Canadian private protected areas are located in regions of higher vertebrate species richness than government protected areas
Abstract
Nongovernmental organizations contribute to the securement and management of protected areas, but it is not well known how their lands compare to government protected areas or the effectiveness of different land acquisition strategies. Using data from the International Union for Conservation of Nature and BirdLife International, we estimated total and at-risk terrestrial native vertebrate species richness in southern Canada among (i) private protected areas secured by the Nature Conservancy of Canada (NCC), government protected areas, and randomly sampled land; (ii) conservation agreements and fee simple (directly acquired) NCC properties; and (iii) purchased or donated fee simple properties. Controlling for property size and ecoregion, NCC protected areas were predicted to be in areas with 6% and 13% more total and at-risk species than randomly sampled land and 4% and 6% more total and at-risk species than government protected areas. Within NCC protected areas, conservation agreements were predicted to be in areas with 2% and 4% more total and at-risk species than fee simple properties, but purchased properties had similar numbers of total and at-risk species as donated properties. Although we caution that diversity estimates were based on course-grained range maps, our findings suggest that private protected areas are important in conserving biodiversity.
Introduction
The development and exploitation of land by humans, now estimated to be 50% of the Earth’s terrestrial surface (Hooke et al. 2012), is widely recognized as the leading cause of worldwide declines in biodiversity (Maxwell et al. 2016; Johnson et al. 2017; Sanchez-Bayo and Wyckhuys 2019). One solution to mitigate these declines is the creation of protected areas. The International Union for Conservation of Nature (IUCN) defines protected areas as “a clearly defined geographical space, recognised, dedicated and managed, through legal or other effective means, to achieve the long-term conservation of nature with associated ecosystem services and cultural values” (Dudley 2008). In 1992, the United Nations set a worldwide goal of having 17% of all terrestrial land protected by 2020 (United Nations Convention on Biological Diversity 1992). In Canada, approximately 12.1% of terrestrial land and 13.8% of marine environments are currently covered by protected areas (Environment and Climate Change Canada 2019a). As of 2015, over 95% of these protected areas were under government jurisdiction and governance (Environment and Climate Change Canada 2016). However, the effectiveness of government protected areas for conserving biodiversity has been debated. One study found that, in 9 of 15 ecozones, randomly generated plots were over 4 times as likely to have more endangered species than government protected areas (Deguise and Kerr 2006). Conversely, a recent meta-analysis found that government-run marine protected areas had higher fish biomass compared with unprotected waters (Ban et al. 2014). Similarly, evidence suggests that government protected areas in Canada have higher species richness and species at risk richness than randomly selected parcels of similar-size land (Schuster et al. 2019).
Nongovernment protected areas could also play an important role in protecting biodiversity. A recent study provided evidence that Indigenous-managed lands in Australia, Brazil, and Canada contained higher species richness than both IUCN categories I–VI protected areas and a random sample of land (Schuster et al. 2019). Conservation agreements, which are legal agreements that limit the use of land for the purpose of conservation, are one method by which protected areas can be secured by nongovernmental organizations. In the United States, conservation agreements had a lower human modification index than a random sample of land in the same region (Fouch et al. 2019). Additionally, while conservation agreements in the United States Rocky Mountains represented under 5% of total land area, they increased the protected area coverage of 10% of ecosystems within the region (Graves et al. 2019). There is less information, however, on the contribution of private protected areas to biodiversity conservation outside the United States, despite there being many active nongovernmental organizations that secure and manage protected areas around the world.
In this study, we examined estimates of the number of native terrestrial vertebrate species (amphibians, birds, mammals, and reptiles) and species at risk (SAR) in protected areas created by Canadian governments and the Nature Conservancy of Canada (NCC) located in the southern half of Canada (Fig. 1). NCC is a not-for-profit organization founded in 1962 with the goal of “creating a legacy for future generations by conserving important natural areas and biological diversity across all regions of Canada” (Nature Conservancy of Canada 2020c). Over the course of its existence, NCC has contributed to the conservation of over 14 million ha of habitat (Nature Conservancy of Canada 2019c), has directly protected over 2 million ha of land (includes fee simple ownership, conservation agreements, land transferred to partners and resource rights directly secured by NCC), and currently has over 348 470 ha of land under their direct management (fee simple and conservation agreements). For over a decade, NCC has used a quantitative prioritization procedure to acquire protected areas (Arnold and Kraus 2009). While this procedure is not based on standardized species diversity data collected over a broad geographic area, their prioritization does attempt to target areas of high biodiversity for land securement. Thus, we hypothesized that NCC protected areas in Canada would have higher biodiversity estimates than randomly sampled pieces of land, but similar biodiversity compared with government protected areas. Following this hypothesis, we predicted that total native vertebrate species richness and SAR richness on NCC protected areas would be higher than or equal to that of government protected areas and would be higher than a random sample of land.
Fig. 1.

We also evaluated species diversity based on NCC’s methods of securement. Generally, NCC secures land through either conservation agreements (also known as conservation easements, covenants, or servitudes depending on provincial jurisdiction) or direct acquisition (known as “fee simple”). Conservation agreements are legal agreements that exist between a private landowner and NCC that permanently limits, but does not entirely prevent, land-use activities that are incompatible with the protection of targeted conservation values. In contrast, fee simple properties are secured and managed solely by NCC (Nature Conservancy of Canada 2019a). We hypothesized that fee simple properties would have both higher total biodiversity and SAR diversity than conservation agreements in part because fee simple ownership does not limit the scope of the species or habitats being targeted for conservation and also because there is a much lower overall degree of residential and commercial activity on fee simple properties. Following this hypothesis, we predicted more total and SAR species would be expected to be present on fee simple properties than on conservation agreements properties. Finally, fee simple properties can either be secured via direct purchase or donation. We hypothesized that properties directly purchased would be in regions that have higher total and SAR biodiversity than properties received through donations because NCC would more likely invest limited financial resources in purchasing fee simple properties that are identified of greater priority through their rigorous planning and prioritization procedure. In contrast, while potential properties offered through donation are still subjected to the same evaluation process, there is still an opportunistic element to their acquisition. Following this hypothesis, we predicted that there will be higher total and SAR species diversity on purchased protected areas than on donated protected areas.
Methods
Data collection and management
Shapefiles of NCC properties were obtained from the NCC geospatial database and classified into two groups: conservation agreement and fee simple. Fee simple properties were further divided by acquisition method (purchased, donated, other). Shapefiles of government protected areas were obtained from the Canadian Protected and Conserved Areas Database (Government of Canada 2019; https://www.canada.ca/en/environment-climate-change/services/national-wildlife-areas/protected-conserved-areas-database.html). Government protected areas were subset to include only provincially and federally run protected areas. Range maps for birds were obtained from BirdLife International (2018, datazone.birdlife.org/species/requestdis) and range maps for reptiles, amphibians, and mammals were obtained from the IUCN (2020, iucnredlist.org/resources/spatial-data-download). Amphibian, avian, reptile, and mammal species were classified as SAR if they were designated as Special Concern, Threatened, Endangered, Extirpated, or Extinct under Schedule I of Canada’s Species at Risk Act (SARA), candidate species for SARA by the Committee on the Status of Endangered Wildlife in Canada (COSEWIC), and (or) critically endangered, endangered, or vulnerable by the IUCN. Ecoregion data were obtained from Environment and Climate Change Canada Canadian terrestrial ecological framework (Environment and Climate Change Canada 2019b).
All protected areas were subset to only those located in the southerly half of Canada, including some of the southern boreal region (Fig. 1; Bradstreet et al. 2018), which is where NCC focuses most of its land acquisition and management efforts. The IUCN and BirdLife International range maps were also subset to only include species found within this boundary (n = 597). To control for potential spatial biases in biodiversity, we also assigned all NCC and government protected areas to an ecoregion, which is an ecologically distinct region defined by Environment Canada (Statistics Canada 2018), and included as a random effect in all statistical models examining variation in species richness (see model details below). In cases where protected areas crossed over the boundary of two ecoregions (n = 716 NCC parcels, n = 606 government parcels), one of the two ecoregions was randomly selected for the species richness model analysis.
For both NCC and government lands, some protected areas with the same name were composed of multiple land parcels with each parcel being represented as a separate polygon with a unique ID in the GIS database. For NCC, there was a total of 5301 land parcels representing 1321 fee simple protected areas and 637 conservation agreements, and for government lands, there was a total of 6684 land parcels representing 6510 protected areas. For the purposes of the species richness models (details below), we treated land parcels individually but for the purposes of comparing size between NCC protected areas and government protected areas and within different types of NCC protected areas, we used the amalgamated parcels with the same protected area name. Using the National Oceanic and Atmospheric Administration Biogeography Branch’s sampling design tool (NOAA Biogeography Branch 2016), we also generated a random sample of 13 222 points. A minimum sample of 20 points per ecoregion was used to allow an adequate sample size for analysis. A circular buffer was then created around each point using a randomly selected area from an already existing protected area (Schuster et al. 2019).
Overlay analysis
To estimate the total number of species and number of SAR distributed on protected area and randomly generated areas, we then conducted an overlay analysis. First each of the range maps, protected areas, and random areas were separated into individual shapefiles using a python script (Van Rossum and Drake 1995; Patterson 2016) that divides a shapefile with multiple polygons into a shape file of polygons with the same attributes (Schuster et al. 2019). For example, a shapefile with all bird species was split to create a singular polygon for each bird species. Each IUCN and BirdLife International range map was then intersected with a grid to reduce script run time (Schuster et al. 2019). An overlay analysis was then run to count the number of species in each of the three land classifications (Schuster et al. 2019). Using a second python script, each land parcel was clipped with the range map for each species. If this clip produced an existing shapefile then the species was considered present on the protected area and the total number of species in each land parcel was calculated. After looking at Pearson residual versus fitted value plots, land parcels under 50 m2 or over 36 303 km2 (n = 30) were removed from the data set to meet assumptions of the statistical models. For the data set used to examine overall species richness, any parcels that contained zero species (n = 47) were removed from the analysis because we deemed this as an unlikely result. However, for the data set used to examine species at risk richness, because there were fewer species overall, parcels with zero SAR were kept in the analysis. Python script for the overlay analysis is presented in Supplementary Material 1.
Statistics
We used the package lme4 (Bates et al. 2015) in R statistical computing package, v 3.5.3 (R Core Team 2019) to perform linear mixed effect models to predict the variation in total species and SAR richness. In the first set of models, we included protected area type (factor levels: NCC protected areas, government protected areas, a random sample of land), size (ha), and the interaction between protected area type and size as fixed effects, and ecoregion as a random intercept. In the second set of models comparing NCC properties, protected area type (factor levels: conservation agreement or fee simple), size (ha), and an interaction between the two predictor variables were included as fixed effects and ecoregion was included as a random intercept. In the third set of models, we included acquisition method (factor levels: donated or purchased), area (ha), and an interaction between acquisition method and size (ha) as fixed effects and ecoregion as a random intercept. Starting with the full models stated above, model selection for total species and SAR models was performed separately by comparing AIC scores of all possible model combinations using the R package MuMIn (Barton 2019), considering all models within ΔAIC ≤ 2 of the top model to have equal support. We computed 95% confidence intervals of parameters using the Wald method to assess the relative importance of each fixed effect in the top-ranked models. Both the response and numerical predictor variables were z-scaled for analyses because this helped reduced the influence of extreme values and meet the assumptions of the model. Values were then back-transformed for plotting. Means are reported with ± SD.
Results
NCC protected areas ( = 187 ha, SD = 1347, range = 0.034–54 612, n = 1958) were, on average, an order of magnitude smaller than government protected areas ( = 4988, SD = 39 064 ha, range = 0.01–1 378 067, n = 6267). Within NCC protected areas, conservation agreement properties ( = 214 ha, SD = 642, range = 0.038–12 306, n = 637) were similar in size compared with fee simple properties ( = 174 ha, SD = 1579, range = 0.034–54 612, n = 1321) and, within fee simple properties, donated properties ( = 108 ha, SD = 547, range = 0.034–8964, n = 325) were similar in size to purchased properties ( = 142 ha, SD = 497, range = 0.079–8735, n = 925).
Comparing species diversity between NCC protected areas, government protected areas, and the random sample of land, our best supported model included protected area type and size as fixed effects and ecoregion as a random effect (ΔAIC of next best model =10.71; Table 1). Government protected areas were predicted to be located in areas with 4% (i.e., 7 species; Fig. 2A) fewer species than NCC protected areas of the same size (coefficient = −0.179, 95% CI = −0.207, −0.150; Table 1). The random sample had the lowest species diversity compared with both protected area types, with 6% (i.e., 11 species; Fig. 2A) fewer species than areas where NCC properties were located, when controlling for size and ecoregion (coefficient = −0.277, 95% CI = −0.308, −0.246; Table 1). Models examining SAR richness yielded similar results (Fig. 2B; Table S1).
Table 1.
Model selection | Parameter estimates (∼ protected area type + size) | |||||
---|---|---|---|---|---|---|
Model | K | AIC | ΔAIC | Fixed effects | Coefficient | 95% CI |
Protected area type + size | 6 | 43 835.0 | 0.00 | Intercept | −0.123 | (−0.326, 0.079) |
Protected area type × size | 8 | 43 845.7 | 10.71 | Protected area type: government | −0.179 | (−0.207, −0.150) |
Protected area type | 5 | 43 892.7 | 57.67 | Land management: random | −0.277 | (−0.308, −0.246) |
Size | 4 | 44 147.3 | 312.22 | Size | 0.032 | (0.024, 0.039) |
Intercept only | 3 | 44 198.3 | 363.28 | Random intercept | σ2 | |
Ecoregion | 1.418 |
Note
Model selection (left) was based on AIC. For each model, K (number of parameters), AIC (Akaike Information Criterion), and ΔAIC (the difference in AIC compared to the top model) are shown. The parameter estimates for the top model are shown on the right.
Fig. 2.

For total species richness within NCC properties, the best-supported model included NCC protected area type (conservation agreement, fee simple) as a fixed effect and ecoregion as a random effect (ΔAIC of next best model = 8.69; Table 2, Fig. 2C). However, fee simple properties were predicted to be in areas that have only 2% (i.e., 4 species) lower species diversity than conservation agreements while controlling for ecoregion (coefficient for fee simple protected areas = −0.115, 95% CI = −0.171, −0.058; Fig. 2C, Table 2). Within NCC fee simple properties, the best supported models included NCC property type (donated, purchased) but the next best supported model was an intercept only model with a ΔAIC of 1.46 (Table 3, Fig. 2E). Models examining SAR richness yielded similar results for both protected area type and whether the property was donated or purchased (Figs. 2D and 2F; Tables S2 and S3).
Table 2.
Model selection | Parameter estimates (∼ protected area type) | |||||
---|---|---|---|---|---|---|
Model | K | AIC | ΔAIC | Fixed effects | Coefficient | 95% CI |
NCC protected area type | 4 | 12 748.7 | 0.00 | Intercept | −0.397 | (−0.081, −0.712) |
NCC protected area type + size | 5 | 12 757.3 | 8.69 | NCC protected area type: Fee simple | −0.115 | (−0.058, −0.171) |
NCC protected area type × size | 6 | 12 757.4 | 8.73 | Random intercept | σ2 | |
Intercept only | 3 | 12 762.4 | 13.73 | Ecoregion | 1.386 | |
Size | 4 | 12 766.1 | 17.45 |
Note
Model selection (left) was based on AIC. For each model, K (number of parameters), AIC (Akaike Information Criterion), and ΔAIC (the difference in AIC compared to the top model) are shown. The parameter estimates for the top model are shown on the right.
Table 3.
Model selection | ||||
---|---|---|---|---|
Model | K | AIC | ΔAIC | wi |
Property type | 4 | 7478.7 | 0.00 | 0.638 |
Intercept only | 3 | 7480.2 | 1.46 | 0.307 |
Property type + size | 4 | 7484.5 | 5.80 | 0.035 |
Size | 5 | 7486.0 | 7.31 | 0.017 |
Property type × size | 6 | 7489.47 | 10.72 | 0.003 |
Note
For each model, K (number of parameters), AIC (Akaike Information Criterion), ΔAIC (the difference in AIC compared to the top model), and wi (model weight) are shown. The intercept model had similar support to the top ranked model, so no parameter estimates are presented.
Discussion
Our study provides evidence that, while controlling for ecoregion and size, NCC protected areas tend to be located in areas of higher species richness when compared with government protected areas and that both of these protected area types are located in areas of higher species richness and SAR species richness than randomly sampled land. We also provide evidence that conservation agreement properties have a higher total species and SAR richness compared with properties that are solely managed by NCC (i.e., fee simple) and that donated fee simple properties have similar diversity compared with directly acquired fee simple properties. Overall, our work provides some evidence for the notion that nongovernmental agencies make significant contributions to the protection of biodiversity at a national level.
Although we used IUCN range maps to estimate vertebrate species richness, several studies have shown these maps can overestimate ranges or lead to misclassification on the presence/absence of species (Di Marco et al. 2017; Ramesh et al. 2017). Specifically, range maps have been shown to overestimate species at a spatial resolution of under 200 km (Hurlbert and Jetz 2007). This is important to note given the average size of NCC protected areas (187 ha) is smaller than this resolution, meaning there may be an over estimation of species diversity in these protected areas. While we considered the use of these alternative data sets, such as citizen science data or range maps generated by the Committee on the Status of Endangered Wildlife in Canada, we found that they were less complete than IUCN range maps (Di Marco et al. 2017), had issues with accuracy at small spatial resolution, and (or) had sampling bias (Hurlbert and Jetz 2007; Perkins 2020) and were, therefore, not suitable for our analyses. Although local data on species diversity is available for some protected areas, there are no standardized data available for NCC or government protected areas making these local data unsuitable for our broad analysis. Therefore, at this time, the IUCN maps, with over 75 000 animals (IUCN 2020), appear to represent the most complete data source available (Di Marco et al. 2017). Use of this data set also provides the advantage of conducting standardized comparisons with other areas of the world. That said, potential inaccuracies within the IUCN data set suggest that our results should be considered with some caution. Another, perhaps equally cautionary note, is that we have not included invertebrate diversity in this assessment because range maps or abundance data for this hyper-diverse taxon are nonexistent.
One explanation why NCC protected areas would have been created in regions with higher species richness than government protected areas could be related to the motivation behind their creation or acquisition. Historically, federally run protected areas in Canada have been created with multiple goals, one of which is to conserve biodiversity, but these could also include conserving locations of cultural importance as well for public recreation and education (Dearden and Dempsey 2004). Although the National Parks Act was created in 1930, it was not until 1969 that policies on the creation of national parks were first updated to prioritize ecological needs and not until 1988 that this was made law (Dearden and Dempsey 2004). This is in contrast with NCC, whose sole goal since their 1962 inception has been focused on the protection of biodiversity (Nature Conservancy of Canada 2019b).
Of the 15 federal and provincial government organizations responsible for planning and securing protected areas in Canada, 13 have created a systematic framework for creating and managing protected areas; however, as of 2016, these strategies have only been partially implemented (Environment and Climate Change Canada 2016). Because the creation of government protected areas can take years (Hume 2008), it is possible that the outcomes of these strategies will not be seen for a number of years ahead. Even in cases where protected areas have been created by government organizations, the priority behind their creation is not always to maximize the number of species protected. For example, a top priority of 80% of government organizations is representation of all ecological regions (Environment and Climate Change Canada 2016) rather than optimizing species richness across a jurisdiction. In contrast, NCC has been implementing formal conservation plans since the late 1990s (Arnold and Kraus 2009) and prioritizes nationally and provincially significant land, habitats for at risk species, and land that increases connectivity between protected areas (Nature Conservancy of Canada 2020a).
Another plausible explanation for NCC protected areas being located where species richness is higher than government protected areas is that nongovernmental organizations typically have fewer stakeholders to consult and requirements to fulfill during the land acquisition process. Since 1988, public participation has been included in the creation and modification procedures of federal protected areas (Dearden and Dempsey 2004). While often valuable, public participation can also delay, modify, or even halt the creation of protected areas (Bendickson 2009). In addition, federal protected areas need to be approved by the House of Commons and Senate (Bendickson 2009). In contrast, although NCC always engages in discussions with neighbouring landowners, they are not legally required to get external input when creating a protected area (Nature Conservancy of Canada 2020a). NCC does follow external guidelines as part of the Natural Areas Conservation Program, which is a federally funded program to aid nongovernmental organizations in establishing protected areas (Environment and Climate Change Canada 2018). Under this program, organizations are audited every five years to measure their effectiveness at achieving their program goals and progress that has been made (Nature Conservancy of Canada 2020a). Thus, nongovernmental organizations have the potential to take advantage of opportunities quicker than a government agency. For example, when land became available next to the Waterton Lakes National Park, NCC was able to act quickly, partly because they did not require public discussion or approval from Parliament (Nature Conservancy of Canada 2020d).
Interestingly, our results did not provide support for the hypothesis that fee simple properties being in areas with higher vertebrate species richness than conservation agreement properties. In contrast, the top model predicted an average of 4 additional species and 0.4 additional species at risk on conservation agreement properties compared to fee simple properties. One possible explanation for this result is that conservation agreements are, in theory, able to protect diverse habitat types specifically targeted as a result of the quantitative land prioritization procedure but that can be difficult to directly acquire, either because there is little to no available habitat to purchase, such as ranch lands (Bendickson 2009), or the cost of acquisition is high, such as habitats near urban developments (Morris 2011). Whatever the reason, we view this result as positive news for many land trust organizations because conservation agreements are an effective way to designate conservation land and can make up a significant portion of a protected area portfolio. At NCC, for example, conservation agreements represent 40% of protected areas.
Our model comparing types of fee simple properties provided no support for the hypothesis predicting that estimated vertebrate species richness would be higher on directly purchased property compared with donated property. The reason why we proposed this hypothesis was because there are more opportunities to be selective about purchasing specific properties, whether it be through individuals or organizations approaching NCC to sell their property or through standard real estate channels. In contrast, NCC may accept land donations that are of lesser conservation importance because the conservation benefit relative to the financial cost remains extremely high. However, NCC receives many more requests for land donations than they are capable of accepting. In this way, there is, to some extent, a selection process such that properties that are offered through donations still go through a prioritization procedure (Nature Conservancy of Canada 2020b). Therefore, donated fee simple properties form a very important component of land holdings for NCC and likely for other nongovernmental land trusts.
Although we used broad patterns of species richness as our metric of “effectiveness”, there are a number of other potentially useful approaches. One possibility is an estimate of “human development” using a combination of distance to population centres, proximity to roads, and accessibility of the protected area to humans (Fouch et al. 2019). The degree of connectivity between protected areas has also been used to assess effectiveness of protected areas (Bargelt et al. 2020), although this relies on the assumption that higher connectivity enhances dispersal and promotes long-term population persistence for all species that are included in the analysis. There are also other biological indicators that may be informative. For example, abundance has been used to assess protected areas where the data are available (e.g., African mammals; Craigie et al. 2010). Abundance can be used to examine changes over time, which provides the potential to use it in a before-and-after design (Craigie et al. 2010). Another potential biological indicator which has been used to assess biodiversity is beta diversity (Bergamin et al. 2017). Challenges that arise with these measures, however, revolve around data availability and accuracy, particularly when analyzing effectiveness of protected areas at a national scale.
Our results highlight the important role of private protected areas for land conservation. We also show that conservation agreements and donated fee simple properties are key tools for the success of nongovernmental organizations. While our work emphasizes the positive contributions of a nongovernmental organization, we note that our results are based on broad patterns of species diversity based on range maps and do not depict the actual number of species located in each study location. However, our general findings do suggest that it will be important that multiple governance bodies, including Indigenous groups (Schuster et al. 2019), continue to establish and manage protected areas for effectively conserving biodiversity. It will also be important for organizations that create protected areas to consider how they identify areas of high biodiversity, especially with the limitations of current large-scale biodiversity data sets (Hurlbert and Jetz 2007). While nongovernment organizations may have more flexibility for targeting specific areas or habitats, governments have the ability to secure much larger protected areas and all possible approaches will be needed to stem global biodiversity loss.
Acknowledgements
We thank Michael Bradstreet for his valuable insight and for assistance with data collection and analysis. Claire Kremen and Angela Brennan provided valuable guidance during all stages of the project. This work was supported by The W. Garfield Weston Foundation. We also thank the editor and two anonymous reviewers whose comments improved the manuscript.
References
Arnold H, and Kraus D. 2009. Conservation assessment, planning & implementation at multiple scales. In CCEA Marine Protected Areas Conference, Ottawa, Ontario.
Ban N, Beck M, Salomon A, and Cripps K. 2014. Applying empirical estimates of marine protected area effectiveness to assess conservation plans in British Columbia, Canada. Biological Conservation, 180: 134–148.
Bargelt L, Fortin M-J, and Murray DL. 2020. Assessing connectivity and the contribution of private lands to protected area networks in the United States. PLoS ONE, 15(3): e0228946.
Barton K. 2019. MuMIn: multi-model inference. V 2.43.15 [online]: Available from CRAN.R-project.org/package=MuMIn.
Bates D, Maechler M, Bolker B, and Walker S. 2015. Fitting linear mixed effects models using lme4. Journal of Statistical Software, 67(1): 1–48.
Bendickson J. 2009. Legal framework for protected areas. International Union for the Conservation of Nature, Gland, Switzerland [online]: Available from iucn.org/downloads/canada.pdf.
Bergamin R, Bastazini V, Velez-Martin E, Debastiani V, Zanini K, Loyola R, et al. 2017. Linking beta diversity patterns to protected areas: lessons from the Brazilian Atlantic Rainforest. Biodiversity and Conservation, 26: 1557–1568.
BirdLife International. 2018. Bird species distribution maps of the world. Handbook of the Birds of the World. Version 2018.1 [online]: Available from datazone.birdlife.org/species/requestdis.
Bradstreet M, Hebb A, and Kraus D. 2018. Conservation assessment for Southern Canada. Nature Conservancy of Canada, Toronto, Ontario.
Craigie I, Baillie J, Balmford A, Carbone C, Collen B, Green R, et al. 2010. Large mammal population declines in Africa’s protected areas. Biological Conservation, 143(9): 2221–2228.
Dearden P, and Dempsey J. 2004. Protected areas in Canada: decade of change. Canadian Geographer, 48: 225–239.
Deguise I, and Kerr J. 2006. Protected areas and prospects for endangered species conservation in Canada. Conservation Biology, 20(1): 48–55.
Di Marco M, Watson J, Possingham H, and Venter O. 2017. Limitations and trade-offs in the use of species distribution maps for protected area planning. Journal of Applied Ecology, 54: 402–411.
Dudley N (Editor). 2008. Guidelines for applying protected area management categories. International Union for the Conservation of Nature, Gland, Switzerland.
Environment and Climate Change Canada. 2016. Canadian protected areas status report 2012–2015. Environment and Climate Change Canada, Gatineau, Quebec [online]: Available from publications.gc.ca/collections/collection_2016/eccc/En81-9-2016-eng.pdf.
Environment and Climate Change Canada. 2018. Evaluation of the habitat conservation partnerships program: Appendix A. Environment and Climate Change Canada, Gatineau, Quebec [online]: Available from canada.ca/en/environment-climate-change/corporate/transparency/priorities-management/evaluations/evaluation-habitat-conservation-partnerships-program/appendix-a.html.
Environment and Climate Change Canada. 2019a. Canadian environmental sustainability indicators: Canada’s conserved areas. Environment and Climate Change Canada, Gatineau, Quebec [online]: Available from canada.ca/en/environment-climate-change/services/environmentalindicators/conserved-areas.html.
Environment and Climate Change Canada. 2019b. Canadian terrestrial ecological framework. Environment and Climate Change Canada: Monitoring Assessment and Information Management Division, Gatineau, Quebec.
Fouch F, Baldwin R, Gerard P, Dyckman C, and Theobald D. 2019. Landscape-level naturalness of conservation easements. Landscape Ecology, 34: 1967–1987.
Global Administrative Areas Database. 2020. Map of Canada [online]: Available from gadm.org/index.html.
Government of Canada. 2019. Canadian Protected and Conserved Areas Database. Environment and Climate Change Canada, Gatineau, Quebec [online]: Available from canada.ca/en/environment-climate-change/services/national-wildlife-areas/protected-conserved-areas-database.html.
Graves R, Williamson M, Belote R, and Jodi S. 2019. Quantifying the contribution of conservation easements to large-landscape conservation. Biological Conservation, 232: 83–96.
Hooke R, Martin-Duque J, and Pedraza J. 2012. Land transformation by humans: a review. Geological Society of America Today, 22(12): 4–10.
Hume M. 2008. An ‘oasis’ of 20,000 intrigues under the sea. Global and Mail.
Hurlbert AH, and Jetz W. 2007. Species richness, hotspots, and the scale dependence of range maps in ecology and conservation. Proceedings of the National Academy of Sciences of the United States of America, 104(33): 13384–13389.
International Union for the Conservation of Nature (IUCN). 2020. The IUCN Red List of threatened species. Version 2019-3 [online]: Available from iucnredlist.org.
Johnson C, Balmfor A, Brook B, Buettel J, Galetti M, Gaungchun L, et al. 2017. Biodiversity losses and conservation responses in the anropocene. Science, 356: 270–275.
Maxwell SL, Fuller R, Brooks T, and Watson J. 2016. The ravages of guns nets and bulldozers. Nature, 536: 143–145.
Morris A. 2011. Conservation easements and urban parks: from private to public use. Natural Resources Journal, 51: 357–384.
Nature Conservancy of Canada. 2019a. Conservation agreement. Nature Conservancy of Canada [online]: Available from natureconservancy.ca/en/what-we-do/resource-centre/glossary/conservation_agreement.html.
Nature Conservancy of Canada. 2019b. Who we are. Nature Conservancy of Canada [online]: Available from natureconservancy.ca/en/who-we-are/.
Nature Conservancy of Canada. 2019c. What we do. Nature Conservancy of Canada [online]: Available from natureconservancy.ca/en/what-we-do/.
Nature Conservancy of Canada. 2020a. Frequently asked questions. Nature Conservancy of Canada [online]: Available from natureconservancy.ca/en/what-we-do/government-of-canada-partnership/conservation-program/frequently-asked-questions.html.
Nature Conservancy of Canada. 2020b. Gifts of land. Nature Conservancy of Canada [online]: Available from natureconservancy.ca/en/what-you-can-do/other-ways-to-help/give-land/.
Nature Conservancy of Canada. 2020c. Mission and values. Nature Conservancy of Canada [online]: Available from natureconservancy.ca/en/who-we-are/mission-values/.
Nature Conservancy of Canada. 2020d. Waterton Park Front Project. Nature Conservancy of Canada [online]: Available from natureconservancy.ca/en/where-we-work/alberta/featured-projects/waterton-park-front-project.html.
NOAA Biogeography Branch. 2016. Sampling design tool [online]: Available from arcgis.com/home/item.html?id=28f08ca526ae44e8ac107a2a0d5f50e3.
Patterson D. 2016. Split layer by attributes [online]: Available from arcgis.com/home/item.html?id=15ca63aebb4647a4b07bc94f3d051da5.
Perkins D. 2020. Blind spots in citizen science data: implications of volunteer bias in eBird data. Master’s thesis, North Carolina State University, Raleigh, North Carolina.
R Core Team. 2019. R: a language and environment for statistical computing. R Foundation for Statistical Computing, Vienna, Austria [online]: Available from R-project.org.
Ramesh V, Gopalakrishna T, Barve S, and Melnick D. 2017. IUCN greatly underestimates threat levels of endemic birds in the Western Ghats. Biological Conservation, 210: 205–221.
Sanchez-Bayo F, and Wyckhuys K. 2019. Worldwide decline of the entomofauna: a review of its drivers. Biological Conservation, 232: 8–27.
Schuster R, Germain R, Bennett J, Reo N, and Arcese P. 2019. Vertebrate biodiversity on indigenous-managed lands in Australia, Brazil and Canada equals that in protected areas. Environmental Science and Policy, 101: 1–6.
Statistics Canada. 2018. Introduction to the ecological land classification (ELC). Statistics Canada [online]: Available from statcan.gc.ca/eng/subjects/standard/environment/elc/2017-1.
United Nations Convention on Biological Diversity. 1992. TARGET 11—technical rationale extended (provided in document COP/10/INF/12/Rev.1). United Nations Convention on Biological Diversity, New York City, New York [online]: Available from cbd.int/sp/targets/rationale/target-11/.
Van Rossum G, and Drake Jr, FL. 1995. Python reference manual. Centrum voor Wiskunde en Informatica Amsterdam.
Supplementary material
Supplementary Material 1 (DOCX / 21 KB)
- Download
- 20.36 KB
Information & Authors
Information
Published In
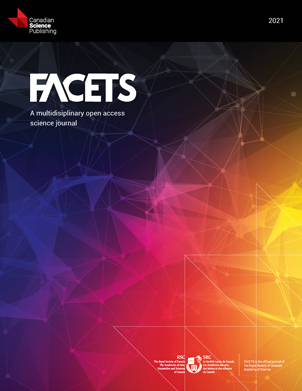
FACETS
Volume 6 • Number 1 • January 2021
Pages: 1323 - 1336
Editor: Fanie Pelletier
History
Received: 7 December 2020
Accepted: 15 April 2021
Version of record online: 12 August 2021
Copyright
© 2021 Custode et al. This work is licensed under a Creative Commons Attribution 4.0 International License (CC BY 4.0), which permits unrestricted use, distribution, and reproduction in any medium, provided the original author(s) and source are credited.
Data Availability Statement
All relevant data are within the paper and in the Supplementary Material.
Key Words
Sections
Subjects
Authors
Author Contributions
LBC and DRN conceived and designed the study.
All performed the experiments/collected the data.
LBC, MMG, NB, CE, MP, and DRN analyzed and interpreted the data.
SMK, M-MR-C, and DRN contributed resources.
All drafted or revised the manuscript.
Competing Interests
Two of the authors on this paper (Samantha Knight, Marie-Michele Rouseau-Clair) are employees of the Nature Conservancy of Canada (NCC). A third author (Ryan Norris), while faculty at the University of Guelph, is part of a formal partnership between the University of Guelph and NCC and receives a portion of his salary from NCC. All data used for this paper and the code used to analyze these data will be made publicly available.
Metrics & Citations
Metrics
Other Metrics
Citations
Cite As
Leonardo B. Custode, Matthew M. Guzzo, Natasha Bush, Claire Ewing, Michael Procko, Samantha M. Knight, Marie-Michele Rousseau-Clair, and D. Ryan Norris. 2021. Canadian private protected areas are located in regions of higher vertebrate species richness than government protected areas. FACETS.
6: 1323-1336.
https://doi.org/10.1139/facets-2020-0108
Export Citations
If you have the appropriate software installed, you can download article citation data to the citation manager of your choice. Simply select your manager software from the list below and click Download.
Cited by
1.
2. A Canada-wide macro assessment of protected area connectivity