A critique of Hubbert’s model for peak oil
Abstract
In 1956, Shell Oil Company geologist M. King Hubbert published a model for the growth and decline over time of the production rates of oil extracted from the land mass of the continental US. Employing an estimate for the amount of ultimately recoverable oil and a logistic curve for the oil production rate, he accurately predicted a peak in US oil production for 1970. His arguments and the success of his prediction have been much celebrated, and the original paper has 1400 publication citations to date. The theory of “peak oil” (and subsequently, of natural resource scarcity in general) has consequently become associated with Hubbert and “Hubbert” curves and models. However, his prediction for the timing of a world peak oil production rate and the subsequent predictions of many others have proven inaccurate. We revisit the Hubbert model for oil extraction and provide an analysis of it and several variants in the language of (time) autonomous differential equations.
Introduction
In his seminal paper, prepared for a meeting of the American Petroleum Institute in Texas in 1956, Shell Oil geologist Marion King Hubbert attempted to answer the question, “how far along have we come on our way to complete exploration [of world oil fields]?” (Hubbert 1956, p. 2). The paper presented a resource model constructed to answer this question. Hubbert writes that,
as an essential part of our analysis, we can assume with complete assurance that the industrial exploitation of the fossil fuels will consist in the progressive exhaustion of an initially fixed supply to which there will be no significant additions. (Hubbert 1956, p. 4).
Consequently, the two fundamental questions that drive the analysis, both for Hubbert and again here in this critique, are as follows:
1.
What is the recoverable amount of the “initially fixed supply”?
2.
Can we predict the timing of the “progressive exhaustion” of this ultimate resource amount?
As per Morehouse (1997), we will use the term “ultimate resource” (UR) for the amount in question 1, and note that several synonyms are often used: “estimated ultimate recovery” (EUR), “ultimate recoverable resources”, and “ultimate recovery” (Morehouse 1997; Maggio and Cacciola 2012).
In 2005, to mark its 125th anniversary, Science published a special issue introduction titled “What Don’t We Know?” (Kennedy and Norman 2005). Among this list of the most profoundly vexing inquiries currently confounding the human race is an updated variant of the Hubbert questions: “What can replace cheap oil—and when?” (Kerr and Service 2005, p. 101). In this form of the question there appears to be an implicit assumption that progressive exhaustion of world oil reserves results in “expensive” (or even, “no more”) oil. Thus, this question leads back to the twin questions of ultimate reserve amount and prediction of future production levels, now nuanced by additional questions concerning: the cost and difficulty of extraction, the availability of cost-competitive energy alternatives, and society’s willingness and ability to curb its appetite for cheap oil. These latter questions, though increasingly relevant, are not addressed. Rather, we attempt to explore, update, and critique the answers to the two fundamental questions provided by the original Hubbert model from 60 years ago.
Reserve to production ratio
A first estimate of the UR of oil is to use the petroleum engineering profession’s estimate of “world oil reserve”, R(t), which is the amount of technically and economically recoverable oil from all known world oil fields at a particular time, t ≥ 0, measured in years. Such estimates are fraught with uncertainty, depend heavily on reliable geologic and engineering data (not always available), require expert and experienced interpretation of those data, and are hopefully untainted by geo-political interference. “Proven” reserves, based on the definition approved by the Society of Petroleum Engineers, are those claimed to have a reasonable certainty (statistically, at least 90% confidence) of being recoverable. Such proven reserves are known in the industry as “1P” (Society of Petroleum Engineers 2005).
In this paper we will use the consistent time series oil data as published annually by British Petroleum (BP) (BP 2017a, 2017b). These BP data, both for “reserve” and for “production”, purport to be inclusive regarding the type of oil referenced. According to BP,
Oil reserves include field condensate and natural gas liquids as well as crude oil. This inclusive approach helps to develop consistency with the oil production numbers published in the Review, which also include these categories of oil. Liquid hydrocarbon fuels from non-hydrocarbon sources, such as ethanol from corn or sugar or synthetic oil derived from natural gas (so-called GTL or gas-to-liquids), are not included in either the reserves or production series. (BP 2017c)
We will use BP data for the world oil production rate at time t, here denoted P(t) gigabarrels (Gbbl)/year. From the same source, R(t) consists of 37 data points, beginning in 1980 (t = 0) to the most recent values (t = 36) from 2016. Data for P(t) go back to 1965, yielding 52 data points. The cumulative amount of oil extracted, Q(t) Gbbl, since the discovery of oil in Pennsylvania in 1859, is then constructed by adding the yearly production amounts to an estimate from Gallagher (2011) for Q(0), herein labelled Qo = Q(0), setting t = 0 to a convenient year.
When we use R(t) as a first estimate of UR, the simplest estimate of the “time to depletion” (i.e., an answer to the second posed question) is to compute the ratio, R(t)/P(t) years, which measures the number of years remaining given the current reserve level and present rate of extraction (production) until the reserve is completely exhausted. Measurements of this R/P ratio have been employed for decades, popping up in diverse places. For instance, in the environmental science text (Harte 1988), the computation based on 1980 data1, reportedly extracted from Hubbert (1969), is as follows:
The Institution of Mechanical Engineers in the UK posted a 2014 article to their website, which has since been removed, titled “When will oil run out?”. Employing the R/P ratio, they stated:
There are an estimated 1.3 trillion barrels of proven oil reserve left in the world’s major fields, which at present rates of consumption will be sufficient to last 40 years. By 2040, production levels may be down to 15 million barrels per day—around 20% of what we currently consume. (Institution of Mechanical Engineers 2014)
Other journalists use the same information in a less alarmist fashion. For instance Maclean’s from February 2014:
According to BP’s Statistical Review of World Energy, global oil reserves at the end of 2012 were 1.7 trillion barrels. Given that the world consumes about 86 million barrels of crude oil per day, it would be easy to conclude we’ll run out of oil in 55 years, or sooner if we increase consumption. (Leach 2014)
The author then goes on to explain why, despite generally increasing consumption rates, the oil reserves amount has increased over the past 30 years rather than declined, concluding with a definitive prediction, “So, what’s the bottom line? Are we going to run out of oil? No”. (Leach 2014)
So what do the data show? Figures 1a and 1b show the most recent world oil reserve and production data from BP, with their regression lines in each case. Both reserve and production exhibit strongly increasing trends. The regression line for reserve has a very strong correlation coefficient of 0.9895, and reserves have been increasing at a rate of almost 30 Gbbl/year since 1980 (slope of 29.17). The correlation coefficient for production is also strong at 0.9564, and world oil production has approximately tripled since 1964.
Fig. 1.

Figure 2 shows the time history of the R/P ratio for the world with the regression line for the computed data.
Fig. 2.

Clearly the data show an upward trend in the R/P ratio as well; the regression line has a slope of 0.5004. Thus, the number of years of oil remaining has been increasing by half a year per year since 1980. Further, the growth in the R/P ratio has occurred despite steadily increasing production.2 The correlation coefficient of 0.8932, corresponding to a coefficient of determination of r2 = 0.7979 for the R/P ratio data, indicates that the growth trend is strong. As a consequence we conclude that the R/P ratio does not provide a reasonable predictor for the end of the age of oil.
1
A factor 6.1 × 1018 J/Gbbl converts the crude oil energy amounts in joules (J) to gigabarrels of oil (Gbbl).
2
A >50% increase since 1980.
Hubbert model: The logistic curve
M. King Hubbert was interested in the answers to the two fundamental questions in the context of oil, coal, and natural gas, and their application to various regions, such as individual oil fields, Texas, the US, and finally, the world. In this paper, we focus on world oil. To address his questions, Hubbert proposed a more sophisticated approach than the simplistic R/P ratio. He realized that production rate curves, as functions of time, for oil fields, whole geographic regions, and other fossil fuels share the feature in which the “curve starts slowly and then rises more steeply until finally an inflection point is reached after which it becomes concave downward” (Hubbert 1956, p. 6). He deduced that “the significance of this is that during the initial stages [of resource exploitation] all of these rates of production tend to increase exponentially with time” (Hubbert 1956, p. 7). However, “no finite resource can sustain for longer than a brief period such a rate of growth of production; therefore, although production rates tend initially to increase exponentially, physical limits prevent their continuing to do so” (Hubbert 1956, p. 8). He proposed two requirements for his models (Hubbert 1956, p. 9):
1.
The production rate is zero at the outset and “after passing through one or several maxima, it must decline again to zero”.
2.
The area under the production rate curve (above the time axis), between the outset time and the end time, t → ∞, is the UR amount.
Consequently, to satisfy requirements 1 and 2, Hubbert settled on a resource production rate model that is a family of curves bearing the characteristic shape of a bell (Hubbert 1956, fig. 11, p. 10). These logistic curves depend on three parameters, Q∞, r, and b. They are described algebraically by the formula
(1)
From eq. (1) Q(t) can be recovered as:where Qo = Q(0) and . Equation (2) follows from eq. (1) because the production/extraction rate, P(t), is the time derivative of the cumulative extracted amount, Q(t).
(2)
Picking the parameters to match physical data and (or) estimates is an exercise in curve fitting. The first parameter, Q∞, represents the UR. Hubbert used his expertise as an oil geologist to carefully justify a global estimate for Q∞, the “initial amount present” for the whole world, namely Q∞ =1250 Gbbl (Hubbert 1956, p. 22). This value becomes the area under the world oil production curve, in agreement with eq. (2). Next, the value b can be computed from the initial amount via b = ln(Q∞/Qo−1) (c.f. eq. (4), evaluated at t = 0). Finally, r is calculated from Hubbert’s estimate of the peak (maximum) production rate, “two and one-half times the present [1956] rate” (Hubbert 1956, p. 22). Thus, Pmax = 12.5 Gbbl/year, and r comes from the relation Pmax = rQ∞/4. The result of this model by Hubbert (1956, fig. 20) “… places the [predicted] date of the peak at about the year 2000” (Hubbert 1956, p. 22).
In the mathematical modelling of physical phenomena, simple curve fitting has inherent weaknesses. A wide variety of curve families with simple algebraic structure can be chosen to fit a given set of data over time, but the usefulness of such models as predictors of the future is suspect, unless the choice is based on more fundamental dynamics or first principles. In this case, the family of curves Hubbert employed arises from the solution of a differential equation called the logistic equation. In this context, the logistic equation relates the production rate P(t) and the cumulative amount Q(t) dynamically in time, allowing for a more fundamental justification of the model. In the authors’ opinion, it is this more fundamental justification that gives the Hubbert peak oil model its credibility and enduring impact. We therefore attempt the following detailed presentation.
Noting that P is the time rate of change of Q (i.e., P(t) = Q′(t)), the logistic differential equation for Q(t) is:
(3)
This model is to be satisfied for all t > 0 and for the given (small, positive) initial value, Qo = Q(0). The solution of the differential equation is the unknown Q(t), which satisfies the initial value at t = 0 for given (positive, constant) parameters, r and Q∞. As the differential equation depends only on Q and the model parameters, and not explicitly on t, it is termed time-autonomous. The model from eq. (3) requires that P = Q′ be jointly proportional to a “growth term”, Q, and to a “limiting term”, (1 − Q(t)/Q∞). We examine each of these model features in order.
Suppose the limiting term had little effect and could be ignored. This situation would occur for the time period near the outset at t = 0, when Q ≈ 0, which gives the limiting term a value approximately equal to one. In this initial period the model essentially behaves like the easier relation, Q′(t)/Q(t) = r (take the limiting term to be 1 in eq. (3) and divide through by the remaining operative term, Q). This latter equation says that the relative growth rate, Q′/Q, is a constant, r, allowing us a first principles understanding of the r parameter as the relative growth rate. The solution would now be exponential, Q(t) = Qoert and P(t) = Q′(t) = rQoert. As desired, for a brief time near the outset, the production rate starts close to zero and increases exponentially at relative growth rate r. However such a model cannot be valid for an extended time period, as P(t) has no maxima and never again declines (much less to zero). Rather, P(t) → ∞ as t → ∞, violating the physical limits for a finite resource. To get the production rate to reach a maximum, and decline again toward zero (producing the characteristic bell shape), the model must limit the growth term. Thus, the “limiting term” begins to take effect as Q increases, thereby reducing the effective relative growth rate.
So when Q(t) is not small, the limiting term, (1 − Q(t)/Q∞) is not one, has effect, and cannot be ignored. Again dividing by Q, eq. (3) can be written Q′(t)/Q(t) = r(1 − Q(t)/Q∞). Now it is apparent that the relative growth rate is not the constant r, but rather a linearly decreasing function of Q, whose value near the outset is close to r, and decreases to zero as Q(t) grows to Q∞. Thus, as Q(t) (and therefore its derivative, P(t)) initially grows exponentially, the limiting term (1 − Q(t)/Q∞) decreases. This decrease in the limiting term causes the production rate Q′ = P to peak and then to decline. Because the production rate declines to zero (as desired) as the cumulative amount grows toward Q∞, it does so increasingly slowly and never exceeds Q∞. Thus, Q∞ represents the UR, the amount Hubbert carefully estimated to answer question 1, before using it in his logistic model to answer question 2. With this interpretation of Q∞ as UR, the limiting term represents the proportion of world oil remaining in the ground at t. The authors view the magnitude of Q(t) as a proxy measure of the current size of the aggregate global oil economy, including the amounts invested in equipment and oil infrastructure, the number of uses of oil, and the sheer number of end users. In this view, the rate of change, P(t) = Q′(t), would indeed be proportional to Q(t), as per the logistic model in eq. (3).
Thus, the logistic differential equation, eq. (3), has the following fundamental dynamic interpretation in the context of resource extraction: it models a production rate that changes (grows or declines) simultaneously proportional to
1.
the current cumulative resource extracted—a proxy measure of the current “size” of the world oil economy, driving production growth; and
2.
the current remaining amount of unextracted resource—the increasing production difficulty as remaining resource shrinks growth and limits the influence of the cumulative amount.
The parameter r is the relative growth rate of cumulative extracted resource, in the absence of any resource limit. The parameter, Q∞, represents the physical resource limit, the UR.
The solution of the logistic equation, eq. (3), is achieved by the standard technique of separating variables and integrating, which yields:from which P(t) = Q′(t) is given by eq. (1) (see Figs. 3a and 3b). The characteristic shape of Q(t) is sigmoidal, rising asymptotically from 0 as t increases from −∞, passing through an inflection point, and asymptotically growing to Q∞ as t → ∞. It is symmetric about its inflection point, t⋆ = b/r, where P′(t⋆) = Q″(t⋆) = 0. Therefore, at the inflection Q(t⋆) = Q∞/2. Consequently P(t) is symmetric about its peak, P(t⋆) = rQ∞/4 = Pmax. Thus, according to Hubbert’s model, exactly half the resource remains in the ground at the time when the production level peaks. Subsequent declines in the production level precisely mirror the swiftness of the earlier production level rise.
(4)
Fig. 3.

Hubbert model versus current data
So what do the data show? We make three comparisons: Hubbert’s 1956 model versus subsequent data for the US, Hubbert’s 1956 model versus subsequent world data, and finally world data to 2016 used to “best fit” an updated Hubbert logistic curve.
Hubbert model versus US data
When Hubbert applied his model to oil production for the continental US (with two different estimates for the ultimate resource), the larger of the two UR estimates correctly predicted the peak production to occur in 1970 as shown by Hubbert (1956, fig. 21). As described previously, the model also predicts a subsequent steep production rate decline, mirroring the prepeak exponential rise. The BP data (BP 2017b) shown in Fig. 4 certainly show the celebrated peak in 1970, cementing the impact of Hubbert’s model. However, the subsequent decline is not symmetric with the earlier rise and so fails to follow the model logistic curve as predicted. Increases in the late 1970s, because of new large discoveries in Alaska, and the current remarkable rise since 2008 make the present production rate higher than at the 1970 peak. The current increases are due to the advent of a new technology: fracking, a process that has made so-called “tight oil”3 economical to extract. Of course, other technologies created since 1956 have also had impact on production, such as deep water drilling platforms and horizontal drilling. From the clear evidence of the data, Hubbert’s logistic model as applied to the US appears unable to answer either of the two fundamental questions. Despite the success of the initial prediction of peak oil for the US, the Hubbert model does not seem to adequately capture the fundamental dynamics.
Fig. 4.

Hubbert model versus world data
The situation for Hubbert’s 1956 model predictions for world oil (peak production rate, time of peak, and UR) are all inaccurate. The world cumulative oil (extracted) is shown in Fig. 5 and results from adding the BP production data in Fig. 1b to Gallagher’s estimate for the year 1966 Q = 185.226 Gbbl (see Gallagher (2011), table 1, p. 793). Whereas Hubbert’s careful estimate of world UR was Q∞ = 1250 Gbbl, world cumulative oil exceeded that amount in 2011 and stands at 1423 Gbbl in 2016, (see Fig. 5) with an additional 1706.7 Gbbl of proven reserve. (Fig. 1a.) Additionally, the predicted peak production rate of 12.5 Gbbl/year, which was to occur in the year 2000, was exceeded a mere 10 years later in 1966. As of 2016, the world production rate is up to 35.24 Gbbl/year, is still rising (the regression line slope is about 1% or 0.33 Gbbl/(year·year) since 1965), and has no apparent peak in sight (see Fig. 1b). Again the Hubbert model has turned out to be inaccurate.
Fig. 5.

Using Hubbert logistic model to predict UR
Perhaps the Hubbert model can be updated from the current data? Using the BP world production and cumulative oil data to 2016, fitted to the Hubbert model, the computed “best” value for the parameter Q∞ would be the predicted UR. This fitting can be accomplished in two ways. In the first, noting that P = Q′ by definition and letting Y = P/Q be the relative rate of change of Q, rewrite the logistic equation, eq. (3), as
(5)
This is called the “Hubbert linearization” (Hubbert 1982). Now the variables (Q, Y) must be linearly related. Thus, data point pairs, (Qi, Yi) = (Q(ti), P(ti)/Q(ti)), i = 1 … 52, can be used to find the standard regression line. From this method, the BP data produce best fit values: r = 0.0703 per year, Q∞ = 1739.85 Gbbl, with the correlation coefficient −0.9097. The disadvantage of this method is that although the differential equation has been “fit”, it has not been solved; there is still a free initial condition (arbitrary constant of integration). To plot the solution curve given by eq. (4), a particular Q value (usually the first or the last data point) has to be arbitrarily preferred by being forced onto the model curve. Rather than arbitrarily preferring a single data point, we will use an alternative approach to fit the data to the logistic model.
The second technique allows the solution curve for the logistic equation, eq. (4), to be fit with the constant of integration left as one of the fitted parameters. Again we use linear regression, despite the curve’s nonlinear form, and its three independent parameters. Rewriting eq. (4) as:we again define a new variable, Y(t; Q∞) = ln(Q∞/Q − 1), which is linearly related to t for each (fixed) value of the free parameter Q∞. Therefore, the data (ti, Yi), where Yi = Y(ti; Q∞), produce a standard linear regression formula for intercept and slope parameters, b = b(Q∞) and r = r(Q∞), for each fixed value of Q∞. Now construct the squared-deviation error function:and minimize this function numerically or graphically (Maple® 15 produces a strong visual minimum in the plot). This second technique has the advantage of fitting three free parameters and does not require discrete slope data. From this method, the BP data produce best fit values: r = 0.0617 per year, b = 2.2305, and Q∞ = 1922.5 Gbbl, with root mean square error 19.6 Gbbl. Thus, the current data, applied to Hubbert’s model, predict UR to be just <2000 Gbbl.
(6)
(7)
The Hubbert cumulative model curve with UR set to 1922.5 is plotted against the BP data in Fig. 6. The fit looks very good. However, the current world reserve of 1706.7 Gbbl added to the current cumulative of 1423 Gbbl means that true UR is certainly larger than the sum, 3.1 trillion barrels. Therefore the predicted amount of 1922.5 Gbbl is far too small. Put another way, if Fig. 6 is correct, the world has only a half-trillion barrels of oil currently remaining, and peak oil occurred when Q(t) = 961.3 Gbbl back in 2001. Clearly the Hubbert model remains inaccurate, even when applied using up-to-date data.
Fig. 6.

3
Oil trapped in layers of shale and sedimentary rock, rather than pooled in reservoirs.
A system of ordinary differential equations: A Hubbert variant
Given that we have demonstrated the inaccuracy of the Hubbert logistic model, eq. (3), to adequately capture the dynamics driving the data sets for reserve, production, and cumulative extraction of world oil, answers to our questions 1 and 2 seem elusive. The particular problem seems to be the ongoing growth of reserve, making earlier estimates of UR either wrong or, at best, poorly justified. As Morehouse (1997, p. vii) stated, “reserves growth” is an “intricate puzzle”, and he names the phenomenon “ultimate recovery appreciation”, “the generally observed increase of EUR over time”. Reserve growth is driven by two related factors: the discovery of new oil fields and technological innovation (both new and improved technologies).
As an example, we point to the case of the Hibernia oil project off the coast of Newfoundland, Canada. When the original development project was submitted for government approval before production began in 1997, the application included a reserve estimate of “recoverable oil of 563 million barrels” and a lifespan of about 20 years. However, CBC News reported in December 2016 (Roberts 2016), that the billionth barrel was pumped on the 21st of that month, and that the current expectations are for production to continue until about 2040, recovering 1.6 billion barrels cumulative. The reserve growth is due in part to new abilities to extend drilling horizontally.
The report is striking; after exceeding the oil-field production target by 77% and approaching the end of the projected lifespan of the field, the reserve remaining actually exceeds the original projections of the resource. One wonders whether this same report will be repeated in a further 20 years, with an announcement that the remaining oil still exceeds a half-billion barrels. Yet such a possibility cannot be discounted, even while reason suggests the process cannot continue indefinitely.
A successful oil production model must account for the phenomenon of reserve growth. A wide variety of Hubbert model variants have been proposed in the literature. These include multi-Hubbert, modified Hubbert, and higher-order Hubbert models, such as those proposed by Campbell and Laherrère (1998), Berg and Korte (2008), Sorrell and Speirs (2010), Maggio and Cacciola (2012). Various other curve-fitting processes have also been employed (see Lynch (2003) and Brandt (2010)). Here we propose a simple, new, “Hubbert variant” model that allows for dynamic reserve growth with a reasonable justification. The model is a system of two time-autonomous differential equations, one for cumulative oil, Q(t), and a second for reserve, R(t), including three positive-value parameters:
(8)
(9)
The first, eq. (8), reproduces the Hubbert logistic model with one modification. Here, the growth-limiting term, the remaining unextracted resource, Q∞ − Q(t), has been replaced by the current reserve, R. Because reserve is only the best 1P estimate of the remaining resource, as R(t) ≤ Q∞− Q(t), the impact of this new growth-limiting term is felt earlier in the time history.4 The second, eq. (9), says that the rate of change of the reserve has two independent (additive) factors. The first of these is obvious: reserve declines at an annual rate equal to the amount extracted each year by the current production rate, P. The second term also allows reserve to grow logistically, with relative growth rate α and growth-limiting constant β. In this view, reserve, like a water-loaded sponge, can always have some additional percentage α of the current loading R added to R, because we can squeeze the sponge. Here the ability to “squeeze” the sponge mimics the effect of technology-driven resource expansion. However, this process cannot continue indefinitely and growth is therefore limited by the factor (1 − βR).
Note that in the limiting case where reserve is not allowed to grow (i.e., take α = 0), the model reverts to the Hubbert model. Setting α = 0 in eq. (9) makes R′ = −P = −Q′ (with the latter equality by the definition of production rate). Integrating now implies that R(t) = −Q(t) + Q∞, where the constant of integration is chosen to match the total reserve when cumulative is zero, i.e., when reserve was UR. Using this expression to replace R in eq. (8) and recalling that ρ = r/Q∞, we recover the Hubbert model. Therefore, when we express the solution of the Hubbert model in (Q, R) coordinates (with Q on the horizontal axis), the curve is the straight line, R + Q = Q∞, with time increasing along the line to the right—the direction of increasing cumulative. For different assumed values of Q∞ the Hubbert lines are all parallel, with intercept values Q∞ on both axis. In Fig. 7 two possible Hubbert lines are plotted, the first using the current, 2016, value of R + Q = 3130 [Gbbl] as the Q∞.
Fig. 7.

The Hubbert variant model can be solved analytically: dividing eq. (9) by eq. (8) and using the chain rule yields a linear differential equation for R as a function of the independent variable, Q:which is solved in the standard way. The solution is:where c is the arbitrary constant of integration and a represents the collection of parameters a = αβ/ρ. The model, eq. (11), has three, positive parameters to be determined, a, c, and 1/β. We used the “NonlinearFit” routine of the Maple® 15 mathematics package to find optimal values (in the least squares sense) for these parameters to best fit this model equation to our 37-point data sets for R and Q. The result is plotted in Fig. 7, in the plane of cumulative versus reserve.
(10)
(11)
The “best fit” Hubbert variant model curve in Fig. 7 does fit the BP data reasonably well. From the graph, the horizontal intercept where R = 0 is interpreted as the UR. The model predicts a UR of 5484.2 Gbbl, answering question 1. Using this value as Q∞ produces the second Hubbert line on the plot. Given that all Hubbert model curves (for any chosen value of Q∞) are lines parallel to the two in Fig. 7, it should be clear that none bear any resemblance to the actual data. This observation again confirms that the Hubbert model for world oil is highly inaccurate and is therefore refuted. To some, the above predicted UR (5.5 terabarrels (Tbbl)) may seem unjustifiably high.
As for the variant model, we add the following observations. Time is an (unknown) parameter along the curve. Differentiating eq. (8) with respect to time (having first replaced R with R(Q(t)) from eq. (11)) and numerically finding the zero, allows us to say, definitively, that the best fitting model of this type predicts peak world oil production to occur when the cumulative amount of oil is Q = 2998 Gbbl, for which the corresponding reserve has declined to R = 1199.7 Gbbl. To determine the time at which this event occurs requires us to estimate the parameter ρ in the differential equation, eq. (8). Unfortunately, there is no analytic solution available to allow the parameter to be estimated from the data to minimize the error. However, we can make the following rough estimate of ρ; using the most recent (2016) data value for Q, P, and R, we estimate ρ = P(2016)/[Q(2016)R(2016)]. Now eq. (8) can be solved numerically, using the initial condition of Q(2016), thereby creating a time series for Q. Then peak world oil occurs when Q = 3000, for which t = 73.8 [years since 1979], i.e., 2052. The production level at that time is approximately 50 Gbbl/year, which is almost 50% higher than current production. This estimate for the timing of peak oil is an upper bound on values produced by various estimates of the parameter, ρ. Also, world cumulative exceeds Q = 4 Tbbl about 2075 and production effectively ceases by the time Q = 5.4 Tbbl at 2160, giving a rough answer to question 2. We reiterate that these are just rough estimates: the computed time value and production value for the “peak” are sensitive to the estimate of ρ in eq. (8).
4
Note, the relative growth rate parameter, r from the Hubbert model corresponds to a rescaled ρ = r/Q∞ here.
Conclusion
Hubbert need not feel alone in making unrealized predictions concerning world oil production. In a comprehensive review article Brandt (2010, p. 3958) classified 45 “mathematical models of oil depletion of the last century”, all of which attempt to forecast future oil production paths (most with global scope). Concerning the predictive capacity of all of these models, he concluded:
Existing models have fared poorly in predicting global oil production. Even for models that are commonly thought to be successful, after-the-fact interpretation of the success or failure of a predictive effort is not easy (recall the discussion above of Hubbert’s successful prediction). … This author’s judgement with respect to the predictive value of models is as follows: … There is no scientific justification for making specific predictions (e.g., the year of peak production) with any of the surveyed mathematical models: the uncertainties involved make such predictions of little use. (Brandt 2010, p. 3972)
Despite this advice, “Peak Oil” papers and predictions continue to be published and then proven false. For example, Mohr and Evans (2008) made a 2007 prediction for (UR) 2.2 trillion barrels with a world oil production peak in 2013, with the caveat that the peak will be somewhat later if the UR reaches 3 trillion barrels. However, the data (Figs. 1a, 1b, and 5) show that the 2016 value of R + Q = 3130 Gbbl is already >3 trillion barrels, with no production peak in sight, therefore completely refuting the predictions. As a final example, Gallagher (2011) used an “idealized Hubbert curve” to make a 2010 prediction that peak oil had just occurred in 2009 at 30.4 Gbbl/year with UR of 2.24 trillion barrels. Again, these values have already been surpassed, indicating that the prediction has failed.
Brandt ended the earlier quote of his conclusions concerning oil production predictions with the following exhortation (Brandt 2010, p. 3972): “Efforts should move away from making these kinds of predictions, and toward understanding the impacts of the inevitable transition to oil substitutes.”
And so we are back to the beginning: we have not provided credible answers to either of the two fundamental questions. We acknowledge that the answers are elusive because prediction (beyond predicting a regression line trend for the very near term) is extremely difficult. Clearly the underlying dynamics of reserve growth are poorly understood, and though many have made oil production peak and depletion time predictions, none can be trusted. Finally Brandt (2010) points us towards contemplation of the broader aspects of the investigation, along the lines of the question from Science (Kerr and Service 2005): “What can replace cheap oil—and when?”
Acknowledgements
The authors acknowledge helpful discussions with, and computational aid from, Bishop’s University summer undergraduate research assistants, Jacob Courtemanche (2015), and Courtney Drew (2017).
References
Berg P, and Korte S. 2008. Higher-order Hubbert models for world oil production. Petroleum Science and Technology, 26: 217–230.
BP. 2017a. BP statistical review of world energy. bp.com/en/global/corporate/energy-economics/statistical-review-of-world-energy.html.
BP. 2017b. BP statistical review of world energy 2017 workbook. bp.com/content/dam/bp/en/corporate/excel/energy-economics/statistical-review-2017/bp-statistical-review-of-world-energy-2017-underpinning-data.xlsx.
Brandt AR. 2010. Review of mathematical models of future oil supply: historical overview and synthesizing critique. Energy, 35: 3958–3974.
Campbell CJ, and Laherrère JH. 1998. The end of cheap oil. Scientific American, 278(3): 78–83.
Gallagher B. 2011. Peak oil analyzed with a logistic function and idealized Hubbert curve. Energy Policy, 39: 790–802.
Harte J. 1988. Consider a spherical cow: a course in environmental problem solving. University Science Books, Sausalito, California.
Hubbert MK. 1956. Nuclear energy and the fossil fuels. Technical Report Publication No. 95. Shell Development Company, Exploration and Production Research Division, Houston, Texas.
Hubbert MK. 1969. Energy resources. In Resources and man. Committee on Resources and Man, National Academy of Sciences, National Research Council. W. H. Freeman, San Francisco, California.
Hubbert MK. 1982. Techniques of prediction as applied to the production of oil and gas. In Oil and gas supply modeling, National Bureau of Standards special publication 631. Edited by SI Gass. National Bureau of Standards, Washington, D.C. pp. 16–141.
Institution of Mechanical Engineers. 2014. When will oil run out? [online]: Available from archive.is/gwqmW. Originally at: imeche.org/knowledge/themes/energy/energy-supply/fossil-energy/when-will-oil-run-out.html.
Kennedy D, and Norman C. 2005. What don’t we know? Science, 309(5731): 75.
Kerr RA, and Service RF. 2005. What can replace cheap oil—and when? Science, 309(5731): 101.
Leach A. 2014. What happens when we run out of oil? [online]: Available from macleans.ca/economy/what-happens-when-we-run-out-of-oil.
Lynch MC. 2003. The new pessimism about petroleum resources: debunking the Hubbert model (and Hubbert modelers). Minerals & Energy—Raw Materials Report, 18(1): 21–32.
Maggio C, and Cacciola G. 2012. When will oil, natural gas, and coal peak? Fuel, 98: 111–123.
Mohr SH, and Evans GM. 2008. Peak oil: testing Hubbert’s curve via theoretical modeling. Natural Resources Research, 17(1): 1–11.
Morehouse DF. 1997. The intricate puzzle of oil and gas “Reserves Growth. In Natural gas monthly. Energy Information Administration, Washington, D.C. pp. vii–xx.
Roberts T. 2016. Hibernia pumps 1 billionth barrel of oil in Newfoundland’s offshore. CBC News [online]: Available from cbc.ca/news/canada/newfoundland-labrador/hibernia-milestone-billionth-barrel-1.3908620.
Society of Petroleum Engineers. 2005. Glossary of terms used in petroleum reserves/resources definitions [online]: Available from spe.org/industry/docs/GlossaryPetroleumReserves-ResourcesDefinitions_2005.pdf.
Sorrell S, and Speirs J. 2010. Hubbert’s legacy: a review of curve-fitting methods to estimate ultimately recoverable resources. Natural Resources Research, 19(3): 209–230.
Information & Authors
Information
Published In
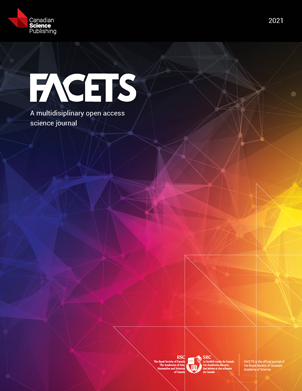
FACETS
Volume 3 • Number 1 • October 2018
Pages: 260 - 274
Editor: Patrick Ingram
History
Received: 30 June 2017
Accepted: 21 November 2017
Version of record online: 8 March 2018
Copyright
© 2018 Jones and Willms. This work is licensed under a Creative Commons Attribution 4.0 International License (CC BY 4.0), which permits unrestricted use, distribution, and reproduction in any medium, provided the original author(s) and source are credited.
Data Availability Statement
All relevant data are within the paper.
Key Words
Sections
Subjects
Authors
Author Contributions
NBW conceived and designed the study.
All contributed resources.
All drafted or revised the manuscript.
Competing Interests
The authors have declared that no competing interests exist.
Metrics & Citations
Metrics
Other Metrics
Citations
Cite As
Trevor H. Jones and N. Brad Willms. 2018. A critique of Hubbert’s model for peak oil. FACETS.
3(1): 260-274. https://doi.org/10.1139/facets-2017-0097
Export Citations
If you have the appropriate software installed, you can download article citation data to the citation manager of your choice. Simply select your manager software from the list below and click Download.
Cited by
1. Production Forecasting at Natural Gas Wells
2. Rethinking plastics
3. Peak oil and the low-carbon energy transition: A net-energy perspective
4. A Copula–Hubbert Model for Co(By)-Product Minerals