Comparison of multiple monitoring techniques for the testing of a scale model timber Warren truss
Abstract
This paper outlines the testing and monitoring procedure of a scale model Warren truss constructed of 2 inch × 4 inch (38 mm × 89 mm) members and bolted connections within a laboratory environment. Several forms of deflection monitoring and strain monitoring instrumentation were utilized throughout this laboratory testing phase of a longer-term research program. Instruments included: an automatic total station, linear variable differential transducers, light detection and ranging, electric strain gauges, and distributed optical fibre sensors. The distributed point load-testing regime included two configurations: (i) the original truss configuration and (ii) the installation of intermediate columns beneath the truss. Objectives of this phase included identifying instrument capabilities, limitations, and overall reliability/effectiveness with respect to representing the behaviour of the truss system. In addition, members of interest and critical monitoring locations along the Warren truss were determined. The purpose of this laboratory endeavour was to determine an optimized structural-health monitoring program prior to implementation in a heritage timber Warren truss structure within the infrastructure inventory of the Department of National Defence (DND). An options analysis of monitoring techniques was conducted whereby the effectiveness of each instrumentation type was evaluated according to relevant metrics/factors to determine an effective monitoring technique for this heritage building and other similar DND truss structures.
1. Introduction
Hangar 1 located at Canadian Forces Base (CFB) St-Jean, Québec, is one of approximately 70 Warren truss structures maintained by the Department of National Defence (DND). Built in 1942, Hangar 1 consists of eleven 68-m long, double-span, parallel Warren trusses. As shown in Fig. 1, the Douglas fir Warren trusses are comprised of (i) bending members including double top and bottom chords and (ii) axial members including vertical struts and diagonal web members. Significant rehabilitation efforts have been made across the DND to ensure that these 80-year-old heritage structures remain serviceable. Repairs and modifications include post-tensioned cables, member replacement, and other conventional timber repair methods (clamping, epoxy injection, and gusset plates, etc.,). In addition to these repair efforts, intermediate columns were installed at the mid-span joints to decrease maximum truss deflections in Hangar 1. Due to these complex repair efforts, material degradation, and environmental stresses, it was perceived that snow loads potentially exceed the serviceability and structural capacity limits of the structure. Subsequently, evacuations of the building (and five other similar truss structures at CFB St-Jean) were/are ordered based on a nominal snow accumulation of 10 cm. Consequently, the impact of these evacuations on the operational capabilities of CFB St-Jean warranted a research-based investigation to include a sustainable Structural Health Monitoring (SHM) program for emergency purposes.
Fig. 1.

The primary purpose of this laboratory endeavour was to determine an optimal SHM program to be employed within Hangar 1. Prior to the installation of monitoring equipment and instrumentation, a stage-wise approach was adopted to maximize the effectiveness of the in-situ SHM program. This stage-wise approach included the construction and testing of a one-quarter size, scaled-down Warren truss within a controlled laboratory setting. Numerous forms of deflection and strain monitoring instrumentation were utilized throughout several loading scenarios. Metrics were applied to determine the effectiveness of each type of monitoring technique. Lastly, lessons learned during the laboratory experiments aided in the development of an optimized monitoring procedure. Objectives during this experimental procedure included:
1.
familiarization and training of personnel for each monitoring technique;
2.
developing an understanding of instrumentation capabilities and limitations;
3.
developing an understanding of the Warren truss behaviour through various loading scenarios;
4.
determining the critical monitoring locations and members of interest along the Warren truss;
5.
comparing the various forms of monitoring techniques, in terms of their ability to capture the behaviour of the laboratory truss specimen;
6.
creating standard operating procedures of how to organize/display the data, remotely access the data, establish alarm systems, and establish communication techniques; and
7.
gaining an understanding of how to deploy the equipment and instrumentation within Hangar 1.
2. Background
2.1. Structural health monitoring
The benefits of SHM within civil engineering include extending lifespan, contributing to infrastructure management, and increasing occupant safety (Chang et al. 2003). Long-term sensing capabilities, temperature and environmental interference, data acquisition, sensor placement, among other numerous factors must be considered during the design of a SHM program (Hannan et al. 2018). A robust SHM program is capable of performing three forms of monitoring: knowledge monitoring to assess the structure and determine structural behaviour, control monioring to include periodic testing, and emergency monitoring to provide an early warning system. An efficient SHM program for Hangar 1 must satisfy the three monitoring forms. As such, the program must be capable of providing data remotely, continuosuly, and in the long term. Furthermore, in terms of emergency monitoring, the program must incorporate an alarm system capable of communicating the level of risk to the occupants of Hangar 1 in real time. Lastly, the program must be nonintrusive and minimize disruptions in the day-to-day operations that occur within Hangar 1. With the minimum SHM criteria now finalized, the measurands required to meet these robust conditions were determined. These measurands were determined to be deflections, structural behaviour (stress/strain, global and localized), in-situ moisture properties, and internal as well as external environmental effects/conditions.
2.2. DND Warren truss structures
SHM programs have been designed and implemented on DND Warren trusses (Locklin et al. 2017). Conventional electric strain gauges, discrete monitoring using fibre optic sensors, vibration and wave-propagation based sensors, and temperature sensors were utilized. However, high data volumes and environmental conditions limited the effectiveness of this SHM endeavour. Moreover, the program did not include deflection instruments or humidity sensors, the latter being essential for timber monitoring. Locklin et al. (2017) also noted the importance of creating a more refined system of sensors thus underlining the importance of determining an optimized SHM program prior to in-situ implementation. Accordingly, the implementation of a more robust and redundant system, combined with a monitoring regime to capture internal and external environmental factors, will lead to the determination of an optimized SHM method. Lastly, previous monitoring endeavours were not capable of collecting data remotely, in real time, or communicating with the user. As such, this monitoring plan must incorporate these capabilities to be efficient for emergency monitoring.
2.3. Timber and truss monitoring instrumentation
The type of instruments utilized in a SHM project is dependent on the nature of the project including the type of structure, material, and loading arrangements, among others. In addition, instrument selection is determined by the requirements of the actual SHM program (i.e., research questions that need to be answered). Several forms of instrumentation were considered during the laboratory component of the overall research program. The instrumentation as part of the monitoring program can be divided into the monitoring of deflections, strains/stresses, and environmental conditions.
Deflection or displacement monitoring is a significant portion of SHM, particularly for serviceability requirements. Linear variable differential transducers (LVDT) have been used extensively for timber monitoring including the in-situ, full-scale load testing of historic timber systems (Foo and Seckin 1988; Branco et al. 2017). Selected limitations include temperature sensitivity, limited range capability, and the requirement of a stable reference system (Moreu et al. 2015), which is a particular issue for the monitoring of long-span structures. The automatic total station (ATS) has been successfully employed in numerous static SHM applications (Merkle and Myers 2004; Psimoulis and Stiros 2013). The autonomous nature of the ATS makes it an ideal instrument for remote, continuous monitoring. The ATS is limited by line of sight and is restricted to determining the behaviour at discrete locations. Conversely, light detection and ranging (LiDAR) can accurately provide distributed global deflection data within a structure (Bertolini et al. 2015). The requirement of extensive postprocessing and the inherent nonautonomous nature of LiDAR limit its effectiveness for emergency monitoring. Other challenges include line-of-sight requirements and large data files.
Electric strain gauges (ESG) are the most traditional technique and a proven method for measuring strain at discrete locations, for both laboratory and field SHM projects, and have been used for the testing and monitoring of timber truss structures (Lanata 2015; Locklin et al. 2017; Helmer-Smith et al. 2021). The reliability of these conventional gauges for general timber monitoring is demonstrated by the field applications described by Palma and Steiger (2020) and Cavalli and Togni (2014). Various types of strain gauges have also been extensively used in steel truss field monitoring programs (Bhalla et al. 2009; Park et al. 2013; Moreu et al. 2017). Highlighted limitations include long-term sensor creep and drift. As such, it is necessary to consider a compensation or reference gauge throughout the long-term program. The correct installation of a strain gauge is nontrivial and requires attention to detail in terms of alignment, type of gauge, epoxy, connectivity, and placement. One inherent limitation of the ESG is that they are restricted to local data points, thus, it is important to optimize the placement of sensors. In addition, this limitation may lead to strain measurements that are misleading or nonrepresentative of the true timber properties due to the high variability and nonhomogeneity of timber (Palma and Steiger 2020). Conversely, fiber optic sensors (FOS) are an emerging form of instrumentation due to their capabilities of providing local, quasi-distributed, or fully distributed strain data. Quasi-distributed fibre bragg grating (FBG) sensors were used by Locklin et al. (2017) and proved more stable than the conventional strain gauges. However, large data files obtained from the FBG sensors were highlighted as a major limitation. Similarly, FOS have been respectively utilized for the quasi-distributed and distributed strain monitoring of historical buildings (Whelan et al. 2002; Bastianini et al. 2005). Distributed FOS have proven to be a successful instrument in laboratory monitoring endeavours (Kwon et al. 1998; Van Der Kooi and Hoult 2018). A steel model truss was instrumented with distributed FOS and loaded under two loading conditions to (i) determine FOS capabilities in capturing local strains and (ii) identify effects of different joint conditions. It was determined that the distributed sensors were successful in capturing complex axial and bending strains as multiple FOS were installed on different faces (top and bottom) of the same member. The effects of different joint conditions, namely fixity in connections, on the overall structure was also captured by the FOS (Van Der Kooi and Hoult 2018). Distributed FOS have also been extensively used as a practical field-monitoring application in recent years. Specific to truss monitoring, distributed FOS accurately measured bending moments along the top and bottom chords of an in-service steel truss railway bridge (Van Der Kooi et al. 2018). In addition, the strain-sensing system captured the general behaviour of members as well as the effects of connections, such as gusset plates. FOS applications are well documented; the reader is referred to Bado and Casas (2021) for a complete review of recent civil engineering applications. A practical issue that affects the performance of FOS is the installation procedure including bonding, adhesive types, and sensor placement issues. Overall, both strain measuring technologies are proven methods in both laboratory and field monitoring applications. Notably, FOS may compliment discrete sensors by providing a more general and complete insight into the behaviour of the structure (Barker et al. 2019). Moreover, the distributed capabilities of the FOS capture general behaviour that may not be observed by conventional, discrete, and local instruments such as strain gauges (Van Der Kooi and Hoult 2018).
Lastly, humidity, temperature, and moisture content are all environmental factors that influence the equilibrium moisture content and subsequent shrinking and swelling of timber members (CSA 2015). Temperature sensors have been extensively coupled with other SHM instruments for validation purposes (Tennyson et al. 2001; Locklin et al. 2017). Moisture sensors and humidity sensors have also been incorporated into past timber monitoring programs (Dietsch et al. 2014; Björngrim et al. 2016). Overall, the inclusion of environmental sensors allows for a correlation between environmental conditions and the corresponding building response.
The laboratory stage provided a means to determine the capabilities and limitations of each instrumentation method. Similarly, this research allowed for a comprehensive comparison of instruments in terms of their effectiveness in monitoring timber elements. As such, within the context of an options analysis, a weighted matrix was utilized to compare the various forms of monitoring. Several factors were considered to determine the effectiveness of the instruments including: accuracy; autonomy; real-time, long-term, and continuous capabilities; installation process; site-specific limitations; operational ability on-site; alarm system compatibility; ease of use; training requirements; and overall cost.
3. Numerical modeling and analytical method
SAP2000® was utilized to produce a 2-D, linear, numerical model of the laboratory truss as shown in Fig. 2. The global geometry of the model in terms of length and height reflected the laboratory truss arrangement (and related properties). The sectional properties of the truss elements were represented as two 2 inch × 4 inch (38 mm × 89 mm) elements, with the exception of the two 2 inch × 8 inch (38 mm × 184 mm) elements utilized at the ends of the truss. The orthotropic material properties of spruce–pine–fir (SPF) No. 2 discussed in Section 4.1 were input into the model. A roller-pin support type was utilized to represent the end support conditions (L0 and L14) in the numerical model. Out-of-plane bending was restricted at the top end connections (U0 and U14) of the truss as metal clamps were utilized within the laboratory (see Fig. 3). Roller supports were utilized to represent the intermediate column support conditions. Loading was applied as distributed point loads of 0.7 kN at each vertical strut. Moment releases were added at both ends of the axial force members (vertical struts and diagonal web members) to represent pinned connections. Linear material properties were utilized, as nonlinear behaviour was not expected due the small loading increments utilized during laboratory testing. It was determined that a 2-D model, in lieu of a 3-D model, would suffice in representing and idealizing the global behaviour of the truss. Analytical calculations, by means of the direct stiffness method, were also performed to validate the numerical model. The direct stiffness method utilized a 96 × 96 matrix to calculate deflections, axial stresses, shear stresses, and bending moments. Similar to the numerical model, top and bottom chords were analyzed as beam (fixed connections) elements, and axial members (vertical struts and diagonal web members) were considered as truss (pinned connections) elements. The analytical results successfully validated the numerical model as selected results were within a 1% margin.
Fig. 2

Fig. 3.

4. Experimental procedure and methodology
4.1. Laboratory specimen
A one-quarter scale Warren truss was designed, constructed, monitored, and tested within the Royal Military College (RMC) Civil Engineering structures laboratory as shown in Fig. 3. The truss was constructed with SPF No. 2 2 inch × 4 inch (38 mm × 89 mm) members, placed to model the in-situ truss, with the notable exception of the variable-sized web members in Hangar 1 (see Fig. 1). The connections were composed of half-inch bolts and half-inch malleable iron washers. The assumed orthotropic properties of SPF No. 2 used for the truss and associated with the numerical model are outlined in Table 1.
Table 1.

4.2. Instrumentation
The instrumentation outlined in Table 2 was utilized, compared, and evaluated throughout the experimental procedure and further employed as part of the in-situ SHM portion of the overall research program. As this paper focusses on the laboratory portion, the reader is referred to Helmer-Smith et al. (2021) for information regarding the in-situ employment of instrumentation within Hangar 1 for local point-load testing. Instrument locations were selected based on preliminary modeling results and several redundant instruments were placed for comparison purposes. As such, the instrumentation could be refined and optimized in terms of the number of sensors as data files were considerable. The multiple forms of SHM instrumentation are shown in Fig. 4. The locations of selected instruments are detailed in Fig. 5.
Table 2.
Instrumentation | Purpose |
---|---|
Deflection/Serviceability Monitoring | |
Automatic total station. Leica TM50: 12 Targets, 2 Control Points. DAQ: GeoMoS Monitor and Analyser | Monitor 3-D deflections of the Warren Truss, utilizing precision targets mounted on selected locations. |
Terrestrial Laser Scanner. Leica ScanStation P40/P30. DAQ: Cyclone 9.1 | Monitor 3-D deflections along the entire Warren truss. Multiple set-ups/scans for change detection over time. |
Linear variable differential transducer. 3 – LDS – 50. DAQ: QUANTUMX and Catman®AP | Monitor vertical deflections of the Warren truss at selected locations. |
Strain Monitoring | |
Electric strain gauges. 24 gauges, KFG-5-120-C1-11 DAQ: QUANTUMX and Catman®AP | Measure strain at selected locations on the Warren truss. |
Fibre optic sensors.DAQ: Luna ODiSI-B – 4 members | Measure distributed strain along the truss at selected structural members. |
Environmental Monitoring | |
Pin penetration moisture meter: Delmhorst Navigator Pro | Measure the in-situ moisture content of the wood. |
Temperature/humidity sensors: 2 HTM2500LF Sensors DAQ: QUANTUMX & Catman®AP | Measure the environmental conditions within the structure and correlate instrument response. |
Note
DAQ, data acquisition.
Fig. 4.

Fig. 5.

A QUANTUM X data acquisition system (DAQ) was used throughout the experimental procedure for the conventional instrumentation utilized as part of this investigation. The software employed for the monitoring procedure was Catman®AP and was compatible with all monitoring instruments except the ATS, LiDAR, and the FOS, as these required specific (proprietary) post-processing software programs. As shown, conventional strain gauges (SG) were installed on several Warren truss members. Multiple gauges were installed on the front (F) and back (B) of the same member (i.e., SG 1(F) and SG 14(B) installed on member L0U1) to determine bending effects. Moreover, the average of the two strain results (given that the gauges are installed front and back) provides the pure axial strain. Employing multiple gauges is also a redundant measure in the case of damaged or unreliable gauges. Finally, the multiple gauges allow for an averaged and more realistic result due to the high variability of timber properties. FOS were employed to capture the internal mechanisms along the entire member, as data were not restricted to localized, discrete points. This provision of distributed strain data is a unique capability of distributed optical sensing techniques. In this experimental procedure, the Luna ODiSI-B (Optical Distributed Sensor Interrogator) was considered. In comparison with other commercially available optical strain sensing technologies, the ODiSI-B can provide an array of strain measurements along a specimen of study. The technology uses a standard, low-cost telecom optical fibre as the transducer and can resolve strain at a spatial resolution (gauge length) as fine as 0.65 mm along a fibre upwards of 40 m in total length (Forbes et al. 2018). Accordingly, the ODiSI-B solution was selected to investigate the strain distribution and potential inhomogeneities across truss members. Limited information was found on using this FOS technology with timber structures. Several installation procedures were trialed. These included, (i) using two adhesives types to bond the FOS: loctite acrylic adhesive and five-minute epoxy resin and (ii) testing two variations of the protective layering along the FOS: nylon protective coating layer (900 μm approximate outer diameter) and acrylate coating (250 μm approximate outer diameter).
4.3. Testing scenarios for a one-quarter scale Warren truss specimen
Five distributed point-load tests (DPLT), with intermediate columns at varying locations, were conducted throughout the experimental procedure as shown in Fig. 6. Intermediate columns were not utilized for Test 1 and Test 5. Test 2 represents the location of the in-situ intermediate columns in Hangar 1. Varying locations of the intermediate columns were considered for Test 3 and Test 4. The DPLT were conducted using weight plates that were manually placed incrementally at each vertical strut along the truss. The total loading on each vertical strut was 0.7 kN, representing a total load of 4.2 kN. The instruments utilizing Catman®AP collected data continuously at a frequency of 1 Hz. The ATS, LiDAR, and FOS data were collected at each load increment.
Fig. 6.

5. Selected results and discussion
5.1. Instrument capabilities and limitations as observed during laboratory experiments
The laboratory stage developed an understanding of the key capabilities and limitations of each instrument. This understanding is crucial in optimizing the SHM program and determining a suitable method for employing the monitoring instruments. Table 3 summarizes the capabilities and limitations of each respective instrument as observed during the laboratory stage. In addition, the error or data variation associated with each instrument is outlined and the requirement for control points or reference instruments is noted. The error in each instrument was determined by variation in control point measurements (ATS and LiDAR) or data noise at zero loading (LVDT, ESG, FOS). Table 3 can be used to analyze and compare SHM instruments for various monitoring projects.
Table 3.
![]() |
![]() |
![]() |
5.2. Instrument reliability and accuracy
This section summarizes selected results based on the data obtained from the various instruments and monitoring methods employed in this laboratory research program. The data are discussed within the framework of their reliably and accuracy in determining the behaviour of the Warren truss system for each of the testing scenarios.
5.2.1. Deflection data
Deflection monitoring instruments including LiDAR, LVDTs, and an ATS captured the deflection of the truss throughout each loading scenario. The variation between each respective instrument provides an insight into the accuracy and reliability of the instrument and (or) methodology in conjunction with the respective calibrations and controls that were conducted for each method/instrument. Data captured by each instrument at the location of ATS targets during Test 1 are graphically compared in terms of their effectiveness in capturing the behaviour of the truss as shown in Fig. 7. Graphically, the LVDTs recorded lower deflection values, specifically, at ATS Target 7, as compared with the other instruments. An unsatisfactory stable reference system resulted in vertical plumbness issues thereby influencing LVDT deflection results. It must be noted that this error is human induced due to poor installation of the physical LVDT and does not reflect poor LVDT performance. However, it demonstrates the level of difficulty associated in accurately monitoring a long-span structure using contact deflection instruments as specific care must be taken to ensure a stable reference system is provided. This specific issue may be compounded due to on-site limitations or building usage requirements. The LiDAR and the ATS data appeared to agree with one another within 20%. Comparing the three forms of instrumentation, the ATS provided the most conservative data while still providing accurate results. This can be stated with confidence as this is a 1-second instrument and external control was used as per standard geomatics control procedures. LiDAR was able to accurately capture the distributed deflections using change detection along the entire truss. The accuracy of this technique was determined to be 0.12 mm at 10 m (well within product specifications of 3 mm at 50 m), and control points were also used for this method.
Fig. 7.

Unforeseen issues including rigid body motion (RBM) of the truss or support settlement caused excessive deflections throughout the experiments. RBM may have occurred at the supports as the truss was simply resting on supports rather than physically connected. The varying locations of the intermediate columns (Tests 2, 3, and 4) during the test program caused load reversal in certain members that may have resulted in lower stiffness properties and contributed to higher deflections. Finally, the consecutive loading and unloading between tests may have caused permanent deformation leading to greater deflections in subsequent tests. Specifically, average deflection results in Test 5 were 40% greater than results obtained during Test 1. As such, it was ineffective to compare deflection instrument results with model and analytical results as well as difficult to relate deflection results with strain results.
5.2.2. Strain data
Both conventional strain gauges and state-of-the-art distributed FOS were utilized to capture the strain behaviour of selected truss members. Axial strain results from Test 1 are compared with model and analytical results in Fig. 8. The two instruments provide compatible results within a 9% margin and as such reliably provide the local strain behaviour of the truss. The instrument results were compared with the model results for the purpose of model validation. The fibre optics provided results within 1%–5% of model and analytical results, and the strain gauges provided results within 4%–8% of model and analytical results. Conversely, FOS results at SG 6 were approximately 100% less than strain results obtained by SG 6. The observed discrepancy may be due to natural timber defects (i.e., knots or cracking) and (or) FOS application issues (i.e., poor bonding or alignment issues). This indicates the importance of redundant instruments on multiple faces of a member. Combined bending and axial strain results along certain points of the bottom chord are shown in Fig. 9. Results were within 2%–6% between the strain gauge and fibre optics. In addition, the fibre and strain experimental data were within 1%–7% of the average of model and analytical results.
Fig. 8.

Fig. 9.

Although both instruments are reliable in providing strain data, the effectiveness of the FOS versus the conventional strain gauge is demonstrated in Fig. 10. As shown, the FOS captures a nonuniform strain profile in an axial member indicating out-of-plane bending. However, the local data provided by the strain gauge does not indicate this out-of-plane bending. The high level of noise in Fig. 10 may also demonstrate the variability of timber properties such as knots, cracking, and other timber defects along the surface. Placement of instruments on these defects may lead to localized results that do not represent the general properties of the timber member. Thus, the use of distributed instruments is recommended to determine an average result that better represents the true timber properties. Overall, the distributed FOS gives a more reliable insight into the continuous strain behaviour of selected members as compared with the conventional strain gauges, which only provide discrete strain data.
Fig. 10.

5.3. Capturing Warren truss behaviour
An objective within this research was to identify and capture the general behaviour of the Warren truss. In doing so, critical monitoring locations and members of interest were determined to optimize the in-situ SHM program.
5.3.1. Optimal deflection monitoring locations
Optimal deflection monitoring locations for all load test set-ups were determined. Maximum ATS results from Test 1 and Test 2, compared in Fig. 11, demonstrate the critical monitoring locations for Warren trusses with intermediate columns (in-situ truss) and without intermediate columns. In addition, it highlights the effectiveness of the intermediate columns in terms of increasing truss serviceability as the maximum deflections in Test 2 are significantly reduced when compared with Test 1. The average calculated percent reduction in deflection is 78% due to the installation of the intermediate columns. Inherently, the optimal deflection monitoring locations (with the aim to monitor point of maximum deflection) for trusses without intermediate columns is at ATS point 6 or the mid-span. Conversely, the optimal deflection monitoring location for a truss with intermediate columns is at ATS points 2 and 3. Other deflection trends were observed using Fig. 11. ATS Points 4, 5, and 8 indicate that diagonal web members are noncritical members in terms of deflection monitoring. In addition, ATS Point 2 is deflected 0.25 mm more than ATS Point 3 in both tests, demonstrating that the top chord deflects slightly more than the bottom chord at the same point along the truss. These trends were also observed in the numerical modeling and analytical results.
Fig. 11.

5.3.2. Optimal strain monitoring locations
Members of interest were determined using the numerical model and laboratory experiments with an aim to identify optimal strain monitoring locations. Laboratory results identified members of interest for the Warren truss with intermediate columns reflecting the in-situ truss. Load reversal in a double web member between Test 1 and Test 2 is shown in Fig. 12. Orientated parallel to grain, the average of the four strain gauges represents the axial force experienced by the web member. As shown, the double diagonal web member switched from tension (as it is originally designed) to compression. This is noteworthy because the calculated compressive resistance of the double web members is approximately 25% of the calculated compressive resistance of the single web member. In addition, it may be assumed that the design capacity of a load-reversed member can be reduced by 10% (Freas 1982). Load and bending reversal were captured by the distributed FOS, conventional strain gauges, analytical results, and numerical modelling. Overall, eight axial members and six bending members succumbed to stress reversal as shown in Fig. 13. Members L4U5, L6U5, L4L6, and their respective mirrored counterparts are considered members of interest for in-situ monitoring. The additional columns also caused members L6U5 and L8U9 to undergo bending as fixity was introduced at the location of the intermediate columns. This is demonstrated in Fig. 14 as strain gauges 6 and 7 display opposite strain magnitudes. This is important to highlight, as the web members are not designed as bending members and as such should have the same strain magnitude.
Fig. 12.

Fig. 13.

Fig. 14.

5.4. Fibre Optic sensor discussion
An initial comparison between the measured FOS strain data on the wooden truss members and that from previous experiments investigating steel supporting members (Forbes et al. 2018) determined the strain measurements to be much less consistent. Two types of protective coatings were trialed along the bottom chord: a nylon protective layer and an acrylate coating. The two optical fibre strain profiles from Test 1 are compared in Fig. 15. As shown, both provide similar strain profiles; however, there is a high level of localized noise. It was hypothesized that this high noise level was a result of inconsistent bonding between the wood face and the fibre due to the inhomogeneity of wood. Thus, warranting further bonding development and testing for FOS installations on wood. Specifically, more testing should be conducted, within a laboratory setting, to determine optimal adhesive types, coating/fibre types, surface preparation procedures, and application/installation techniques for FOS applications on timber members.
Fig. 15.

Due to the relatively large data files obtained along a single sensor (i.e., thousands of strain measurement points), it would be necessary to restrict the data acquisition rate of the FOS without implementation of local post-processing (e.g., change detection). Such development is not prohibitive using the ODiSI-B, which is controlled by a personal computer, but was not investigated within this research effort. Accordingly, the commercial off-the-shelf FOS solution is less effective as an emergency monitoring technique. The FOS proved to provide reliable distributed strain data along the entire truss during testing, as opposed to the local strain data provided by the strain gauges. Within the scope of this research, FOS was determined to be most effective for control/knowledge monitoring, measuring a strain profile of the truss that is not practically feasible with discrete gauges. In this sense, the FOS was utilized based on its spatial resolution rather than its temporal resolution.
5.4. Discussion – instrument comparison
To effectively compare monitoring methods, with an aim to optimize the in-situ monitoring program, an option analysis was conducted. Each respective instrument/method was assigned a numerical value from 1 (lowest) to 5 (highest) for each given parameter. The parameters and respective metrics and (or) criteria that were used to assess the various instrumentation methods included:
1.
Accuracy and reliability. The instrument provides accurate results and reliably indicates the behaviour of the truss. Limiting factors include deviation from true value, data noise, and the requirements for control or redundant measures. A distributed data capability is also favoured.
2.
Autonomy. The instrument and DAQ system can effectively operate/post-process autonomously and requires little routine maintenance. Limiting factors include extensive post-processing and the requirement for on-site data collection.
3.
Real-time, long-term, and continuous capabilities. The instrument/DAQ system can operate and provide processed data in real time, continuously, and in the long term. Limiting factors include high data volumes, extensive post-processing, and the requirement for on-site maintenance.
4.
Installation process/ease of installation. The installation process is an efficient and simple one-time set-up. Limiting factors include the need for a stable reference system, multiple tools/hardware, on-site equipment, and degree of deviation caused by installation errors (i.e., procedure, location, etc.,)
5.
Site-specific limitations. The instrument is not significantly limited by site specific factors such as shelving, extensive occupant usage, and HVAC systems.
6.
Operational ability on site. The instruments and DAQ system can effectively operate within the in-situ environment. Limiting factors include extensive power requirements, vibration induced errors, and environmental limitations.
7.
Alarm system compatibility. The instrument and DAQ system are compatible with alarm systems, communicate effectively, can be programmed with assigned thresholds, and can be effectively used for emergency monitoring. Limiting factors include extensive post-processing, communication issues, and limited threshold input options.
8.
Ease of use and effort. The instrument and DAQ system can be efficiently utilized for its respective purpose. Limiting factors include extensive labour; multiple tools, hardware, and on-site equipment required for operation; significant troubleshooting; and instrument maintenance.
9.
Cost as a function of capability. The instrument and respective DAQ can be purchased and operated at an efficient cost as a function of its capabilities (i.e., distributed data capabilities are inherently more expensive). Limiting factors include expensive instrument costs, software subscriptions, and maintenance costs.
10.
Training requirements. The instrument and DAQ can be operated without extensive training. Limiting factors include the need for on-site training provided by the instrument or software manufacturer.
Based on the experimental data and lessons learned, the respective instruments were compared using an options analysis based on the abovementioned criteria. The purpose of this analysis is to compare all factors associated with the effective selection of monitoring instruments. The parameters are considered based on the experimental data obtained in terms of accuracy and identifying truss behaviour within the laboratory environment. The lessons learned throughout the experimental procedure were considered and parameters were assigned based on the expected capabilities and limitations in-situ or in real-world installation. For example, the installation process was not a limitation throughout the laboratory endeavour. However, it must be considered greatly for the design of the in-situ program. As such, this metric is applied to the design of the in-situ monitoring project, instead of the laboratory project. Values (1–5) are assigned for the respective instrument for each parameter as displayed in Table 4.
Table 4.
Deflection monitoring instrument | Strain monitoring instrument | ||||
---|---|---|---|---|---|
Parameter | Automatic total station | Linear variable differential transducer | Light detection and ranging | Electric strain gauge | Fiber optic sensor |
Accuracy and reliability | 5 | 5 | 4 | 4 | 5 |
Autonomy | 5 | 5 | 1 | 5 | 2 |
Real time, long term, and continuous | 5 | 4 | 1 | 3 | 2 |
Installation process | 5 | 2 | 5 | 2 | 4 |
Site-specific limitations | 4 | 2 | 4 | 4 | 4 |
Operational ability on-site | 5 | 3 | 5 | 3 | 4 |
Alarm system compatibility | 5 | 4 | 1 | 4 | 2 |
Ease of use and effort | 5 | 5 | 2 | 5 | 4 |
Cost (function of capability) | 3 | 5 | 4 | 5 | 5 |
Training requirements | 4 | 5 | 2 | 5 | 3 |
The three primary forms of monitoring include control, knowledge, and emergency monitoring. For this study, control and knowledge monitoring are combined into the same criteria. To determine the optimal instrument for each monitoring form, the above matrix is weighted in terms of what parameters are more important for each respective monitoring case. The weighted percentages are based on how each parameter will affect the efficiency of the monitoring project. For example, autonomy or real-time capabilities are more important and thus weighted much higher for emergency monitoring compared with control. The assigned weights for each case are described in Table 5. The assigned values from Table 4 are then multiplied by the respective weighting and summed as shown in Table 6.
Table 5.
Parameter | Control/knowledge (%) | Emergency (%) |
---|---|---|
Accuracy and reliability | 35 | 15 |
Autonomy | 5 | 15 |
Real time, long term, and continuous capabilities | 10 | 15 |
Installation process | 5 | 5 |
Site-specific limitations | 10 | 5 |
Operational ability on-site | 15 | 15 |
Alarm system compatibility | 5 | 15 |
Ease of use and effort | 5 | 5 |
Cost as a function of capability | 5 | 5 |
Training requirements | 5 | 5 |
Table 6.
Deflection monitoring | Strain monitoring | ||||
---|---|---|---|---|---|
Monitoring case | Automatic total station | Linear variable differential transducer | Light detection and ranging | Electric strain gauge | Fiber optic sensor |
Control/knowledge | 4.8 | 4.1 | 3.4 | 3.9 | 4.0 |
Emergency | 4.8 | 4.1 | 2.7 | 3.9 | 3.3 |
Note:
Bolded numbers identify the highest rated deflection monitoring or strain monitoring instrument for each monitoring case.
Based on the weighted matrix, in terms of deflection monitoring instruments, the ATS is the optimal instrument for emergency monitoring. Specifically, its autonomy, real-time capabilities, simple installation process, and built-in alarm system capabilities significantly outweigh the other deflection instruments. Moreover, the ATS is still operational during short-term power outages due to its long-term battery capabilities, which is advantageous during emergency events such as snow or ice storms. Conversely, the inherent nonautonomous nature of the LiDAR and extensive post-processing makes it unsuitable for emergency monitoring. However, the LiDAR is suitable for control/knowledge monitoring (and historical record keeping with respect to condition) mainly because it can determine deflections along the entire truss, is only restricted by line of sight, and is easy to set up. The ATS is also deemed suitable for control/knowledge monitoring due its simple installation process, accuracy and reliability, and because site limitations can be avoided. Although the ATS is restricted to local points, multiple targets are easily installed to increase the amount of data points available and provide control points. The LVDT is suitable for control/knowledge monitoring in-situ; however, the requirement for a stable reference system is still a major limitation for long-span trusses. Finally, both the ATS and LiDAR can provide transverse, longitudinal, and vertical deflection data. This can provide much insight regarding the 3-D behaviour of the trusses. Conversely, the LVDT is solely restricted to vertical deflection data at local points. In this regard, the LVDT is more suitable as a redundant instrument to validate ATS or LiDAR results.
In terms of strain monitoring, it was determined that the conventional gauges were more suitable for emergency monitoring compared with the FOS. Mainly, it reliably and accurately preformed in real time, continuously, and in the long term. In addition, the associated software was compatible with alarm systems and was inherently autonomous. Due to the extremely large data files obtained during continuous monitoring, the FOS was not deemed effective for emergency monitoring. However, the FOS proved to provide reliable distributed strain data along the entire truss during testing as opposed to the local strain data provided by the strain gauges. As such, it was determined that the FOS is reliable for control/knowledge monitoring as it can provide reliable information that is not necessarily feasible by the conventional gauges. In particular, the FOS provided valuable data in terms of bending stress as it provided an indication into the behaviour of the global truss and truss connections. In addition, the installation process was significantly easier compared with the strain gauges relative to the information being obtained.
6. Conclusion
The results and lessons learned from the laboratory component/experiments described within this paper aided in the development of an optimized monitoring procedure to be implemented in an operational Warren truss structure. During this laboratory phase various forms of monitoring techniques, in terms of their ability to capture the behaviour of the laboratory specimen, were compared and evaluated. It was determined (based on the criteria employed for a Warren Truss structure of this nature) that:
•
The ATS is the optimal emergency deflection monitoring instrument.
•
The ATS and LiDAR are suitable for control and knowledge monitoring.
•
Conventional strain gauges are the optimal emergency strain monitoring instrument.
•
Distributed FOS are best suited for control and knowledge strain monitoring.
Furthermore, lessons learned throughout the laboratory stage included:
•
the requirement for redundant and control instruments,
•
understanding the requirement to determine respective instrument limitations prior to in-situ implementation,
•
detailed instrumentation capabilities and limitations (Table 4) that the reader may refer to when analyzing and comparing SHM instruments for similar monitoring projects, and
•
critical monitoring locations and members of interest based on load/bending reversal, potentially overloaded members, and higher deflection locations.
Lastly, a metric-based comparison determined the suitability of the respective instruments for the three forms of monitoring. Overall, the laboratory testing of the scale model truss aided in determining an optimized SHM program, and the subsequent implementation, within the heritage WWII-era Warren truss structure.
Acknowledgements
The author acknowledges the support and funding provided by the RMC Green Team and the Department of National Defence. The author would also like to acknowledge the personal contributions of Mr. Dexter Gaskin, Mr. John Shaw, Mr. Adam Watson, and Dr. Ioannis Vazaois.
References
Bado MF, and Casas JR. 2021. A review of recent distributed optical fiber sensors applications for civil engineering structural health monitoring. Sensors, 21(5): 1818.
Barker C, Hoult NA, and Tolikonda V. 2019. Evaluation of a railway bridge using distributed and discrete strain sensors. In Proceedings of the 2nd International Conference on Smart Infrastructure and Construction 2019 (ICSIS), Cambridge, UK. 1–3 July 2019.
Bastianini F, Corradi M, Borri A, and di Tommaso A. 2005. Retrofit and monitoring of an historical building using “Smart” CFRP with embedded fibre optic Brillouin sensor. Construction and Building Materials, 19: 525–535.
Bertolini C, Spano A, Invernizzi S, Donadio E, Marzi T, and Sammartano G. 2015. The role of terrestrial LiDAR for mechanical and safety evaluation of historic timber trusses. In Proceedings of the 2nd International Conference on Historic Earthquake-Resistant Timber Frames in the Mediterranean Region, Lisbon, Portugal. 2–4 December 2015.
Bhalla S, Gupta A, Shanker R, Sethi A, Jain S, and Medury A. 2009. Performance and condition monitoring of structures using discrete strain measurements. International Journal of COMADEM, 12(1): 2–14.
Björngrim N, Hagman O, and Wang X. 2016. Moisture content monitoring of a timber footbridge. BioResources, 11(2): 3904–3913.
Branco JM, Sousa HS, and Tsakanika E. 2017. Non-destructive assessment, full-scale load-carrying tests and local interventions on two historic timber collar roof trusses. Engineering Structures, 140: 209–224.
Cavalli A, and Togni M. 2014. Monitoring of historical timber structures: state of the art and prospective. J Civil Struct Health Monit, 5(2): 107–113.
Chang PC, Flatau A, and Liu CS. 2003. Review paper: health monitoring of civil infrastructure. Structural Health Monitoring, 2(3): 257–267.
CSA. 2015. O86-14 Engineering design in wood. Canadian Standards Association Group, Mississauga, Ontario, Canada. 259 p.
Dietsch P, Gamper A, Merk M, and Winter S. 2014. Monitoring building climate and timber moisture gradients in large-span timber structures. Journal of Structural Health Monitoring, 5(2): 153–165.
Foo HC, and Seckin M. 1988. Safety evaluation of a timber Warren truss hangar. Structural Safety, 5(4): 299–315.
Forbes B, Vlachopoulos N, and Hyett AJ. 2018. The application of distributed optical strain sensing to measure the strain distribution of ground support members. FACETS, 3(1): 195–226.
Freas A. 1982. Evaluation, maintenance, and upgrading of wood structures. American Society of Civil Engineers, New York, New York. 444 p.
Green DW, Winandy JE, and Kretschmann DE. 2002. Mechanical properties of wood - technical report No. FPL-GTR-113. Forest Products Laboratory, Madison, Wisconsin. 46 p.
Hannan MA, Hassan K, and Ker PJ. 2018. A review on sensors and systems in structural health monitoring: current issues and challenges. Smart Structures and Systems, 22(5): 509–525.
Helmer-Smith H, Vlachopoulos N, Dagenais, M-A, and Forbes, B. 2021. In-situ load testing of a WWII era timber Warren truss in the development of a structural health monitoring program. Engineering Structures, 239 (2021): 112274.
Kwon I-B, Choi DH, Choi MY, and Moon H. 1998. Real-time health monitoring of a scaled-down steel truss bridge by passive-quadrature 3 × 3 fiber optic Michelson sensors. In Proceedings of SPIE 3325, Smart Structures and Materials 1998: Smart Systems for Bridges, Structures, and Highways, San Diego, CA, US. 17 June 1998.
Lanata F. 2015. Monitoring the long-term behaviour of timber structures. Journal of Civil Structural Health Monitoring, 5(2): 167–182.
Locklin L, Orellana J, and Akhras G. 2017. Monitoring wooden warren truss hangar to extend their design life. Journal of Civil Structural Health Monitoring, 7(2): 263–274.
Merkle W, and Myers J. 2004. Use of the total station for serviceability monitoring of bridges with limited access in missouri, USA. Center of Infrastructure Engineering Studies, Rolla, Missouri. 8 p.
Moreu F, Li J, Jo H, and Kim R. 2015. Reference-free displacements for condition assessment of timber railroad bridges. Journal of Bridge Engineering, 21(2): 04015052.
Moreu F, Kim RE, and Spencer BF. 2017. Railroad bridge monitoring using wireless smart sensors. Struct Control Health Monit, 24: e1863.
Palma P, and Steiger R. 2020. Structural health monitoring of timber structures – Review of available methods and case studies. Construction and Building Materials, 248: 118528.
Park HS, Lee HY, Choi SW, and Kim Y. 2013. A practical monitoring system for the structural safety of mega-trusses using wireless vibrating wire strain gauges. Sensors, 13: 17346–17361.
Psimoulis PA, and Stiros SC. 2013. Measuring deflections of a short-span railway bridge using a robotic total station. Journal of Bridge Engineering, 18(2): 182–185.
Tennyson R, Mufti A, Rizkalla S, Tadros G, and Benmokrane B. 2001. Structural health monitoring of innovative bridges in Canada with fibre optic sensors. Smart Materials and Structures, 10(3): 560–573.
Van Der Kooi K, and Hoult NA. 2018. Assessment of a steel model truss using distributed fibre optic strain sensing. Engineering Structures, 171: 557–568.
Van Der Kooi K, Hoult NA, and Le H. 2018. Monitoring an in-service railway bridge with a distributed fiber optic strain sensing system. Journal of Bridge Engineering, 23(10): 05018007.
Whelan MP, Albrecht D, and Capsoni A. 2002. Remote structural monitoring of the cathedral of como using an optical fiber bragg sensor system. International Society for Optical Engineering, 4694: 242–252.
Information & Authors
Information
Published In
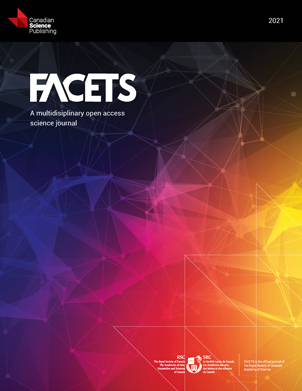
FACETS
Volume 6 • Number 1 • January 2021
Pages: 1510 - 1533
Editor: Arun Valsangkar
History
Received: 2 January 2021
Accepted: 21 April 2021
Version of record online: 2 September 2021
Copyright
© 2021 Helmer-Smith et al, RMC Green Team, Department of National Defence. This work is licensed under a Creative Commons Attribution 4.0 International License (CC BY 4.0), which permits unrestricted use, distribution, and reproduction in any medium, provided the original author(s) and source are credited.
Data Availability Statement
All relevant data are within the paper.
Key Words
Sections
Subjects
Plain Language Summary
Comparing and evaluating monitoring instruments by testing a laboratory scale Warren truss
Authors
Author Contributions
All conceived and designed the study.
HH-S and BF performed the experiments/collected the data.
All analyzed and interpreted the data.
NV and M-AD contributed resources.
All drafted or revised the manuscript.
Competing Interests
Dr. Nicholas Vlachopoulos currently serves on the editorial board of FACETS.
Metrics & Citations
Metrics
Other Metrics
Citations
Cite As
Henry Helmer-Smith, Nicholas Vlachopoulos, Marc-André Dagenais, and Bradley Forbes. 2021. Comparison of multiple monitoring techniques for the testing of a scale model timber Warren truss. FACETS.
6: 1510-1533.
https://doi.org/10.1139/facets-2021-0001
Export Citations
If you have the appropriate software installed, you can download article citation data to the citation manager of your choice. Simply select your manager software from the list below and click Download.
Cited by
1. Long-term deflection monitoring of a heritage, timber truss structure, and development of an integrated emergency alarm program
2. Buildings’ Biaxial Tilt Assessment Using Inertial Wireless Sensors and a Parallel Training Model
3. Assessing the structural behaviour of glued-laminated timber beams using distributed strain sensing