1) (NASEM 2016; Pellegrino et al. 2018), enhanced nitrogen fixation (Q2) (NASEM 2016), disease resistance (Q2 and Q3) (Zaidi et al 2020), pest resistance (Q2 and Q3) (Mezzetti et al. 2020), and reduced herbicide and pesticide use (Q3) (Zhang et al. 2017; Rodrígues and Petrick 2020).
Sensors
Types of sensors include optical (remote observations of farmlands), mechanical (measuring soil compaction), location-based (recording latitude and longitude), dielectric soil moisture, electrochemical (measuring pH and soil nutrients), and airflow sensors. While sensors are common in most of the DATs, sensors were most often mentioned in cases of field-based nutrient management (Q1 and Q3) (see for example Li et al. 2016) in AI and automation applications (Talaviya et al. 2020) and monitoring emissions for climate regulation (Q2) (see Balafoutis et al. 2017). Continued development of micro and nanosensors that are more sensitive and smaller but have stronger connectivity than existing sensors creates big data for agriculture and drives most of the technologies mentioned above (Fraceto et al. 2016).
UAVs and remote sensing
Remote sensing uses a diverse set of active and passive sensors such as LiDAR and radar in addition to passive sensors such as spectrometers and radiometers to image and model the Earth’s surface. In agriculture, remote sensing is used to monitor geographic areas and create data that can inform decisions. Remotely sensed data can come from satellites, aircraft, or UAVs. UAVs are used to monitor plant health, apply pesticides and herbicides, and collect a wide array of remotely sensed data that can be used in PA to inform AI and robotics and automation. Recent intensification of the use of UAVs and remote sensing in agriculture have happened as spatial, temporal, and spectral resolutions have increased. This increase makes the data more useful for informing management decisions and monitoring land use, soil health, and crops (Weiss et al. 2020). While UAVs and remote sensing were most cited in reference to nutrient management (Q1 and Q3) in the records we identified, these technologies can contribute to interventions in every ES category. In a systematic review of 211 peer-reviewed papers addressing remote sensing of ES (not limited to agriculture), de Araujo Barbosa et al. (2015) found that remote sensing was being used primarily to monitor and inform planning in food production and climate regulation. However, they also found remote sensing to be used to monitor and inform decisions on a wide variety of ES (see for example, Weiss et al. 2020). This suggests that if better models of interactions between agriculture systems and ES are developed, there is opportunity to expand research using UAV and remote sensing for monitoring and managing agriculture–ES interactions.
Connectivity
Connectivity, enabled by digital technologies, is transforming agricultural systems. We use a broad framing of connectivity as (i) linkages across the supply chain through technologies such as blockchain, (ii) overcoming the digital divide (lack of availability, affordability, and access to connected devices and telecommunications infrastructure), and (iii) building local community connectivity and alternative food networks through digital platforms.
Blockchain
Supply chain management can benefit in multiple ways from digital technology, the most well-known of which are software management systems that manage, track, and help control shipping. More novel technology such as blockchain offers ways of tracking goods that theoretically offer both agricultural producers as well as consumers opportunities for increased transparency, better global market and direct access, alternative financing arrangements, and the chance to add value to food products (Ge et al. 2017; Antonucci et al. 2019; Astill et al. 2019). Blockchain is a distributed digital ledger that is immutable. When made public, the blockchain shows transactions over time that allow traceability and a great level of transparency and efficiency for market participants (Astill et al. 2019). Blockchain is currently being used to provide transparency and efficiency in agri-food supply chains including coffee, tuna, beef, beer, milk, and pasta (Antonucci et al. 2019). In the case of tuna, blockchain is being used to verify sustainability claims about fishing practices (biodiversity) (Q1) (Antonucci et al. 2019). Increases in efficiency and transparency have the potential to reduce GHG emissions (climate regulation) (Q3) and waste (Q3) (Astill et al. 2019) associated with complex food systems. Yet, there is inadequate empirical data supporting any of the above ES benefits in relation to blockchain. Moreover, there may be negative environmental impacts of current “proof-of-work” blockchain mining systems that consume large amounts of energy—blockchain technology may transition to renewable energy sources or to less energy intensive “proof-of-stake” frameworks to reduce energy demands, emissions, and related ecosystem impacts (Giungato et al. 2017; Zhang et al. 2020). In summary, research on the benefits of applying blockchain to agricultural interactions with ES and overall sustainability requires more empirical work.
Other connectivity technologies
ICT that increase human connections, mobile technology use, cloud computing, and supply chain management options compromise most records in this category. This type of connectivity has a necessary role as it enables many of the DATs but impacts on ES are not well-established.
Advances in ICT are changing food system dynamics. In 2021, there remains a digital divide in the form of both broadband internet and mobile telecommunications access between rural and urban areas in many countries that requires investment in infrastructure (Ramsetty and Adams 2020; Roese 2021). The Canadian Radio-television and Telecommunications Commission (CRTC) declared broadband internet an essential service in 2016—with minimum standards of 50 megabits per second download and 10 megabits per second upload (Ruimy 2018). The government launched a CAD$1.75 billion fund to connect 98% of Canadians to high-speed internet by 2026, and 100% by 2030 (CBC News 2020). By 2020, 45.6% of Canadian rural communities had access to broadband at high speed and 97.4% of these communities had access to Long Term Evolution (LTE) mobile services with 4G wireless access. Broadband internet and mobile technology may benefit all scales of producers by allowing more advanced farm management systems to link local sensors and machines into larger networks. Moreover, this connectivity may enhance the ability of small and medium producers to source inputs, access information and financial services, and sell directly to retail consumers and wholesalers (Quandt et al. 2020; Krell et al 2021). There is a need for more empirical evidence on how connectivity such as mobile services impact pricing, production strategies, and decisions regarding ES (Baumüller 2018).
While expanded ICT connectivity and social media access have increased producers’ ability to organize alternative, regional, and ecologically focused agricultural movements (Stevens et al. 2016), the relevance of online connectivity made especially clear during COVID-19 (Weersink et al. 2021). The COVID-19 crisis saw increasing demand for online food platforms (Change and Meyerhoefer 2020; Thilmany et al. 2021) and increasing relevance of DATs to food system functioning (Fairbairn and Guthman 2020). Having an internet presence and the ability to interact online to receive orders and communicate with customers allowed local producers overwhelmed by increased direct demand (Coppolino 2021) to respond and reorient perishable products in wholesale operations to direct to consumer sales (Thilmany et al. 2021). While there was limited time to scale-up production, digital connectivity increased the flexibility and resilience of producers and the entire food system during the pandemic (Coppolino 2021; Stoll et al. 2021).
While there is not sufficient empirical evidence to support links between these connectivity DATs and ES, theoretically they may (i) reduce food waste (Q3), (ii) optimize transport to lower emissions (climate regulation) (Q3), and (iii) provide opportunities to transparently link environmentally friendly producers with companies and individual consumers thus creating market-based incentives (and possible certification strategies) for agricultural practices that reduce input ES demand and waste (Q1–3). These interactions seem logical, but more empirical research is needed to show measurable benefits to ecosystems and ES.
Novel foods
A third area in which the DAR is poised to benefit ES is in the creation of novel foods that have smaller environmental footprints than contemporary alternatives (Broad 2019; van der Weele et al. 2019). Cellular agriculture, genetics, and 3-D printing approaches draw from big data, computing power, AI, and automation to produce foods that seek to displace conventional products. In creating clusters for this review, genetic engineering was the second highest contributor to this theme, but it was highly associated with management techniques, so we set aside genetics under management. 3-D printing creates customized food items using a mix of traditional and nontraditional ingredients. It is currently being used to create novel food products that replace animal-derived products such as hamburgers (Sun et al. 2015). In 2021, the technology became commercially available as Redefine Meat obtained $29 million for the launch of its industrial-scale digital manufacturing technology that allows 3-D printers to create steaks using exclusively proteins and fats from legumes and grains.6 While there is potential that this technology may benefit ES, we found no empirical research directly linking 3-D printing of foods with ES benefits.
Cellular agriculture
Cellular agriculture uses tissue engineering or fermentation-based processes to produce protein-containing products and tissue-based foods such as meat, fish, and dairy. The primary distinction between tissue engineering and fermentation processes is that tissue engineering uses cells or cell lines from living animals and fermentation does not use such materials. In both cases, protein products mimicking animal muscle and products (e.g., milk) are produced from either cell cultures or bioreactors. Digital technologies allow for precise identification of cellular data needed to produce new products. The cost of producing such products is falling on an exponential curve, suggesting that price parity with animal production is rapidly approaching. Experts suggest that high-volume production using serum-free media is rapidly approaching feasibility (Post et al. 2020).
How these novel foods may impact ES is less clear. The main argument in the literature is that conventional livestock production has a disproportionately large impact on ES due to the GHG emissions caused by clearing forests for pastures, raising grain to feed livestock, and methane emission from ruminant animals (Willett et al. 2019). The energy necessary to produce cultured meat at scale, and how that compares with conventional meat production, is still unclear in scientific literature as LCAs tend to be highly speculative, use incompatible models, compare cultured protein only with ground beef, and use conflicting model assumptions (Mattick et al. 2015; Lynch and Pierrehumbert 2019; van Eenennaam 2019; Glaros et al. 2021). Furthermore, the technology for cellular agriculture is evolving so quickly that LCAs may quickly become out of date. Cultured dairy substitutes are moving rapidly to scale and are already sold in some regional and national markets (e.g., Perfect Day cultured nondairy ice cream).7 Yet, as of 2021, no LCA details potential environmental impacts of switching to cultured dairy substitutes versus conventional dairy products, while it is widely assumed that this alternative pathway would significantly reduce the number of emissions and other environmental impacts inherent to dairy production (GRAIN and IATP 2018). As mentioned above, even the climate regulation and energy demand benefits require assuming that low-carbon energy sources are readily available (Alexander et al. 2017; Lynch and Pierrehumbert 2019).
Given the state of the literature and LCAs that purport to detail cellular agriculture’s environmental impacts, the ES benefits of cellular agriculture are not empirically well supported. There is a need for more systematic and comparable models for LCA on cellular agriculture and the products that are supposed to lose market share. In addition, the field suffers from some of the modelling flaws mentioned above in that consumers are assumed to make a replacement choice between conventional meats/by-products and these novel foods. Yet, people may simply continue consuming conventional meats/by-products while adding these novel foods to their diets. More social and environmental research is required for cellular agriculture.
3
See the University of Guelph’s Controlled Environment Systems Research Facility web.archive.org/web/20210420083842/http://www.ces.uoguelph.ca/
Discussion
This discussion proceeds in two parts. First, we reflect on the need for research that better models agriculture–ES interactions—a prerequisite for measuring DATs as an intervening variable. Second, we explore barriers that may limit DATs’ potential to enhance ES and reduce negative impacts on ES.
The need to better model agriculture systems and ecosystem services
Throughout the literature, difficulties of modelling agriculture–ES interactions create complications for identifying how DATs may intervene in these interactions. As with attempts to link ES to food security (Cruz-Garcia et al. 2016) and economic modelling (Khanna et al. 2018), existing models of agriculture–ES interactions were often undermined by a lack of empirical data. Below, we outline a few common major difficulties for modelling and indicate research possibilities.
First, as mentioned above and illustrated in Fig. 1, agriculture–ES interactions are multidirectional and complex. Agriculture relies on and may harm ES, yet it can enhance services such as carbon sequestration (Yin et al. 2020) and biodiversity (Leroy et al. 2018). ES make agriculture possible, yet cause disservices that reduce agriculture system’s provisioning services—quantity, quality, and diversity of food, fuel, and fibre (Swinton et al. 2007; Zang et al. 2007; TEEB 2018; Bennett et al. 2021). This complexity allows seemingly contradictory entries to coexist in Fig. 1—such as agriculture’s ability to provide landscape connectivity as a service to ecosystem functioning, while simultaneously threatening to fragment landscape connectivity as a disservice to ecosystem functioning (Mitchell et al. 2018). Understanding how DATs influence agriculture–ES interactions heavily relies on context; for example, cellular agriculture production of cultured meats may not reduce GHG emissions more than conventional beef production systems unless low-cost, low-carbon energy generation can be reliably sourced (Alexander et al. 2017; Lynch and Pierrehumbert 2019). Systems models simultaneously show services and disservices depending on scale, time frame, context-accessible resources, and management practices (Saunders et al. 2016; Campagne et al. 2018; TEEB 2018). Empirical research currently lacks the tools needed to measure and monitor multiple ES across temporal and spatial scales and align ES variables with decision-makers’ management decisions (Bennett et al. 2021). There is a need to support systems modelling approaches that can contextualize and situate research on these interactions given the identified limiting variables.
Second, it is difficult to generalize models of trade-offs and synergies between services or bundles of services in different types of agricultural landscapes because of their multidirectional interactions (Bennett et al. 2009; Raudsepp-Hearne et al. 2010), the possibility of reversibility (i.e., permanency of change of an ES) (Rodriguez et al. 2010), and because management decisions in different agricultural sectors have different outcomes on environmental and ES indicators (Clark and Tilman 2017). Despite a recognition that ES are interlinked with each other, most empirical studies linking agricultural practices to ES or ecosystem impacts focus on one variable such as water quality, soil carbon sequestration, nutrient management, or GHG emissions (Khanna et al. 2018; Bennett et al. 2021). Measuring the impacts DATs have on ES trade-offs requires adjusting models and empirical research to diverse types of agriculture, spatial and temporal scales, ES flows over landscapes, nonlinear causal pathways, positive feedback loops, and system thresholds (Dale and Polasky 2007; Schröter et al 2018; Bennett et al. 2021). Future research that addresses the above variables in site-specific, empirical research and as part of larger modelling initiatives can inform how DATs serve as intervening variables in ES–agriculture interactions.
Third, the implementation of DATs can lead to counterintuitive outcomes for ecosystems and ES in the context of complex drivers of agricultural management decisions. These outcomes may undermine model assumptions. For example, the PA literature often assumes farmers are incentivized towards environmental stewardship or that they are rational decision-makers (Khanna et al. 2018). However, human behaviour is more complex. The literature documents the “misuse of data” wherein farmers “utilize precision technology to apply more nitrogen (N) fertilizer to low-yielding portions of rain-fed fields in the hope of increasing yields, rather than less N to avoid fertilizer losses through leaching and runoff of N that crops cannot use” (Basso and Antle 2020, p 254). As well, there may be cases of “lock-in” wherein producers’ sunk costs in technology lead to continued degradation of resources unless some other policy or market incentive intervenes—yet these scenarios may be highly resilient to external forces (Allison and Hobbs 2004; Bennett et al. 2021). This last scenario indicates a clear need to better understand key leverage points for intervening in agriculture–ES interactions. Moreover, it points to a need for more efforts to contextually understand and describe changes that digitalisation produces on management decisions and consequences for agriculture–ES interactions (see for example Rijswijk et al. 2021 for an attempt to create a socio–cyber–physical system framework for agriculture).
The underlying complexity of agriculture–ecosystem interactions, difficulty of modelling ES trade-offs/synergies resulting from DATs, and lack of empirical evidence supporting the assumptions that DATs are used to benefit ES reveal that this is a rich area of research that requires a nuanced approach to temporal and spatial scales, reversibility, management practices, and other context-dependent factors.
Barriers that inhibit the DAR from enhancing ES
This review identifies four key barriers to deploying DATs in such a way as to ensure they benefit ES: (i) economic and governance systems that do not promote ES; (ii) data management, governance, and cybersecurity; (iii) sociocultural resistance (to novel foods); and (iv) training and human resources.
Economic and governance barriers
Technologies are enabled and constrained by the sociopolitical and financial systems in which they are embedded. Mosby et al. (2020) pointed out that farmers may not necessarily use DATs to enhance ES unless they receive financial rewards to do so—that simply being aware of inefficiencies on the farm is not enough to provide motivation for good management. This point was elaborated by Pannell (2017) who reviewed the economic logic of PA tools that allow for the precise use of inputs. Pannell noted that while there are optimal levels of nitrogen application that maximize yield while minimizing waste, these “payoff curves” (defined as the relationship between inputs applied and yields) are quite flat. This suggests that farmers pay little in the way of penalties if they over or under apply inputs such as fertilizers. Thus, the potential benefits of DATs may not provide sufficient economic payoffs to farmers to justify the expense of investing in these technologies. Higgins et al. (2017) showed that investing in new DATs when harvest yield risk is unknown and new technologies’ data formats are not interoperable can also hinder agriculture technology adoption. These strains of research on farmer behaviour suggest that economic incentives and disincentives are needed to ensure technology is deployed to create positive outcomes for the environment. The adoption of DATs that benefit ES requires a long-term process of scholars, stakeholders, and decision-makers engaging in co-development of effective solutions that address diverse needs (Bennett et al. 2021).
Data governance
Virtually all the technologies described as part of the DAR depend on large volumes of data that must be integrated together to produce the insights farmers need to reduce their environmental footprint. In this way, the DAR is no different than similar technological transformations that have unfolded in IT, transportation, or medicine. Data-related challenges are identified in at least three key areas. First, data that come from diverse sources must be interoperable. This requires protocols that allow remote sensing, market, soil, weather, and harvest data to be standardized and harmonized so that algorithms, operations, and apps can access and use the data (Wolfert et al. 2017). Second, there is an urgent need for data governance that addresses privacy protection, data ownership, and compensation for farmers when companies use their field data to improve the performance of private sector technologies (Jakku et al. 2019). Rotz et al. (2019b) pointed out that farmers are justifiably concerned when the data they generate is sold back to them in the form of decision-support tools. Third, as agricultural digitalization occurs these systems also become susceptible to cybersecurity vulnerabilities (Barreto and Amaral 2018; Nikander et al. 2020). Organized, high profile cyber-attacks by hacker groups such as Revil on agricultural businesses such as JBS S.A. (the largest beef producer in the world) and Agromart in Canada are raising alarms about the lack of cybersecurity throughout the agricultural supply chain.8 Such issues must be addressed if DATs are to be adopted to enhance ES.
Sociocultural nature of food
While novel foods and food production strategies may offer environmentally friendly ways of restructuring food systems, it is well documented that many novel technologies may not be readily accepted by communities—whether it be a subtle shift in colour or texture or a move to GE foods (Kuhnlein and Receveur 1996; Finucane and Holup 2005). In many ways, these challenges are somewhat intractable and different in nature than other technological, governmental, or economics of scale issues.
Training, skills, and labour
Labour and training gaps stand in the way of deploying DATs in a way that helps enhance ES. Demographic dynamics of the agricultural sector make investing in modern technologies challenging. The average age of farmers in North America is rising (in Canada it is 55 years old) and 70% of farmers do not have succession plans (Beaulieu 2014). There is an urgent need to assess agricultural labor shortages and train digital agriculture workers in Canada (Stackhouse et al. 2019). Federal reports argue that promoting environmental sustainability is integral to the identity of the Canadian agri-food sector (Advisory Council on Economic Growth 2017; Senate of Canada 2019). Taken together, these reports suggest that there is a skilled labour shortage in the agri-food sector in Canada and a need to invest in sustainability and technology training in post-secondary agricultural programs.
Conclusions: implications for Canadian agriculture
The above four challenges suggest that while DATs hold promise to enhance ES, technology on its own is insufficient to reduce the food system’s environmental footprint. Hoped-for benefits remain largely hypothetical without sustained investment in technical training, policies to incentivize DATs adoption, and further research on the sectoral and regional use scenarios for DATs.
Canadian policies that support digital agricultural innovation should be explicit about the role of specific DATs in transition pathways to more sustainable agriculture futures. This would allow governments to introduce targeted policies (e.g., carbon markets and sector-specific interest rates) and promote targeted investments in education and DAT use scenarios that benefit ES. For example, investments in university research capacity on cellular agriculture and a geographically distributed network of bioreactor centres fueled by renewable energy could position Canada as a cellular agriculture leader while potentially providing ES benefits.
Canadian policies should recognize that DATs present an opportunity to pursue diverse agricultural futures that destabilize unsustainable current food system practices (Klerkx and Rose 2020). A more sustainable and just digital agriculture transition requires engaging with small producers and alternative agroecological approaches that directly support the health of ecosystems and human communities (Wittman et al. 2020; Rose et al. 2021). Canada has a diversity of different types of farming systems, farm sizes, and sectoral concerns across biogeoclimatic and political regions. This diversity needs to be recognized in future research on DATs and ES so that the digital transition in agriculture does not simply intensify and reproduce existing industrial production models (Lajoie-O’Malley et al. 2020). Research that outlines more nuanced understandings of different farmers (e.g., conventional large scale, conventional small scale, traditional, and artisanal) and their decision matrices will allow more targeted interventions to adopt, adapt, and implement DATs that can enhance ES.
To make adequate and strategic policy recommendations on DATs and ES, regional evaluations that involve input from industry, market, academic, and other key participants in the food system such as Dhillon et al. (2020) outlined in British Columbia should be pursued within other provinces and territories. That being said, our scoping review indicates that some DAT are relevant across sectors and may be strategic for the future of agriculture in Canada (e.g., domestic investment in sensor technologies or genetics). Moreover, some DAT clusters such as AI, machine learning, and robotics could form the basis of policies and investments that create synergy between sectors and regions (e.g., from field-based PA to container-based CEA). Most importantly, identifying ES management challenges in unique regions and agricultural sectors would help align evidence-based, targeted adoption of specific DATs that can meet those challenges. Regional and sectoral evaluation may want to use these latter suggestions to frame recommendations for targeted interventions.
The technologies discussed under the banner of the DAR are no panacea. While the DATs discussed here have the potential to allow producers and processors to create high-quality and safe food on less land with fewer inputs, such outcomes are not inevitable. Canada may become a leader in sustainable farming only if adequate empirical research is undertaken to identify the most effective DATs for each sector, there is sustained funding of economic incentives, the diversity of agricultural producers in Canada are acknowledged, and institutions prioritize training in digital technologies for the agricultural sector.
Acknowledgements
This work was supported by ResNet (a NSERC Strategic Partnership Grant for Networks) and the Food from Thought Program at the University of Guelph funded by the Canada First Research Excellence Fund.
References
Advisory Council on Economic Growth. 2017. Unleashing the Growth Potential of Key Sectors. Ottawa. [online]: Available from web.archive.org/web/20201116035610/https://www.budget.gc.ca/aceg-ccce/pdf/key-sectors-secteurs-cles-eng.pdf.
Alexander P, Calum B, Almut A, Clare D, John F, Dominic M, and Mark DAR. 2017. Could Consumption of Insects, Cultured Meat or Imitation Meat Reduce Global Agricultural Land Use? Global Food Security, 15: 22–32.
Allison HE, and Hoobs RJ. 2004. Resilience, adaptive capacity, and the ‘Lock-in Trap’ of the Western Australian Agricultural Region. Ecology and Society, 9 (1).
Altheide D, Coyle M, DeVriese K, and Schneider C. 2008. Emergent qualitative document analysis. In Handbook of emergent methods. Edited by SN Hesse-Biber and P Leavy, The Guilord Press. New York. pp. 127–151.
Antonucci F, Simone F, Corrado C, Federico P, Luciano R, and Paolo M. 2019. A review on blockchain applications in the agri-food sector. Journal of the Science of Food and Agriculture, 99(14): 6129–6138.
Arksey H, and O’Malley L. 2005. Scoping studies: Towards a methodological framework. International Journal of Social Research Methodology: Theory and Practice, 8(1): 19–32.
Assandri G, Giuseppe B, Paolo P, and Mattia B. 2018. Beautiful agricultural landscapes promote cultural ecosystem services and biodiversity conservation. Agriculture, Ecosystems and Environment, 256: 200–210.
Astill J, Dara RA, Campbell M, Farber JM, Fraser EDG, Sharif S, and Yada RY. 2019. Transparency in food supply chains: a review of enabling technology solutions. Trends in Food Science and Technology, 91: 240–247.
Baer SG, and Birgé HE. 2018. Soil ecosystem services: An overview. In Managing soil health for sustainable agriculture volume 1: Fundamentals. Edited by D Reicosky. Burleigh Dodds Science Publishing Limited. Cambridge. pp. 1–24.
Balafoutis A, Beck B, Fountas S, Vangeyte J, Wal T, Soto I, et al. 2017. Precision agriculture technologies positively contributing to ghg emissions mitigation, farm productivity and economics. Sustainability, 9(8): 1339.
Barreto L, and Amaral A. 2018. Smart farming: Cyber security challenges. 9th International Conference on Intelligent Systems 2018: Theory, Research and Innovation in Applications, IS 2018 - Proceedings, 870–876.
Basso,B. and Antle J. 2020. Digital agriculture to design sustainable agricultural systems. Nature Sustainability, 3(4): 254–256.
Baumüller H. 2018. The little we know: An exploratory literature review on the utility of mobile phone-enabled services for smallholder farmers. Journal of International Development, 30(1): 134–54.
Beacham AM, Vickers LH, and Monaghan JM. 2019. Vertical farming: A summary of approaches to growing skywards. Journal of Horticultural Science and Biotechnology, 94(3): 277–283.
Beaulieu MS. 2014. Demographic Changes in Canadian Agriculture. Statistics Canada. [online]: Available from https://www150.statcan.gc.ca/n1/pub/96-325-x/2014001/article/11905-eng.htm.
Benis, K, Reinhart C, and Ferrão P. 2017. Building-integrated agriculture (bia) in urban contexts: Testing a simulation-based decision support workflow. Building Simulation Conference Proceedings, 5(August): 2337–2346.
Bennett EM., Peterson GD, and Gordon LJ. 2009. Understanding relationships among multiple ecosystem services. Ecology Letters, 12(12): 1394–1404.
Bennett EM, Baird J, Baulch H, Chaplin-Kramer R, Fraser E, Loring P, et al. 2021. Ecosystem services and the resilience of agricultural landscapes. Advances in Ecological Research, 64: 1–43.
Bertoglio R, Corbo C, Renga FM, and Matteucci M. 2021. The digital agricultural revolution: A bibliometric analysis literature review (Preprint). ArXiv:2103.12488. [online]: Available from arxiv.org/abs/2103.12488.
Broad GM. 2019. Plant-Based and cell-based animal product alternatives: an assessment and agenda for food tech justice. Geoforum, 107: 223–26.
Bronson K. 2019. Looking through a responsible innovation lens at uneven engagements with digital farming. NJAS - Wageningen Journal of Life Sciences, 90–91(December): 100294.
Brookes G, and Barfoot P. 2017. GM crops: Global socio-economic and environmental impacts 1996-2015. Dorchester. [online]: Available from web.archive.org/web/20200615073429/https://www.pgeconomics.co.uk/pdf/2017globalimpactstudy.pdf.
Brookes G, and Barfoot P. 2018. Environmental impacts of genetically modified (GM) crop use 1996-2016: Impacts on pesticide use and carbon emissions. GM Crops and Food, 9(3): 109–39.
Campagne CS, Roche PK, and Salles JM. 2018. Looking into pandora’s box: Ecosystem disservices assessment and correlations with ecosystem services. Ecosystem Services, 30: 126–136.
Capmourteres V, Adams J, Berg A, Fraser E, Swanton C, and Anand M. 2018. Precision conservation meets precision agriculture: A case study from southern Ontario. Agricultural Systems, 167: 176–185.
CBC News. 2020. Trudeau promises to connect 98% of Canadians to high-speed internet by 2026. CBC News. [online]: Available from https://www.cbc.ca/news/politics/broadband-internet-1.5794901.
Chang H-H, and Meyerhoefer C. 2020. Covid-19 and the demand for online food shopping services: empirical evidence from taiwan. Working Paper 27427. National Bureau of Economic Research. Cambridge. [online]: Available from web.archive.org/web/20200824050536/https://www.nber.org/papers/w27427.pdf.
Charania I, and Li, X. 2020. Smart farming: Agriculture’s shift from a labor intensive to technology native industry. Internet of Things, 9: 100142.
Clapp J. 2021. Explaining growing glyphosate use: The political economy of herbicide-dependent agriculture. Global Environmental Change, 67 (February): 102239.
Clark M, and Tilman D. 2017. Comparative analysis of environmental impacts of agricultural production systems, agricultural input efficiency, and food choice. Environmental Research Letters, 12(6).
Coppolino A. 2021. Farm store and online sales shoot up as farmers pivot due to pandemic. 2021. [online]: Available from cbc.ca/news/canada/kitchener-waterloo/andrew-coppolino-farm-online-produce-sale-covid-19-1.5590279.
Cruz-Garcia GS, Sachet E, Vanegas M, and Piispanen K. 2016. Are the major imperatives of food security missing in ecosystem services research? Ecosystem Services, 19: 19–31.
Dale VH, and Polasky S. 2007. Measures of the effects of agricultural practices on ecosystem services. Ecological Economics, 64(2): 286–296.
Delgado, JA, Short NM, Roberts DP, and Vandenberg B. 2019. Big data analysis for sustainable agriculture on a geospatial cloud framework. Frontiers in Sustainable Food Systems 3(July).
de Araujo Barbosa CC, Atkinson PM, and Dearing JA. 2015. Remote sensing of ecosystem services: A systematic review. Ecological Indicators 52: 430–43.
De Clercq M, Vats A, and Biel A. 2018. Agriculture 4.0: The future of farming technology. World Government Summit Report. [online]: Available from https://www.oliverwyman.com/our-expertise/insights/2018/feb/agriculture-4-0--the-future-of-farming-technology.html.
Dhillon P, Newman L, and Gupta A. 2020. The future of B.C.’s food system. Victoria, British Columbia. [online]: Available from engage.gov.bc.ca/app/uploads/sites/121/2020/01/FSTF-Report-2020-The-Future-of-Food.pdf.
Duckett T, Pearson S, Blackmore S, Grieve B, Chen W-H, Cielniak G, Cleaversmith J, et al. 2018. Agricultural robotics: The future of robotic agriculture. London. [online]: Available from arxiv.org/abs/1806.06762.
Duncan E, Glaros A, Ross DZ, and Nost E. 2021. New but for whom? Discourses of Innovation in precision agriculture. Agriculture and Human Values, 1–19.
Fairbairn M, and Guthman J. 2020. Agri-Food Tech discovers silver linings in the pandemic. Agriculture and Human Values, 37(3): 587–588.
Finger R, Swinton SM, Benni NE, and Walter A. 2019. Precision farming at the nexus of agricultural production and the environment. Annual Review of Resource Economics, 11: 313–335.
Finucane ML, and Holup JL. 2005. Psychosocial and cultural factors affecting the perceived risk of genetically modified food: an overview of the literature. Social Science and Medicine, 60(7): 1603–1612.
Foley JA, Ramankutty N, Brauman KA, Cassidy ES, Gerber JS, Johnston M, et al. 2011. Solutions for a cultivated planet. Nature, 478(7369): 337–342.
Forabosco F, Löhmus M, Rydhmer L, and Sundström LF. 2013. Genetically modified farm animals and fish in agriculture: A review. Livestock Science, 153(1–3): 1–9.
Forchino AA, Lourguioui H, Brigolin D, and Pastres R. 2017. Aquaponics and sustainability: The comparison of two different aquaponic techniques using the Life Cycle Assessment (LCA). Aquacultural Engineering, 77: 80–88.
Fraceto LF, Grillo R, de Medeiros GA, Scognamiglio V, Rea G, and Bartolucci C. 2016. Nanotechnology in agriculture: Which innovation potential does it have? Frontiers in Environmental Science, 4(March): 20.
Fraser EDG, and Campbell M. 2019. Agriculture 5.0: reconciling production with planetary health. One Earth, 1(3): 278–80.
Garbach K, Milder JC, DeClerck FAJ, Montenegro de Wit M, Driscoll L, and Gemmill-Herren B. 2017. Examining multi-functionality for crop yield and ecosystem services in five systems of agroecological intensification. International Journal of Agricultural Sustainability, 15(1): 11–28.
Ge L, Brewster C, Spek J, Smeenk A, and Top J. 2017. Blockchain for agriculture and food:Findings from the pilot study. Wageningen Economic Research, [online]: Available from library.wur.nl/WebQuery/wurpubs/fulltext/426747.
Giungato P, Rana R, Tarabella A, and Tricase C. 2017. current trends in sustainability of bitcoins and related blockchain technology. Sustainability, 9(12): 2214.
Glaros A, Marquis S, Major C, Quarshie P, Ashton L, Green AG, et al. 2021. Food frontiers: A review of five promising foods, technologies, techniques and their potential implications for the global food system. Trends in Food Science and Technology.
Godfray HJ, Beddington JR, Crute IR, Haddad L, Lawrence D, Muir JF, et al. 2010. Food security: The challenge of feeding 9 billion people. Science, 327(5967): 812–818.
Gossen BD, and McDonald MR. 2020. New technologies could enhance natural biological control and disease management and reduce reliance on synthetic pesticides. Canadian Journal of Plant Pathology, 42(1): 30–40.
GRAIN, and IATP. 2018. Emissions impossible: How big meat and dairy are heating up the planet. barcelona. [online]: Available from grain.org/media/W1siZiIsIjIwMTgvMDcvMTcvMTdfNTFfMTdfNzgzX0VtaXNzaW9uc19pbXBvc3NpYmxlX0VOXzEyX3BhZ2UucGRmIl1d.
Greenlight Biosciences. 2021. Protecting Honeybees from the Varroa Mite. 2021. [online]: Available from web.archive.org/web/20210520124034/https://www.greenlightbiosciences.com/blog/greenlight-working-to-protect-honeybees-from-the-varroa-destructor-mite/.
Haines-Young, R, and Potschin M. 2018. Common International Classification of Ecosystem Services (CICES) V5.1 Guidance on the Application of the Revised Structure. [online]: Available from web.archive.org/web/20210318042255/https://cices.eu/.
Harfouche AL, Jacobson DA, Kainer D, Romero JC, Harfouche AH, Mugnozza GS, et al. 2019. Accelerating Climate Resilient Plant Breeding by Applying Next-Generation Artificial Intelligence. Trends in Biotechnology, 37(11): 1217–1235.
Higgins V, Bryant M, Howell A, and Battersby J. 2017. Ordering Adoption: Materiality, Knowledge and Farmer Engagement with Precision Agriculture Technologies. Journal of Rural Studies, 55 (October): 193–202.
Howlett BG, Todd JH, Willcox BK, Rader R, Nelson WR, Gee M, et al. 2021. Using Non-Bee and Bee Pollinator-Plant Species Interactions to Design Diverse Plantings Benefiting Crop Pollination Services. Advances in Ecological Research, 64: 45–103.
Jakku E, Taylor B, Fleming A, Mason C, Fielke S, Sounness C, and Thorburn P. 2019. If they don’t tell us what they do with it, why would we trust them?’ trust, transparency and benefit-sharing in smart farming. NJAS - Wageningen Journal of Life Sciences, 90–91(December): 100285.
Jiren TS, Dorresteijn I, Schultner J, and Fischer J. 2018. The governance of land use strategies: Institutional and social dimensions of land sparing and land sharing. Conservation Letters, 11(3): 1–8.
Kakamoukas G, Sarigiannidis P, Maropoulos A, Lagkas T, Zaralis K, and Karaiskou C. 2021. Towards climate smart farming—a reference architecture for integrated farming systems. Telecom, 2(1): 52–74.
Kanter DR, Bell AR, and McDermid SS. 2019. Precision Agriculture for Smallholder Nitrogen Management. One Earth, 1(3): 281–284.
Khanna M, Swinton SM, and Messer KD. 2018. Sustaining our natural resources in the face of increasing societal demands on agriculture: Directions for future research. Applied Economic Perspectives and Policy, 40(1): 38–59.
Khosla R, Fleming K, Delgado JA, Shaver TM, and Westfall DG. 2002. Use of site-specific management zones to improve nitrogen management for precision agriculture. Journal of Soil and Water Conservation, 57(6) 513–518.
Klerkx, L, Jakku E, and Labarthe P. 2019. A review of social science on digital agriculture, smart farming and agriculture 4.0: new contributions and a future research agenda. NJAS - Wageningen Journal of Life Sciences, 90–91(December): 100315.
Klerkx L, and Rose D. 2020. Dealing with the game-changing technologies of agriculture 4.0: how do we manage diversity and responsibility in food system transition pathways? Global Food Security, 24: 100347.
Krell NT, Giroux SA, Guido Z, Hannah C, Lopus SE, Caylor KK, and Evans TP. 2021. Smallholder farmers’ use of mobile phone services in central kenya. Climate and Development 13(3): 215–27.
Kuhnlein HV, and Receveur O. 1996. Dietary change and traditional food systems of indigenous peoples. Annual Review of Nutrition, 16: 417–442.
Lajoie-O’Malley A, Bronson K, van der Burg S, and Klerkx L. 2020. The future(s) of digital agriculture and sustainable food systems: an analysis of high-level policy documents. Ecosystem Services, 45: 101183.
Leroy G, Baumung R, Boettcher P, Besbes B, From T, and Hoffmann I. 2018. Animal genetic resources diversity and ecosystem services. Global Food Security, 17: 84–91.
Li A, Duval BD, Anex R, Scharf P, Ashtekar JM, Owens PR, and Ellis C. 2016. A case study of environmental benefits of sensor-based nitrogen application in corn. Journal of Environmental Quality, 45 (2): 675–83.
Lievens A, Petrillo M, Querci M, and Patak A. 2015. Genetically modified animals: options and issues for traceability and enforcement. Trends in Food Science and Technology, 44(2): 159–176.
Long C. 2014. Transgenic livestock for agriculture and biomedical applications. BMC Proceedings, 8(S4): O29.
Lonkila A, and Kaljonen M. 2021. Promises of meat and milk alternatives: An integrative literature review on emergent research themes. Agriculture and Human Values, 38: 625–639.
Lynch J, and Pierrehumbert R. 2019. Climate impacts of cultured meat and beef cattle. Frontiers in Sustainable Food Systems, 3: 1–11.
MacPherson J, Paul C, and Helming K. 2020. Linking ecosystem services and the sdgs to farm-level assessment tools and models. Sustainability, 12(16): 1–19.
Mattick CS, Landis AE, Allenby BR, and Genovese NJ. 2015. Anticipatory life cycle analysis of in vitro biomass cultivation for cultured meat production in the united states. Environmental Science and Technology, 49(19): 11941–49.
Mezzetti B, Smagghe G, Arpaia S, Christiaens O, Dietz-Pfeilstetter A, Jones H, et al. 2020. RNAi: What is its position in agriculture? Journal of Pest Science, 93(4): 1125–1130.
Miles C. 2019. The combine will tell the truth: on precision agriculture and algorithmic rationality. Big Data and Society, 6(1): 205395171984944.
Mitchell S, Weersink A, and Erickson B. 2018. Adoption of precision agriculture technologies in ontario crop production. Canadian Journal of Plant Science, 98(6): 1384–1388.
Mosby I, Rotz S, and Fraser EDG. 2020. Uncertain harvest: The future of food on a warming planet. University of Regina Press, Regina.
NASEM. 2016. Genetically engineered crops: Experiences and prospects. In Committee on genetically engineered crops: past experience and future prospects, board on agriculture and natural resources, and division on earth and life sciences. National Academies of Sciences, Engineering, and Medicine. The National Academies Press, Washington, D.C.
Newell R, Newman L, Dickson M, Vanderkooi B, Fernback T, and White C. 2021. Hydroponic fodder and greenhouse gas emissions: a potential avenue for climate mitigation strategy and policy development. FACETS, 6(1): 334–357.
Nikander J, Manninen O, and Laajalahti M. 2020. Requirements for cybersecurity in agricultural communication networks. Computers and Electronics in Agriculture, 179: 105776.
Pannell DJ. 2017. Economic Perspectives on Nitrogen in Farming Systems: Managing Trade-Offs between Production, Risk and the Environment. Soil Research, 55(5–6): 473–78.
Pellegrino E, Bedini S, Nuti M, and Ercoli L. 2018. Impact of genetically engineered maize on agronomic, environmental and toxicological traits: A meta-analysis of 21 years of field data. Scientific Reports, 8(1): 1–12.
Phillips PWB, Relf-Eckstein JA, Jobe G, and Wixted B. 2019. Configuring the New Digital Landscape in Western Canadian Agriculture. NJAS - Wageningen Journal of Life Sciences, 90–91(December): 100295.
Post MJ, Levenberg S, Kaplan DL, Genovese N, Fu J, Bryant CJ, et al. 2020. Scientific, sustainability and regulatory challenges of cultured meat. Nature Food, 1(7): 403–415.
Power AG. 2010. Ecosystem Services and Agriculture: Tradeoffs and Synergies. Philosophical Transactions of the Royal Society B: Biological Sciences, 365(1554): 2959–2971.
Quandt A, Salerno JD, Neff JC, Baird TD, Herrick JE, McCabe JT, et al. 2020. Mobile phone use is associated with higher smallholder agricultural productivity in Tanzania, East Africa. PLoS ONE, 15(8 August): 1–16.
Ramsetty A, and Adams C. 2020. Impact of the digital divide in the age of COVID-19. Journal of the American Medical Informatics Association, 27(7): 1147–48.
Raudsepp-Hearne C, Peterson GD, and Bennett EM. 2010. Ecosystem service bundles for analyzing tradeoffs in diverse landscapes. Proceedings of the National Academy of Sciences of the United States of America, 107(11): 5242–5247.
Reid WV, Mooney HA, Cropper A, Capistrano D, Carpenter SR, Chopra K, et al. 2005. Ecosystems and Human Well-Being-Synthesis: A Report of the Millennium Ecosystem Assessment. Assessment of Climate Change in the Southwest United States: A Report Prepared for the National Climate Assessment. Island Press, Washington, D.C.
Rijswijk K, Klerkx L, Bacco M, Bartolini F, Bulten E, Debruyne L, et al. 2021. Digital Transformation of agriculture and rural areas: a socio-cyber-physical system framework to support responsibilisation. Journal of Rural Studies, 85 (January): 79–90.
Rodrigues TB, and Petrick JS. 2020. Safety considerations for humans and other vertebrates regarding agricultural uses of externally applied RNA molecules. Frontiers in Plant Science, 11(April): 407.
Rodríguez JP, Beard TD, Bennett EM, Cumming GS, Cork SJ, Agard J. et al. 2006. Trade-Offs across Space, Time, and Ecosystem Services. Ecology and Society, 11(1).
Roese J. 2021. COVID-19 exposed the digital divide. here’s how we can close it. World Economic Forum. [online]: Available from web.archive.org/web/20210130043252/https://www.weforum.org/agenda/2021/01/covid-digital-divide-learning-education/.
Rose DC, Wheeler R, Winter M, Lobley M, and Chilvers J. 2021. Agriculture 4.0: Making it work for people, production, and the planet. Land Use Policy, 100: 104933.
Rotz S, Gravely E, Mosby I, Duncan E, Finnis E, Horgan M, et al. 2019a. Automated pastures and the digital divide: How agricultural technologies are shaping labour and rural communities. Journal of Rural Studies, 68: 112–122.
Rotz S, Duncan E, Small M, Botschner J, Dara R, Mosby I, et al. 2019b. The Politics of Digital Agricultural Technologies: A Preliminary Review. Sociologia Ruralis, 59(2): 203–229.
Ruimy D. 2018. Broadband Connectivity in Rural Canada: Submission to the House of Commons Standing Committee on Industry, Science and Technology. House of Commons Standing Committee on Industry, Science and Technology (April).
Saunders ME, Peisley RK, Rader R, and Luck GW. 2016. Pollinators, Pests, and Predators: Recognizing Ecological Trade-Offs in Agroecosystems. Ambio, 45(1): 4–14.
Schönfeld M, Heil R, and Bittner L. 2018. Big data on a farm-smart farming. In Big data in context: legal, social and technological insights. Edited by T Hoeren and B Kolany-Raiser. Springer International Publishing, Cham. pp. 109–120.
Schrijver R, Poppe K, and Daheim C. 2016. Precision agriculture and the future of farming in europe. European Parliament Research Service, Scientific Foresight Unit (STOA).
Schröter M, Koellner T, Alkemade R, Arnhold S, Bagstad KJ, Erb KH, et al. 2018. Interregional flows of ecosystem services: Concepts, typology and four cases. Ecosystem Services, 31: 231–241.
Senate of Canada. 2019. Made in Canada: Growing Canada’s value-added food sector. Ottawa. [online]: Available from sencanada.ca/en/info-page/parl-42-1/agfo-made-in-canada/.
Springmann, M, Clark M, Mason-D’Croz D, Wiebe K, Bodirsky BL, Lassaletta L, et al. 2018. Options for Keeping the Food System within Environmental Limits. Nature, 562(7728): 519–525.
Stackhouse J, Schrumm A, King C, Agopsowic A, Freestone C, and Chow D. 2019. Farmer 4.0. Toronto. [online]: Available from web.archive.org/web/20201104203725/http://www.rbc.com/economics/economic-reports/pdf/other-reports/Farmer4_aug2019.pdf.
Stevens TM, Aarts N, Termeer CJAM, and Dewulf A. 2016. Social media as a new playing field for the governance of agro-food sustainability. Current Opinion in Environmental Sustainability, 18: 99–106.
Stoll JS, Harrison HL, Sousa ED, Callaway D, Collier M, Harrell K, et al. 2021. Alternative Seafood Networks During COVID-19: Implications for resilience and sustainability. Frontiers in Sustainable Food Systems, 5: 614368.
Sucharew H, and Macaluso M. 2019. Methods for research evidence synthesis: The scoping review approach. Journal of Hospital Medicine, 14(7): 416–18.
Sun ZC, Yu QL, and Han L. 2015. The Environmental Prospects of Cultured Meat in China. Journal of Integrative Agriculture, 14(2): 234–240.
Swinton SM, Lupi F, Robertson GP, and Hamilton SK. 2007. Ecosystem Services and Agriculture: Cultivating Agricultural Ecosystems for Diverse Benefits. Ecological Economics, 64(2): 245–52.
Talaviya T, Shah D, Patel N, Yagnik H, and Shah M. 2020. Implementation of artificial intelligence in agriculture for optimisation of irrigation and application of pesticides and herbicides. Artificial Intelligence in Agriculture, 4: 58–73.
TEEB. 2018. TEEB for agriculture and food: Scientific and economic foundations. Edited by A Müller, P Sukhdev, and P Kumar, UN Environment, Geneva, Switzerland.
Thilmany D, Canales E, Low SA, and Boys K. 2021. Local food supply chain dynamics and resilience during COVID-19. Applied Economic Perspectives and Policy, 43(1): 86–104.
Tricco AC, Lillie E, Zarin W, O’Brien KK, Colquhoun H, Levac D, Moher D, et al. 2018. PRISMA extension for scoping reviews (PRISMA-ScR): Checklist and explanation. Annals of Internal Medicine, 169(7): 467–73.
Tzounis A, Katsoulas N, Bartzanas T, and Kittas C. 2017. Internet of things in agriculture, recent advances and future challenges. Biosystems Engineering, 164: 31–48.
Van Berkel, DB., and Verburg PH. 2014. Spatial quantification and valuation of cultural ecosystem services in an agricultural landscape. Ecological Indicators, 37: 163–74.
van der Weele C, Feindt P, van der Goot AJ, van Mierlo B, and van Boekel M. 2019. Meat alternatives: An integrative comparison. Trends in Food Science and Technology, 88: 505–512.
van Eenennaam AL. 2019. Alternative meats and alternative statistics: What do the data say? In Range Beef Cow Symposium XXV, November 18–20, 2019. Mitchell, Nebraska. p. 37–49. [online]: Available from https://biobeef.faculty.ucdavis.edu/2019/12/15/alternative-meats-and-alternative-statistics-what-do-the-data-say-1-of-4/.
Von Döhren P, and Haase D. 2015. Ecosystem disservices research: a review of the state of the art with a focus on cities. Ecological Indicators, 52: 490–497.
Walch K. 2019. How AI Is transforming agriculture. Forbes. 2019. [online]: Available from web.archive.org/web/20210208142832/https://www.forbes.com/sites/cognitiveworld/2019/07/05/how-ai-is-transforming-agriculture/.
Weersink A, Fraser E, Pannell D, Duncan E, and Rotz S. 2018. Opportunities and challenges for big data in agricultural and environmental analysis. Annual Review of Resource Economics, 10: 19–37.
Weersink A, von Massow M, Bannon N, Ifft J, Maples J, McEwan K, et al. 2021. COVID-19 and the agri-food system in the united states and canada. Agricultural Systems, 188: 103039.
Weiss M, Jacob F, and Duveiller G. 2020. Remote sensing for agricultural applications: A meta-review. Remote Sensing of Environment, 236(August 2019): 111402.
Wildeman, R. 2020. Vertical Farming: A Future Perspective or a Mere Conceptual Idea? University of Twente.
Willett W, Rockström J, Loken B, Springmann M, Lang T, Vermeulen S, et al. 2019. Food in the anthropocene: The eat–lancet commission on healthy diets from sustainable food systems. The Lancet, 393(10170): 447–492.
Wittman H, James D, and Mehrabi Z. 2020. Advancing food sovereignty through farmer-driven digital agroecology. International Journal of Agriculture and Natural Resources 47(3): 235–248.
Wolfert S, Ge L, Verdouw C, and Bogaardt MJ. 2017. Big data in smart farming – A review. Agricultural Systems, 153: 69–80.
Yin S, Zhang X, Lyu J, Zhi Y, Chen F, Wang L, Liu C, and Zhou S. 2020. Carbon sequestration and emissions mitigation in paddy fields based on the dndc model: A review. Artificial Intelligence in Agriculture 4: 140–149.
Zaidi SSA, Mahas A, Vanderschuren H, and Mahfouz MM. 2020. Engineering crops of the future: crispr approaches to develop climate-resilient and disease-resistant plants. Genome Biology, 21(1): 1–19.
Zhang A, Zhong RY, Farooque M, Kang K, and Venkatesh VG. 2020. Blockchain-Based life cycle assessment: an implementation framework and system architecture. Resources, Conservation and Recycling, 152 (September 2019): 104512.
Zhang J, Khan SA, Heckel DG, and Bock R. 2017. Next-Generation insect-resistant plants: RNAi-mediated crop protection. Trends in Biotechnology, 35(9): 871–882.
Zhang W, Ricketts TH, Carney CKJ, and Swinton SM. 2007. Ecosystem services and dis-services to agriculture. Ecological Economics, 64(2): 253–260.
Information & Authors
Information
Published In
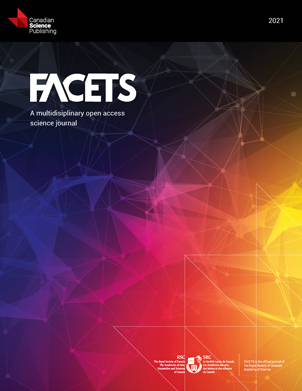
FACETS
Volume 6 • Number 1 • January 2021
Pages: 1955 - 1985
Editor: Elena M. Bennett
History
Received: 19 February 2021
Accepted: 20 August 2021
Version of record online: 2 December 2021
Notes
This paper is part of a Collection titled “Food, Fiber, Fuel, and Function: Pathways to manage ecosystem services in Canada’s working landscapes”.
Copyright
© 2021 Green et al. This work is licensed under a Creative Commons Attribution 4.0 International License (CC BY 4.0), which permits unrestricted use, distribution, and reproduction in any medium, provided the original author(s) and source are credited.
Data Availability Statement
All relevant data are within the paper.
Key Words
Sections
Subjects
Authors
Author Contributions
AGG, KBK, and EDGF conceived and designed the study.
AGG performed the experiments/collected the data.
AGG analyzed and interpreted the data.
EDGF contributed resources.
All drafted or revised the manuscript.
Competing Interests
Evan Fraser is a guest editor of the Food, Fiber, Fuel, and Function collection.
Metrics & Citations
Metrics
Other Metrics
Citations
Cite As
Arthur G. Green, Abdul-Rahim Abdulai, Emily Duncan, Alesandros Glaros, Malcolm Campbell, Rob Newell, Philip Quarshie, Krishna Bahadur KC, Lenore Newman, Eric Nost, and Evan D. G. Fraser. 2021. A scoping review of the digital agricultural revolution and ecosystem services: implications for Canadian policy and research agendas. FACETS.
6(): 1955-1985. https://doi.org/10.1139/facets-2021-0017
Export Citations
If you have the appropriate software installed, you can download article citation data to the citation manager of your choice. Simply select your manager software from the list below and click Download.
Cited by
1. Use of digital technology for research data and information transfer within the Australian grains sector: A case study using Online Farm Trials
2. Himalayan ecosystem services and climate change driven agricultural frontiers: a scoping review
3. Digital revolution for the agroecological transition of food systems: A responsible research and innovation perspective
4. Survey on the Applications of Blockchain in Agriculture
5. Cacao agroforestry systems beyond the stigmas: Biotic and abiotic stress incidence impact
6. Managing Canada’s land- and seascapes for multiple ecosystem services in the Anthropocene: introduction to the Food, Fiber, Fuel, and Function collection