Association of CLOCK gene variants with obesity and adiposity-related anthropometric, metabolic, and behavioral parameters
Abstract
Introduction
Material and methods
Study population
Blood sample collection
Anthropometric parameters
Metabolic parameters
Behavioral parameters
DNA isolation and genotyping
Statistical analysis
Power calculation
Results
Genotypic frequencies of the CLOCK variants and HWE
Unadjusted | Adjusted for age and gender | |||||||||
---|---|---|---|---|---|---|---|---|---|---|
Genetic Model | Genotype/alleles | Controls | Cases | OR | 95% CI | p | OR | 95% CI | p | h- index |
rs4864548 | ||||||||||
Additive | G vs A | G = 347 (43%) | G = 337 (55%) | 1.071 | 0.851–1.348 | 0.558 | 1.090 | 0.864–1.375 | 0.466 | — |
A = 265 (57%) | A = 275 (45%) | |||||||||
Pairwise Comparison | ||||||||||
Wild type homozygous vs. mutant homozygous | GG vs. AA | — | — | 1.029 | 0.642–1.648 | 0.906 | 1.063 | 0.660–1.712 | 0.803 | 3.3 |
Wild type homozygous vs. heterozygous | GG vs. GA | — | — | 1.656 | 1.148–2.388 | 0.007 | 1.648 | 1.142–2.379 | 0.008 | 3.3 |
Dominant | AA + GA | 200 (47.1%) | 225 (52.9%) | 1.458 | 1.031–2.063 | 0.033 | 1.471 | 1.038–2.084 | 0.030 | 3.3 |
GG | 105 (56.5%) | 81 (43.5%) | ||||||||
Over- dominant | GA | 131 (42.8%) | 175 (57.2%) | 1.638 | 1.190–2.255 | 0.002 | 1.611 | 1.169–2.221 | 0.004 | 3.3 |
GG + AA | 168(55.1%) | 137 (44.9%) | ||||||||
Recessive | AA | 63 (55.8%) | 50 (44.2%) | 0.750 | 0.498–1.131 | 0.170 | 0.750 | 0.512–1.176 | 0.232 | 3.3 |
GG + GA | 242 (48.6%) | 256 (51.4%) | ||||||||
Hardy–Weinberg Equilibrium | ||||||||||
Cases | p = 0.006 | |||||||||
Controls | P = 0.141 | |||||||||
rs6843722 | ||||||||||
Additive | A vs C | A = 373 (61%) | A = 344 (56 %) | 1.230 | 0.972–1.556 | 0.084 | 1.249 | 0.985–1.585 | 0.067 | |
C = 239 (39 %) | C = 268 (44 %) | |||||||||
Pairwise Comparison | ||||||||||
Wild type homozygous vs. mutant homozygous | AA vs. CC | — | — | 1.267 | 0.779–2.062 | 0.341 | 1.311 | 0.801–2.147 | 0.281 | 1.864 |
Wild type homozygous vs. heterozygous | AA vs. AC | 1.805 | 1.262–2.581 | 0.001 | 1.812 | 1.264–2.597 | 0.001 | 1.864 | ||
Dominant | CC + AC | 188 (61.4%) | 222 (72.5%) | 1.659 | 1.180–2.330 | 0.004 | 1.681 | 1.192–2.371 | 0.003 | 1.864 |
AA | 118 (36.8%) | 84 (27.5%) | ||||||||
Over- dominant | CC + AA | 169 (55.2%) | 130 (42.5%) | 1.670 | 1.213–2.299 | 0.002 | 1.657 | 1.203–2.284 | 0.002 | 1.864 |
AC | 137 (44.8%) | 176 (57.5%) | ||||||||
Recessive | AA + AC | 255 (83.3%) | 260 (85%) | 0.885 | 0.573–1.366 | 0.580 | 0.908 | 0.586–1.408 | 0.667 | 1.864 |
CC | 51 (16.7%) | 46 (15.0%) | ||||||||
Hardy–Weinberg Equilibrium | ||||||||||
Cases | p-value = 0.003 | |||||||||
Controls | p-value = 0.199 |
Note: The genotypic/allelic frequencies for CLOCK gene variants, rs6843722 and rs4864548, are shown in counts (percentage in parentheses). The unadjusted and age- and gender-adjusted association of the CLOCKgene variants with overweight/obesity was also determined by logistic regression assuming additive, pair-wise comparison (wild type homozygous vs. mutant homozygous and wild type homozygous vs. heterozygous), dominant, recessive, and over-dominant genetic models. The h-index was computed for determining the appropriate mode of inheritance. The odds ratios along with 95% confidence intervals and p values were calculated to seek the impact and strength of the association, respectively. p < 0.05 was considered statistically significant. Statistically significant p values are shown in bold. OR, odds ratio; CI, confidence interval; h-index, degree of dominance index. G vs A, wild type allele vs mutant allele; GG vs. AA, Wild type homozygous vs. mutant homozygous; GG vs. GA, Wild type homozygous vs. heterozygous; AA + GA, mutant A allele carrier; GG, homozygous wild type genotype carrier; GA, heterozygous genotype carrier; GG + AA, homozygous wild type/mutant genotype carrier; AA, homozygous mutant genotype carrier; GG + GA, wild type G allele carrier; A vs C, wild type allele vs mutant allele; AA vs. CC, wild type homozygous vs. mutant homozygous; AA vs. AC, wild type homozygous vs. heterozygous; CC + AC, mutant C allele carrier; AA, homozygous wild type genotype carrier; AC, heterozygous genotype carrier; CC + AA, homozygous wild type/mutant genotype carrier; CC, homozygous mutant genotype carrier; AA + AC, wild type A allele carrier.
Association of the CLOCK variants rs4864548 and rs6843722 with overweight/obese phenotype
Association of the CLOCK variants rs4864548 and rs6843722 with adiposity-related anthropometric traits
Unadjusted | Adjusted for age and gender | |||||||||
---|---|---|---|---|---|---|---|---|---|---|
Parameters (unit of measurement) | Over-dominant model | Mean | SD | β | 95% CI | p | β | 95% CI | p | p value correcteda |
Weight (kg) | GA | 76.83 | 20.76 | 5.828 | 2.524–9.131 | 0.001 | 5.696 | 2.529–8.863 | <0.001 | <0.001 |
GG + AA | 70.80 | 20.87 | ||||||||
WC (cm) | GA | 99.28 | 18.36 | 5.173 | 2.286–8.059 | <0.001 | 4.847 | 1.995–7.699 | 0.001 | 0.002 |
GG + AA | 94.03 | 17.98 | ||||||||
HC (cm) | GA | 106.70 | 13.57 | 4.232 | 2.012–6.400 | <0.001 | 4.046 | 1.836–6.256 | <0.001 | <0.001 |
GG + AA | 102.30 | 14.40 | ||||||||
WHR | GA | 0.93 | 0.08 | 0.020 | 0.007–0.033 | 0.002 | 0.018 | 0.006–0.031 | 0.004 | 0.005 |
GG + AA | 0.91 | 0.08 | ||||||||
WHtR | GA | 0.80 | 0.33 | 0.117 | 0.065–0.170 | <0.001 | 0.110 | 0.058–0.161 | <0.001 | <0.001 |
GG + AA | 0.67 | 0.33 | ||||||||
Biceps SFT (mm) | GA | 14.97 | 8.93 | 1.606 | 0.231–2.981 | 0.022 | 1.487 | 0.204–2.770 | 0.023 | 0.023 |
GG + AA | 13.32 | 8.37 | ||||||||
Triceps SFT (mm) | GA | 24.73 | 11.93 | 3.249 | 1.408–5.090 | 0.001 | 3.145 | 1.328–4.963 | 0.001 | 0.002 |
GG + AA | 21.42 | 11.23 | ||||||||
Abdominal SFT (mm) | GA | 38.21 | 17.09 | 4.527 | 1.844–7.211 | 0.001 | 4.160 | 1.530–6.789 | 0.002 | 0.003 |
GG + AA | 33.61 | 16.69 | ||||||||
Supra-iliac SFT (mm) | GA | 30.99 | 15.29 | 4.265 | 1.861–6.670 | 0.001 | 4.096 | 1.795–6.397 | 0.001 | 0.002 |
GG + AA | 26.67 | 14.96 | ||||||||
Thigh SFT (mm) | GA | 36.09 | 18.58 | 4.403 | 1.432–7.373 | 0.004 | 4.273 | 1.338–7.208 | 0.004 | 0.005 |
GG + AA | 31.62 | 18.80 | ||||||||
Sub-scapular SFT (mm) | GA | 26.70 | 11.86 | 2.831 | 0.954–4.708 | 0.003 | 2.637 | 0.780–4.494 | 0.005 | 0.005 |
GG + AA | 23.79 | 11.75 | ||||||||
%BF | GA | 28.92 | 9.92 | 2.527 | 0.990–4.060 | 0.001 | 2.321 | 0.814–3.820 | 0.003 | 0.004 |
GG + AA | 26.35 | 9.43 |
Note: Association of the CLOCK gene variant rs4864548 with anthropometric parameters was determined using over-dominant genetic model by linear regression. The β, CI, and p values are provided before and after age and gender adjustment. Benjamini-Hochberg method was used for the correction of multiple comparisons. The effect size (β) along with 95% confidence intervals and p-values were calculated to seek the impact and strength of the association, respectively. p < 0.05 was considered statistically significant. The statistically significant p values are shown in bold. Abbreviations: WC, waist circumference; HC, hip circumference; WHR, waist-to-hip ratio; WHtR, waist-to-height ratio; SFT, skin fold thickness; %BF, body fat percentage; SD, standard deviation; β, effect size; CI, confidence interval; GA, heterozygous genotype carrier; GG + AA, homozygous wild type/mutant genotype carrier.
Unadjusted | Adjusted for age and gender | |||||||||
---|---|---|---|---|---|---|---|---|---|---|
Parameters (unit of measurement) | Over-dominant model | Mean | SD | β | 95% CI | p | β | 95% CI | p | p value correcteda |
Weight (kg) | AC | 76.33 | 20.5 | 4.946 | 1.629–8.262 | 0.004 | 4.553 | 1.372–7.734 | 0.005 | 0.018 |
AA + CC | 71.39 | 21.24 | ||||||||
WC (cm) | AC | 98.42 | 18.01 | 3.514 | 0.611–6.417 | 0.018 | 3.363 | 0.501–6.225 | 0.021 | 0.029 |
AA + CC | 94.91 | 18.55 | ||||||||
HC (cm) | AC | 106.02 | 13.7 | 2.930 | 0.694–5.165 | 0.010 | 2.931 | 0.711–5.151 | 0.010 | 0.024 |
AA + CC | 103.09 | 14.40 | ||||||||
WHR | AC | 0.93 | 0.08 | 0.014 | 0.001–0.027 | 0.030 | 0.014 | 0.001–0.026 | 0.036 | 0.039 |
AA + CC | 0.91 | 0.08 | ||||||||
WHtR | AC | 0.70 | 0.34 | 0.085 | 0.032–0.138 | 0.002 | 0.085 | 0.034–0.137 | 0.001 | 0.012 |
AA + CC | 0.70 | 0.34 | ||||||||
Biceps SFT (mm) | AC | 14.53 | 8.61 | 0.746 | −0.634–2.126 | 0.289 | 0.908 | −0.376–2.193 | 0.165 | 0.165 |
AA + CC | 13.79 | 8.77 | ||||||||
Triceps SFT (mm) | AC | 24.19 | 11.70 | 2.202 | 0.349–4.054 | 0.020 | 2.297 | 0.472–4.121 | 0.014 | 0.027 |
AA + CC | 21.99 | 11.61 | ||||||||
Abdominal SFT (mm) | AC | 37.86 | 17.09 | 3.909 | 1.219–6.600 | 0.004 | 3.729 | 1.090–6.350 | 0.006 | 0.018 |
AA + CC | 33.95 | 16.78 | ||||||||
Supra-iliac SFT (mm) | AC | 30.66 | 15.21 | 3.655 | 1.244–6.065 | 0.003 | 3.359 | 1.053–5.665 | 0.004 | 0.018 |
AA + CC | 27.01 | 15.14 | ||||||||
Thigh SFT (mm) | AC | 35.59 | 18.34 | 3.453 | 0.475–6.430 | 0.023 | 3.61 | 0.681–6.551 | 0.016 | 0.027 |
AA + CC | 32.14 | 19.16 | ||||||||
Sub-scapular SFT (mm) | AC | 26.27 | 11.56 | 2.021 | 0.134–3.907 | 2.048 | 0.036 | 0.187–3.909 | 0.031 | 0.037 |
AA + CC | 24.25 | 12.15 | ||||||||
%BF | AC | 28.52 | 9.90 | 1.743 | 0.198–3.280 | 1.769 | 0.027 | 0.250–3.270 | 0.022 | 0.029 |
AA + CC | 26.77 | 9.54 |
Note: Association of the CLOCK gene variant rs6843722 with anthropometric parameters was found using over-dominant genetic model by linear regression. The β, CI, and p values are provided before and after age and gender adjustment. Benjamini–Hochberg method was used for the correction of multiple comparisons. The effect size (β) along with 95% confidence intervals and p values were calculated to seek the impact and strength of the association, respectively. p < 0.05 was considered statistically significant. The statistically significant p values are shown in bold. WC, waist circumference; HC, hip circumference; WHR, waist-to-hip ratio; WHtR, waist-to-height ratio; SFT, skin fold thickness; %BF, body fat percentage; SD, standard deviation; β, effect size; CI, confidence interval; AC, heterozygous genotype carrier; AA + CC, homozygous wild type/mutant genotype carrier.
Lack of association of the CLOCK variants with adiposity-related metabolic and behavioral parameters
Unadjusted | Adjusted for age, gender, and BMI | ||||||||
---|---|---|---|---|---|---|---|---|---|
Parameters (unit of measurement) | Over-dominant model | Mean | SD | β | 95% CI | p | β | 95% CI | p |
SBP (mmHg) | GA | 117.29 | 14.31 | 0.927 | −1.305–3.160 | 0.415 | −0.363 | −2.394–1.669 | 0.726 |
GG + AA | 116.35 | 13.74 | |||||||
DBP (mmHg) | GA | 77.54 | 10.30 | 0.384 | −1.209–1.978 | 0.636 | −0.596 | −2.093–0.901 | 0.435 |
GG + AA | 77.14 | 9.71 | |||||||
VAI (mmol L−1) | GA | 3.08 | 2.00 | 0.083 | −0.244–0.409 | 0.619 | −0.132 | −0.441–0.177 | 0.403 |
GG + AA | 2.99 | 2.10 | |||||||
LAP (mmol L−1) | GA | 55.11 | 40.07 | 10.04 | 3.506–16.59 | 0.003 | 1.102 | −3.463–5.668 | 0.636 |
GG + AA | 44.79 | 42.46 | |||||||
TyG index | GA | 8.58 | 0.66 | 0.010 | −0.086–0.106 | 0.841 | −0.040 | −0.133–0.052 | 0.392 |
GG + AA | 8.57 | 0.54 | |||||||
FBG (mg dL−1) | GA | 102.75 | 19.10 | 1.637 | −1.544–4.818 | 0.313 | 0.390 | −2.726–3.505 | 0.806 |
GG + AA | 101.06 | 20.91 | |||||||
Fasting insulin (μl U mL−1) | GA | 23.59 | 14.02 | 1.576 | −0.630–3.783 | 0.161 | −0.200 | −2.177–1.776 | 0.842 |
GG + AA | 21.94 | 13.77 | |||||||
HOMA-IR | GA | 6.087 | 4.14 | 0.486 | −0.161–1.133 | 0.140 | −0.061 | −0.639–0.517 | 0.836 |
GG + AA | 5.579 | 4.00 | |||||||
TC (mg dL−1) | GA | 150.27 | 38.94 | −2.349 | −8.634–3.936 | 0.463 | −4.815 | −11.032–1.402 | 0.129 |
GG + AA | 152.48 | 40.16 | |||||||
TG (mg dL−1) | GA | 121.33 | 66.52 | 3.165 | −7.703–14.03 | 0.568 | −2.590 | −12.865–7.685 | 0.621 |
GG + AA | 117.94 | 70.26 | |||||||
HDL-C (mg dL−1) | GA | 30.55 | 8.76 | 0.409 | −1.062–1.879 | 0.585 | 0.503 | −0.970–1.976 | 0.503 |
GG + AA | 30.10 | 9.74 | |||||||
LDL-C (mg dL−1) | GA | 92.07 | 35.76 | 1.151 | −4.602–6.903 | 0.695 | −2.287 | −7.769–3.195 | 0.413 |
GG + AA | 90.79 | 36.67 | |||||||
VLDL-C (mg dL−1) | GA | 24.25 | 13.16 | 0.407 | −1.742–2.556 | 0.710 | −0.665 | −2.701–1.371 | 0.521 |
GG + AA | 23.80 | 13.88 | |||||||
CRI | GA | 5.22 | 1.65 | −0.138 | −0.411–0.136 | 0.324 | −0.255 | −0.519–0.009 | 0.058 |
GG + AA | 5.365 | 1.79 | |||||||
LDL-C/HDL-C | GA | 3.13 | 1.24 | −0.020 | −0.216–0.176 | 0.839 | -.0151 | −0.329–0.028 | 0.099 |
GG + AA | 3.15 | 1.22 | |||||||
TG/HDL-C | GA | 4.29 | 2.99 | 0.019 | −0.468–0.505 | 0.940 | −0.226 | −0.678–0.225 | 0.325 |
GG + AA | 4.28 | 3.13 |
Note: Association between rs4864548 and adiposity-related metabolic traits was determined by linear regression using over-dominant genetic model. The p values before and after adjustment for age, gender and BMI are provided. SBP, systolic blood pressure; DBP, diastolic blood pressure; VAI, visceral adiposity index; LAP, lipid accumulation product; TyG, product of triglycerides and glucose; FBG, fasting blood glucose; HOMA–IR, homeostatic model assessment–insulin resistance; TC, total cholesterol; TG, triglyceride; HDL-C, high-density-lipoprotein cholesterol; LDL-C, low-density-lipoprotein cholesterol; VLDL-C, very-low density-lipoprotein cholesterol; CRI, Coronary Risk Index; LDL-C/HDL-C, LDL-C/HDL-C ratio; TG/HDL-C, triglyceride-to-HDL-C ratio; BMI, body mass index; SD, standard deviation; CI, confidence interval; GA, heterozygous genotype carrier; GG + AA, homozygous wild type/mutant genotype carrier.
Unadjusted | Adjusted for age, gender, and BMI | ||||||||
---|---|---|---|---|---|---|---|---|---|
Parameters (unit of measurement) | Over-dominant model | Mean | SD | β | 95% CI | p | β | 95% CI | p |
SBP (mmHg) | AC | 117.42 | 14.03 | 1.210 | −1.018–3.439 | 0.286 | −0.128 | −2.150–1.894 | 0.901 |
AA + CC | 116.21 | 14.03 | |||||||
DBP (mmHg) | AC | 77.42 | 10.91 | 0.019 | −1.219–1.965 | 0.646 | −0.582 | −2.072–0.909 | 0.444 |
AA + CC | 77.19 | 10.68 | |||||||
VAI (mmol L−1) | AC | 3.04 | 1.96 | −0.001 | −0.327–0.326 | 0.997 | 0.536 | −0.460-.155 | 0.331 |
AA + CC | 3.04 | 2.14 | |||||||
LAP (mmol L−1) | AC | 53.47 | 38.81 | 7.010 | .430–13.591 | 0.037 | −0.547 | −5.097–4.002 | 0.813 |
AA + CC | 46.46 | 44.01 | |||||||
TyG index | AC | 8.58 | 0.66 | 0.021 | −0.075–0.117 | 0.671 | −0.022 | −0.115–0.069 | 0.624 |
AA + CC | 8.56 | 0.54 | |||||||
FBG (mg dL−1) | AC | 102.70 | 19.00 | 1.595 | −1.583–4.773 | 0.325 | 0.892 | −2.209–3.993 | 0573 |
AA + CC | 101.11 | 21.01 | |||||||
Fasting insulin (μl U mL−1) | AC | 23.38 | 13.58 | 1.218 | −0.991–3.428 | 0.279 | −0.397 | −2.367–1.572 | 0.692 |
AA + CC | 22.16 | 14.24 | |||||||
HOMA-IR | AC | 6.022 | 4.03 | 0.377 | −0.271–1.025 | 0.254 | −0.099 | −0.675–0.477 | 0.736 |
AA + CC | 5.64 | 4.13 | |||||||
TC (mg dL−1) | AC | 151.45 | 40.01 | 0.216 | −6.067–6.499 | 0.946 | −1.593 | −7.795–4.608 | 0.614 |
AA + CC | 151.24 | 39.09 | |||||||
TG (mg dL−1) | AC | 121.99 | 66.04 | 4.747 | −6.110–15.60 | 0.391 | −0.809 | −11.043–9.42 | 0.877 |
AA + CC | 117.24 | 70.71 | |||||||
HDL-C (mg dL−1) | AC | 30.68 | 8.84 | 0.724 | -.746–2.194 | 0.334 | 0.895 | −0.572–2.363 | 0.231 |
AA + CC | 29.96 | 9.66 | |||||||
LDL-C (mg dL−1) | AC | 92.47 | 36.53 | 2.110 | −3.637–7.856 | 0.471 | −0.922 | −6.384–4.539 | 0.740 |
AA + CC | 90.36 | 35.81 | |||||||
VLDL-C (mg dL−1) | AC | 24.26 | 13.22 | 0.485 | −1.662–2.632 | 0.657 | −0.574 | −2.602–1.454 | 0.579 |
AA + CC | 23.78 | 13.82 | |||||||
CRI | AC | 5.23 | 1.68 | −0.112 | −0.386–0.161 | 0.421 | −0.221 | 0.484–0.042 | 0.100 |
AA + CC | 5.35 | 1.76 | |||||||
LDL-C/HDL-C | AC | 3.13 | 1.23 | −0.015 | −0.211–0.181 | 0.879 | −0.139 | −0.317–0.039 | 0.127 |
AA + CC | 3.15 | 1.23 | |||||||
TG/HDL-C | AC | 4.30 | 2.94 | 0.016 | −0.469–0.502 | 0.947 | −0.238 | −0.687–0.211 | 0.299 |
AA + CC | 4.28 | 3.17 |
Note: Association between rs6843722 and adiposity-related metabolic traits was determined by linear regression using over-dominant genetic model. The p-values before and after adjustment for age, gender and BMI are provided. SBP, systolic blood pressure; DBP, diastolic blood pressure; VAI, visceral adiposity index; LAP, lipid accumulation product; TyG, product of triglycerides and glucose; FBG, fasting blood glucose; HOMA–IR, homeostatic model assessment–insulin resistance; TC, total cholesterol; TG, triglyceride; HDL-C, high-density-lipoprotein cholesterol; LDL-C, low-density-lipoprotein cholesterol; VLDL-C, very-low density-lipoprotein cholesterol; CRI, Coronary Risk Index; LDL-C/HDL-C, LDL-C/HDL-C ratio; TG/HDL-C, triglyceride-to-HDL-C ratio; BMI, body mass index; SD, standard deviation; CI, confidence interval; AC, heterozygous genotype carrier; AA + CC, homozygous wild type/mutant genotype carrier.
Unadjusted | Adjusted for age, gender, and BMI | |||||||||
---|---|---|---|---|---|---|---|---|---|---|
Parameters | Category | Over-dominant model | Counts (%) | OR | 95% CI | p | OR | 95% CI | p | p value correcteda |
Eating behavior | Random | GG + AA | 179 (49.3%) | 0.977 | 0.708–1.350 | 0.889 | 0.937 | 0.672–1.306 | 0.700 | 0.865 |
GA | 184 (50.6%) | |||||||||
Specific | GG + AA | 121 (48.5%) | ||||||||
GA | 128 (51.4%) | |||||||||
Diet consciousness | No | GG + AA | 219 (50.2%) | 0.849 | 0.597–1.206 | 0.360 | 0.825 | 0.577–1.179 | 0.291 | 0.865 |
GA | 217 (49.8%) | |||||||||
Yes | GG + AA | 81 (46.0%) | ||||||||
GA | 95 (54.0%) | |||||||||
TFDF | High | GG + AA | 82 (47.7%) | 1.013 | 0.681–1.508 | 0.949 | 0.952 | 0.631–1.437 | 0.816 | 0.865 |
GA | 90 (52.3%) | |||||||||
Low | GG + AA | 108 (48.0%) | ||||||||
GA | 117 (52.0%) | 0.889 | 0.611–1.293 | 0.539 | 0.907 | 0.615–1.337 | 0.621 | 0.865 | ||
Moderate | GG + AA | 110 (36.7%) | ||||||||
GA | 105 (48.8 %) | |||||||||
Physical activity | Low | GG + AA | 88 (39.3%) | 0.495 | 0.325–0.753 | 0.001 | 1.788 | 1.157–2.763 | 0.009 | 0.865 |
GA | 136 (60.7 %) | |||||||||
Moderate | GG + AA | 127 (53.4%) | 0.570 | 0.394–0.825 | 0.003 | 1.116 | 0.731–1.703 | 0.612 | 0.865 | |
GA | 111 (46.6%) | |||||||||
High | GG + AA | 85 (56.7%) | ||||||||
GA | 65 (43.3%) | |||||||||
Sleep-wake cycle | Irregular | GG + AA | 150 (47.5%) | 1.129 | 0.822–1.552 | 0.453 | 1.053 | 0.760–1.459 | 0.756 | 0.865 |
GA | 166 (52.5%) | |||||||||
Regular | GG + AA | 150 (50.7%) | ||||||||
GA | 146 (49.3%) | |||||||||
Sleep duration | Insufficientb | GG + AA | 167 (48.0%) | 1.092 | 0.793–1.505 | 0.590 | 1.029 | 0.741–1.428 | 0.865 | 0.865 |
GA | 181 (52.0%) | |||||||||
Sufficientc | GG + AA | 133 (50.4%) | ||||||||
GA | 131 (49.6%) |
Note: Association between the rs4864548 and behavioral parameters was sought by logistic regression using over-dominant genetic model. The OR, CI, and p values before and after adjusting for age and gender are provided. The odds ratio along with 95% confidence intervals and p values were calculated to seek the impact and strength of the association, respectively. Benjamini-Hochberg method was used for the correction of multiple comparisons. p < 0.05 was considered statistically significant. Regular sleep timings were taken between 8:00 pm –12:00 and wake timings between 5:00–7.30 am TFDF, Tendency towards fat-dense food; OR, odds ratio; CI, confidence interval; GA, heterozygous genotype carrier; GG + AA, homozygous wild type/mutant genotype carrier.
Unadjusted | Adjusted for age, gender, and BMI | |||||||||
---|---|---|---|---|---|---|---|---|---|---|
Parameters | Category | Over-dominant model | Counts (%) | OR | 95% CI | p value | OR | 95% CI | p value | p value correcteda |
Eating behavior | Random | AA + CC | 174 (49.8%) | 1.095 | 0.793–1.512 | 0.582 | 1.062 | 0.74–1.477 | 0.493 | 0.907 |
AC | 189 (52.1%) | |||||||||
Specific | AA + CC | 125 (50.2%) | ||||||||
AC | 124 (49.8%) | |||||||||
Diet consciousness | No | AC | 220 (50.5%) | 0.909 | 0.640–1.291 | 0.594 | 0.882 | 0.619–1.257 | 0.487 | 0.627 |
AA + CC | 216 (49.5%) | |||||||||
Yes | AA + CC | 83 (47.2%) | ||||||||
AC | 93 (52.8%) | |||||||||
TFDF | High | AA + CC | 82 (47.7%) | 1.031 | 0.693–1.534 | 0.879 | 0.976 | 0.649–1.469 | 0.907 | 0.907 |
AC | 90 (52.3%) | |||||||||
Low | AA + CC | 109 (48.4%) | ||||||||
AC | 116 (51.6%) | 0.931 | 0.641–1.353 | 0.708 | 0.969 | 0.659–1.424 | 0.872 | 0.907 | ||
Moderate | AA + CC | 108 (50.2%) | ||||||||
AC | 107 (49.8) | |||||||||
Physical activity | Low | AA + CC | 93 (41.5%) | 1.745 | 1.149–2.649 | 0.009 | 1.569 | 1.019–2.417 | 0.041 | 0.369 |
AC | 131 (58.5%) | |||||||||
Moderate | AA + CC | 123 (51.7%) | 1.158 | 0.769–1.746 | 0.483 | 1.148 | 0.754–1.747 | 0.520 | 0.907 | |
AC | 115 (48.3%) | |||||||||
High | AA + CC | 83 (55.3%) | ||||||||
AC | 67 (44.7%) | |||||||||
Sleep-wake cycle | Irregular | AA + CC | 151 (47.8%) | 1.093 | 0.796–1.501 | 0.584 | 1.021 | 0.739–1.412 | 0.898 | 0.907 |
AC | 165 (52.2%) | |||||||||
Regular | AA + CC | 148 (50.0%) | ||||||||
AC | 148 (50.0%) | |||||||||
Sleep duration | Insufficientb | AA + CC | 162 (46.6%) | 1.140 | 0.828–1.570 | 0.421 | 1.086 | 0.785–1.504 | 0.618 | 0.907 |
AC | 186 (53.4%) | |||||||||
Sufficientc | AA + CC | 137 (51.9%) | ||||||||
AC | 127 (48.1%) |
Note: Association between the rs6843722 and behavioral parameters was assessed by logistic regression using over-dominant genetic model. The OR, CI, and p values before and after adjusting for age and gender are provided. The odds ratio along with 95% confidence intervals and p values were calculated to seek the impact and strength of the association, respectively. Benjamini-Hochberg method was used for the correction of multiple comparisons. p < 0.05 was considered statistically significant.Regular sleep timings were taken between 8:00 pm –12:00 and wake timings between 5:00–7.30 am. TFDF, Tendency towards fat-dense food; OR, odds ratio; CI, confidence interval; AC, heterozygous genotype carrier; AA + CC, homozygous wild type/mutant genotype carrier.
Discussion
Conclusions
Acknowledgments
Competing interest statement
References
Information & Authors
Information
Published In
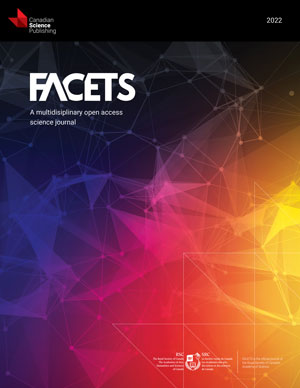
History
Copyright
Data Availability Statement
Key Words
Sections
Subjects
Authors
Author Contributions
Metrics & Citations
Metrics
Other Metrics
Citations
Cite As
Export Citations
If you have the appropriate software installed, you can download article citation data to the citation manager of your choice. Simply select your manager software from the list below and click Download.